3.1.5
Reference Guide
This guide describes the Apache Kafka implementation of the Spring Cloud Stream Binder. It contains information about its design, usage, and configuration options, as well as information on how the Stream Cloud Stream concepts map onto Apache Kafka specific constructs. In addition, this guide explains the Kafka Streams binding capabilities of Spring Cloud Stream.
1. Apache Kafka Binder
1.1. Usage
To use Apache Kafka binder, you need to add spring-cloud-stream-binder-kafka
as a dependency to your Spring Cloud Stream application, as shown in the following example for Maven:
<dependency>
<groupId>org.springframework.cloud</groupId>
<artifactId>spring-cloud-stream-binder-kafka</artifactId>
</dependency>
Alternatively, you can also use the Spring Cloud Stream Kafka Starter, as shown in the following example for Maven:
<dependency>
<groupId>org.springframework.cloud</groupId>
<artifactId>spring-cloud-starter-stream-kafka</artifactId>
</dependency>
1.2. Overview
The following image shows a simplified diagram of how the Apache Kafka binder operates:
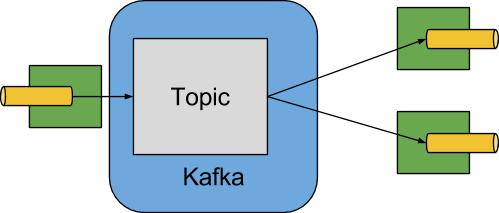
The Apache Kafka Binder implementation maps each destination to an Apache Kafka topic. The consumer group maps directly to the same Apache Kafka concept. Partitioning also maps directly to Apache Kafka partitions as well.
The binder currently uses the Apache Kafka kafka-clients
version 2.3.1
.
This client can communicate with older brokers (see the Kafka documentation), but certain features may not be available.
For example, with versions earlier than 0.11.x.x, native headers are not supported.
Also, 0.11.x.x does not support the autoAddPartitions
property.
1.3. Configuration Options
This section contains the configuration options used by the Apache Kafka binder.
For common configuration options and properties pertaining to the binder, see the binding properties in core documentation.
1.3.1. Kafka Binder Properties
- spring.cloud.stream.kafka.binder.brokers
-
A list of brokers to which the Kafka binder connects.
Default:
localhost
. - spring.cloud.stream.kafka.binder.defaultBrokerPort
-
brokers
allows hosts specified with or without port information (for example,host1,host2:port2
). This sets the default port when no port is configured in the broker list.Default:
9092
. - spring.cloud.stream.kafka.binder.configuration
-
Key/Value map of client properties (both producers and consumer) passed to all clients created by the binder. Due to the fact that these properties are used by both producers and consumers, usage should be restricted to common properties — for example, security settings. Unknown Kafka producer or consumer properties provided through this configuration are filtered out and not allowed to propagate. Properties here supersede any properties set in boot.
Default: Empty map.
- spring.cloud.stream.kafka.binder.consumerProperties
-
Key/Value map of arbitrary Kafka client consumer properties. In addition to support known Kafka consumer properties, unknown consumer properties are allowed here as well. Properties here supersede any properties set in boot and in the
configuration
property above.Default: Empty map.
- spring.cloud.stream.kafka.binder.headers
-
The list of custom headers that are transported by the binder. Only required when communicating with older applications (⇐ 1.3.x) with a
kafka-clients
version < 0.11.0.0. Newer versions support headers natively.Default: empty.
- spring.cloud.stream.kafka.binder.healthTimeout
-
The time to wait to get partition information, in seconds. Health reports as down if this timer expires.
Default: 10.
- spring.cloud.stream.kafka.binder.requiredAcks
-
The number of required acks on the broker. See the Kafka documentation for the producer
acks
property.Default:
1
. - spring.cloud.stream.kafka.binder.minPartitionCount
-
Effective only if
autoCreateTopics
orautoAddPartitions
is set. The global minimum number of partitions that the binder configures on topics on which it produces or consumes data. It can be superseded by thepartitionCount
setting of the producer or by the value ofinstanceCount * concurrency
settings of the producer (if either is larger).Default:
1
. - spring.cloud.stream.kafka.binder.producerProperties
-
Key/Value map of arbitrary Kafka client producer properties. In addition to support known Kafka producer properties, unknown producer properties are allowed here as well. Properties here supersede any properties set in boot and in the
configuration
property above.Default: Empty map.
- spring.cloud.stream.kafka.binder.replicationFactor
-
The replication factor of auto-created topics if
autoCreateTopics
is active. Can be overridden on each binding.If you are using Kafka broker versions prior to 2.4, then this value should be set to at least 1
. Starting with version 3.0.8, the binder uses-1
as the default value, which indicates that the broker 'default.replication.factor' property will be used to determine the number of replicas. Check with your Kafka broker admins to see if there is a policy in place that requires a minimum replication factor, if that’s the case then, typically, thedefault.replication.factor
will match that value and-1
should be used, unless you need a replication factor greater than the minimum.Default:
-1
. - spring.cloud.stream.kafka.binder.autoCreateTopics
-
If set to
true
, the binder creates new topics automatically. If set tofalse
, the binder relies on the topics being already configured. In the latter case, if the topics do not exist, the binder fails to start.This setting is independent of the auto.create.topics.enable
setting of the broker and does not influence it. If the server is set to auto-create topics, they may be created as part of the metadata retrieval request, with default broker settings.Default:
true
. - spring.cloud.stream.kafka.binder.autoAddPartitions
-
If set to
true
, the binder creates new partitions if required. If set tofalse
, the binder relies on the partition size of the topic being already configured. If the partition count of the target topic is smaller than the expected value, the binder fails to start.Default:
false
. - spring.cloud.stream.kafka.binder.transaction.transactionIdPrefix
-
Enables transactions in the binder. See
transaction.id
in the Kafka documentation and Transactions in thespring-kafka
documentation. When transactions are enabled, individualproducer
properties are ignored and all producers use thespring.cloud.stream.kafka.binder.transaction.producer.*
properties.Default
null
(no transactions) - spring.cloud.stream.kafka.binder.transaction.producer.*
-
Global producer properties for producers in a transactional binder. See
spring.cloud.stream.kafka.binder.transaction.transactionIdPrefix
and Kafka Producer Properties and the general producer properties supported by all binders.Default: See individual producer properties.
- spring.cloud.stream.kafka.binder.headerMapperBeanName
-
The bean name of a
KafkaHeaderMapper
used for mappingspring-messaging
headers to and from Kafka headers. Use this, for example, if you wish to customize the trusted packages in aBinderHeaderMapper
bean that uses JSON deserialization for the headers. If this customBinderHeaderMapper
bean is not made available to the binder using this property, then the binder will look for a header mapper bean with the namekafkaBinderHeaderMapper
that is of typeBinderHeaderMapper
before falling back to a defaultBinderHeaderMapper
created by the binder.Default: none.
- spring.cloud.stream.kafka.binder.considerDownWhenAnyPartitionHasNoLeader
-
Flag to set the binder health as
down
, when any partitions on the topic, regardless of the consumer that is receiving data from it, is found without a leader.Default:
false
. - spring.cloud.stream.kafka.binder.certificateStoreDirectory
-
When the truststore or keystore certificate location is given as a classpath URL (
classpath:…
), the binder copies the resource from the classpath location inside the JAR file to a location on the filesystem. The file will be moved to the location specified as the value for this property which must be an existing directory on the filesystem that is writable by the process running the application. If this value is not set and the certificate file is a classpath resource, then it will be moved to System’s temp directory as returned bySystem.getProperty("java.io.tmpdir")
. This is also true, if this value is present, but the directory cannot be found on the filesystem or is not writable.Default: none.
1.3.2. Kafka Consumer Properties
To avoid repetition, Spring Cloud Stream supports setting values for all channels, in the format of spring.cloud.stream.kafka.default.consumer.<property>=<value> .
|
The following properties are available for Kafka consumers only and
must be prefixed with spring.cloud.stream.kafka.bindings.<channelName>.consumer.
.
- admin.configuration
-
Since version 2.1.1, this property is deprecated in favor of
topic.properties
, and support for it will be removed in a future version. - admin.replicas-assignment
-
Since version 2.1.1, this property is deprecated in favor of
topic.replicas-assignment
, and support for it will be removed in a future version. - admin.replication-factor
-
Since version 2.1.1, this property is deprecated in favor of
topic.replication-factor
, and support for it will be removed in a future version. - autoRebalanceEnabled
-
When
true
, topic partitions is automatically rebalanced between the members of a consumer group. Whenfalse
, each consumer is assigned a fixed set of partitions based onspring.cloud.stream.instanceCount
andspring.cloud.stream.instanceIndex
. This requires both thespring.cloud.stream.instanceCount
andspring.cloud.stream.instanceIndex
properties to be set appropriately on each launched instance. The value of thespring.cloud.stream.instanceCount
property must typically be greater than 1 in this case.Default:
true
. - ackEachRecord
-
When
autoCommitOffset
istrue
, this setting dictates whether to commit the offset after each record is processed. By default, offsets are committed after all records in the batch of records returned byconsumer.poll()
have been processed. The number of records returned by a poll can be controlled with themax.poll.records
Kafka property, which is set through the consumerconfiguration
property. Setting this totrue
may cause a degradation in performance, but doing so reduces the likelihood of redelivered records when a failure occurs. Also, see the binderrequiredAcks
property, which also affects the performance of committing offsets. This property is deprecated as of 3.1 in favor of usingackMode
. If theackMode
is not set and batch mode is not enabled,RECORD
ackMode will be used.Default:
false
. - autoCommitOffset
-
Starting with version 3.1, this property is deprecated. See
ackMode
for more details on alternatives. Whether to autocommit offsets when a message has been processed. If set tofalse
, a header with the keykafka_acknowledgment
of the typeorg.springframework.kafka.support.Acknowledgment
header is present in the inbound message. Applications may use this header for acknowledging messages. See the examples section for details. When this property is set tofalse
, Kafka binder sets the ack mode toorg.springframework.kafka.listener.AbstractMessageListenerContainer.AckMode.MANUAL
and the application is responsible for acknowledging records. Also seeackEachRecord
.Default:
true
. - ackMode
-
Specify the container ack mode. This is based on the AckMode enumeration defined in Spring Kafka. If
ackEachRecord
property is set totrue
and consumer is not in batch mode, then this will use the ack mode ofRECORD
, otherwise, use the provided ack mode using this property. - autoCommitOnError
-
In pollable consumers, if set to
true
, it always auto commits on error. If not set (the default) or false, it will not auto commit in pollable consumers. Note that this property is only applicable for pollable consumers.Default: not set.
- resetOffsets
-
Whether to reset offsets on the consumer to the value provided by startOffset. Must be false if a
KafkaBindingRebalanceListener
is provided; see Using a KafkaBindingRebalanceListener. See Resetting Offsets for more information about this property.Default:
false
. - startOffset
-
The starting offset for new groups. Allowed values:
earliest
andlatest
. If the consumer group is set explicitly for the consumer 'binding' (throughspring.cloud.stream.bindings.<channelName>.group
), 'startOffset' is set toearliest
. Otherwise, it is set tolatest
for theanonymous
consumer group. See Resetting Offsets for more information about this property.Default: null (equivalent to
earliest
). - enableDlq
-
When set to true, it enables DLQ behavior for the consumer. By default, messages that result in errors are forwarded to a topic named
error.<destination>.<group>
. The DLQ topic name can be configurable by setting thedlqName
property or by defining a@Bean
of typeDlqDestinationResolver
. This provides an alternative option to the more common Kafka replay scenario for the case when the number of errors is relatively small and replaying the entire original topic may be too cumbersome. See Dead-Letter Topic Processing processing for more information. Starting with version 2.0, messages sent to the DLQ topic are enhanced with the following headers:x-original-topic
,x-exception-message
, andx-exception-stacktrace
asbyte[]
. By default, a failed record is sent to the same partition number in the DLQ topic as the original record. See Dead-Letter Topic Partition Selection for how to change that behavior. Not allowed whendestinationIsPattern
istrue
.Default:
false
. - dlqPartitions
-
When
enableDlq
is true, and this property is not set, a dead letter topic with the same number of partitions as the primary topic(s) is created. Usually, dead-letter records are sent to the same partition in the dead-letter topic as the original record. This behavior can be changed; see Dead-Letter Topic Partition Selection. If this property is set to1
and there is noDqlPartitionFunction
bean, all dead-letter records will be written to partition0
. If this property is greater than1
, you MUST provide aDlqPartitionFunction
bean. Note that the actual partition count is affected by the binder’sminPartitionCount
property.Default:
none
- configuration
-
Map with a key/value pair containing generic Kafka consumer properties. In addition to having Kafka consumer properties, other configuration properties can be passed here. For example some properties needed by the application such as
spring.cloud.stream.kafka.bindings.input.consumer.configuration.foo=bar
. Thebootstrap.servers
property cannot be set here; use multi-binder support if you need to connect to multiple clusters.Default: Empty map.
- dlqName
-
The name of the DLQ topic to receive the error messages.
Default: null (If not specified, messages that result in errors are forwarded to a topic named
error.<destination>.<group>
). - dlqProducerProperties
-
Using this, DLQ-specific producer properties can be set. All the properties available through kafka producer properties can be set through this property. When native decoding is enabled on the consumer (i.e., useNativeDecoding: true) , the application must provide corresponding key/value serializers for DLQ. This must be provided in the form of
dlqProducerProperties.configuration.key.serializer
anddlqProducerProperties.configuration.value.serializer
.Default: Default Kafka producer properties.
- standardHeaders
-
Indicates which standard headers are populated by the inbound channel adapter. Allowed values:
none
,id
,timestamp
, orboth
. Useful if using native deserialization and the first component to receive a message needs anid
(such as an aggregator that is configured to use a JDBC message store).Default:
none
- converterBeanName
-
The name of a bean that implements
RecordMessageConverter
. Used in the inbound channel adapter to replace the defaultMessagingMessageConverter
.Default:
null
- idleEventInterval
-
The interval, in milliseconds, between events indicating that no messages have recently been received. Use an
ApplicationListener<ListenerContainerIdleEvent>
to receive these events. See Example: Pausing and Resuming the Consumer for a usage example.Default:
30000
- destinationIsPattern
-
When true, the destination is treated as a regular expression
Pattern
used to match topic names by the broker. When true, topics are not provisioned, andenableDlq
is not allowed, because the binder does not know the topic names during the provisioning phase. Note, the time taken to detect new topics that match the pattern is controlled by the consumer propertymetadata.max.age.ms
, which (at the time of writing) defaults to 300,000ms (5 minutes). This can be configured using theconfiguration
property above.Default:
false
- topic.properties
-
A
Map
of Kafka topic properties used when provisioning new topics — for example,spring.cloud.stream.kafka.bindings.input.consumer.topic.properties.message.format.version=0.9.0.0
Default: none.
- topic.replicas-assignment
-
A Map<Integer, List<Integer>> of replica assignments, with the key being the partition and the value being the assignments. Used when provisioning new topics. See the
NewTopic
Javadocs in thekafka-clients
jar.Default: none.
- topic.replication-factor
-
The replication factor to use when provisioning topics. Overrides the binder-wide setting. Ignored if
replicas-assignments
is present.Default: none (the binder-wide default of -1 is used).
- pollTimeout
-
Timeout used for polling in pollable consumers.
Default: 5 seconds.
- transactionManager
-
Bean name of a
KafkaAwareTransactionManager
used to override the binder’s transaction manager for this binding. Usually needed if you want to synchronize another transaction with the Kafka transaction, using theChainedKafkaTransactionManaager
. To achieve exactly once consumption and production of records, the consumer and producer bindings must all be configured with the same transaction manager.Default: none.
- txCommitRecovered
-
When using a transactional binder, the offset of a recovered record (e.g. when retries are exhausted and the record is sent to a dead letter topic) will be committed via a new transaction, by default. Setting this property to
false
suppresses committing the offset of recovered record.Default: true.
1.3.3. Resetting Offsets
When an application starts, the initial position in each assigned partition depends on two properties startOffset
and resetOffsets
.
If resetOffsets
is false
, normal Kafka consumer auto.offset.reset
semantics apply.
i.e. If there is no committed offset for a partition for the binding’s consumer group, the position is earliest
or latest
.
By default, bindings with an explicit group
use earliest
, and anonymous bindings (with no group
) use latest
.
These defaults can be overridden by setting the startOffset
binding property.
There will be no committed offset(s) the first time the binding is started with a particular group
.
The other condition where no committed offset exists is if the offset has been expired.
With modern brokers (since 2.1), and default broker properties, the offsets are expired 7 days after the last member leaves the group.
See the offsets.retention.minutes
broker property for more information.
When resetOffsets
is true
, the binder applies similar semantics to those that apply when there is no committed offset on the broker, as if this binding has never consumed from the topic; i.e. any current committed offset is ignored.
Following are two use cases when this might be used.
-
Consuming from a compacted topic containing key/value pairs. Set
resetOffsets
totrue
andstartOffset
toearliest
; the binding will perform aseekToBeginning
on all newly assigned partitions. -
Consuming from a topic containing events, where you are only interested in events that occur while this binding is running. Set
resetOffsets
totrue
andstartOffset
tolatest
; the binding will perform aseekToEnd
on all newly assigned partitions.
If a rebalance occurs after the initial assignment, the seeks will only be performed on any newly assigned partitions that were not assigned during the initial assignment. |
For more control over topic offsets, see Using a KafkaBindingRebalanceListener; when a listener is provided, resetOffsets
should not be set to true
, otherwise, that will cause an error.
1.3.4. Consuming Batches
Starting with version 3.0, when spring.cloud.stream.binding.<name>.consumer.batch-mode
is set to true
, all of the records received by polling the Kafka Consumer
will be presented as a List<?>
to the listener method.
Otherwise, the method will be called with one record at a time.
The size of the batch is controlled by Kafka consumer properties max.poll.records
, fetch.min.bytes
, fetch.max.wait.ms
; refer to the Kafka documentation for more information.
Bear in mind that batch mode is not supported with @StreamListener
- it only works with the newer functional programming model.
Retry within the binder is not supported when using batch mode, so maxAttempts will be overridden to 1.
You can configure a SeekToCurrentBatchErrorHandler (using a ListenerContainerCustomizer ) to achieve similar functionality to retry in the binder.
You can also use a manual AckMode and call Ackowledgment.nack(index, sleep) to commit the offsets for a partial batch and have the remaining records redelivered.
Refer to the Spring for Apache Kafka documentation for more information about these techniques.
|
1.3.5. Kafka Producer Properties
To avoid repetition, Spring Cloud Stream supports setting values for all channels, in the format of spring.cloud.stream.kafka.default.producer.<property>=<value> .
|
The following properties are available for Kafka producers only and
must be prefixed with spring.cloud.stream.kafka.bindings.<channelName>.producer.
.
- admin.configuration
-
Since version 2.1.1, this property is deprecated in favor of
topic.properties
, and support for it will be removed in a future version. - admin.replicas-assignment
-
Since version 2.1.1, this property is deprecated in favor of
topic.replicas-assignment
, and support for it will be removed in a future version. - admin.replication-factor
-
Since version 2.1.1, this property is deprecated in favor of
topic.replication-factor
, and support for it will be removed in a future version. - bufferSize
-
Upper limit, in bytes, of how much data the Kafka producer attempts to batch before sending.
Default:
16384
. - sync
-
Whether the producer is synchronous.
Default:
false
. - sendTimeoutExpression
-
A SpEL expression evaluated against the outgoing message used to evaluate the time to wait for ack when synchronous publish is enabled — for example,
headers['mySendTimeout']
. The value of the timeout is in milliseconds. With versions before 3.0, the payload could not be used unless native encoding was being used because, by the time this expression was evaluated, the payload was already in the form of abyte[]
. Now, the expression is evaluated before the payload is converted.Default:
none
. - batchTimeout
-
How long the producer waits to allow more messages to accumulate in the same batch before sending the messages. (Normally, the producer does not wait at all and simply sends all the messages that accumulated while the previous send was in progress.) A non-zero value may increase throughput at the expense of latency.
Default:
0
. - messageKeyExpression
-
A SpEL expression evaluated against the outgoing message used to populate the key of the produced Kafka message — for example,
headers['myKey']
. With versions before 3.0, the payload could not be used unless native encoding was being used because, by the time this expression was evaluated, the payload was already in the form of abyte[]
. Now, the expression is evaluated before the payload is converted. In the case of a regular processor (Function<String, String>
orFunction<Message<?>, Message<?>
), if the produced key needs to be same as the incoming key from the topic, this property can be set as below.spring.cloud.stream.kafka.bindings.<output-binding-name>.producer.messageKeyExpression: headers['kafka_receivedMessageKey']
There is an important caveat to keep in mind for reactive functions. In that case, it is up to the application to manually copy the headers from the incoming messages to outbound messages. You can set the header, e.g.myKey
and useheaders['myKey']
as suggested above or, for convenience, simply set theKafkaHeaders.MESSAGE_KEY
header, and you do not need to set this property at all.Default:
none
. - headerPatterns
-
A comma-delimited list of simple patterns to match Spring messaging headers to be mapped to the Kafka
Headers
in theProducerRecord
. Patterns can begin or end with the wildcard character (asterisk). Patterns can be negated by prefixing with!
. Matching stops after the first match (positive or negative). For example!ask,as*
will passash
but notask
.id
andtimestamp
are never mapped.Default:
*
(all headers - except theid
andtimestamp
) - configuration
-
Map with a key/value pair containing generic Kafka producer properties. The
bootstrap.servers
property cannot be set here; use multi-binder support if you need to connect to multiple clusters.Default: Empty map.
- topic.properties
-
A
Map
of Kafka topic properties used when provisioning new topics — for example,spring.cloud.stream.kafka.bindings.output.producer.topic.properties.message.format.version=0.9.0.0
- topic.replicas-assignment
-
A Map<Integer, List<Integer>> of replica assignments, with the key being the partition and the value being the assignments. Used when provisioning new topics. See the
NewTopic
Javadocs in thekafka-clients
jar.Default: none.
- topic.replication-factor
-
The replication factor to use when provisioning topics. Overrides the binder-wide setting. Ignored if
replicas-assignments
is present.Default: none (the binder-wide default of -1 is used).
- useTopicHeader
-
Set to
true
to override the default binding destination (topic name) with the value of theKafkaHeaders.TOPIC
message header in the outbound message. If the header is not present, the default binding destination is used.Default:
false
. - recordMetadataChannel
-
The bean name of a
MessageChannel
to which successful send results should be sent; the bean must exist in the application context. The message sent to the channel is the sent message (after conversion, if any) with an additional headerKafkaHeaders.RECORD_METADATA
. The header contains aRecordMetadata
object provided by the Kafka client; it includes the partition and offset where the record was written in the topic.ResultMetadata meta = sendResultMsg.getHeaders().get(KafkaHeaders.RECORD_METADATA, RecordMetadata.class)
Failed sends go the producer error channel (if configured); see Error Channels.
Default: null.
The Kafka binder uses the partitionCount setting of the producer as a hint to create a topic with the given partition count (in conjunction with the minPartitionCount , the maximum of the two being the value being used).
Exercise caution when configuring both minPartitionCount for a binder and partitionCount for an application, as the larger value is used.
If a topic already exists with a smaller partition count and autoAddPartitions is disabled (the default), the binder fails to start.
If a topic already exists with a smaller partition count and autoAddPartitions is enabled, new partitions are added.
If a topic already exists with a larger number of partitions than the maximum of (minPartitionCount or partitionCount ), the existing partition count is used.
|
- compression
-
Set the
compression.type
producer property. Supported values arenone
,gzip
,snappy
,lz4
andzstd
. If you override thekafka-clients
jar to 2.1.0 (or later), as discussed in the Spring for Apache Kafka documentation, and wish to usezstd
compression, usespring.cloud.stream.kafka.bindings.<binding-name>.producer.configuration.compression.type=zstd
.Default:
none
. - transactionManager
-
Bean name of a
KafkaAwareTransactionManager
used to override the binder’s transaction manager for this binding. Usually needed if you want to synchronize another transaction with the Kafka transaction, using theChainedKafkaTransactionManaager
. To achieve exactly once consumption and production of records, the consumer and producer bindings must all be configured with the same transaction manager.Default: none.
- closeTimeout
-
Timeout in number of seconds to wait for when closing the producer.
Default:
30
- allowNonTransactional
-
Normally, all output bindings associated with a transactional binder will publish in a new transaction, if one is not already in process. This property allows you to override that behavior. If set to true, records published to this output binding will not be run in a transaction, unless one is already in process.
Default:
false
1.3.6. Usage examples
In this section, we show the use of the preceding properties for specific scenarios.
Example: Setting ackMode
to MANUAL
and Relying on Manual Acknowledgement
This example illustrates how one may manually acknowledge offsets in a consumer application.
This example requires that spring.cloud.stream.kafka.bindings.input.consumer.ackMode
be set to MANUAL
.
Use the corresponding input channel name for your example.
@SpringBootApplication
@EnableBinding(Sink.class)
public class ManuallyAcknowdledgingConsumer {
public static void main(String[] args) {
SpringApplication.run(ManuallyAcknowdledgingConsumer.class, args);
}
@StreamListener(Sink.INPUT)
public void process(Message<?> message) {
Acknowledgment acknowledgment = message.getHeaders().get(KafkaHeaders.ACKNOWLEDGMENT, Acknowledgment.class);
if (acknowledgment != null) {
System.out.println("Acknowledgment provided");
acknowledgment.acknowledge();
}
}
}
Example: Security Configuration
Apache Kafka 0.9 supports secure connections between client and brokers.
To take advantage of this feature, follow the guidelines in the Apache Kafka Documentation as well as the Kafka 0.9 security guidelines from the Confluent documentation.
Use the spring.cloud.stream.kafka.binder.configuration
option to set security properties for all clients created by the binder.
For example, to set security.protocol
to SASL_SSL
, set the following property:
spring.cloud.stream.kafka.binder.configuration.security.protocol=SASL_SSL
All the other security properties can be set in a similar manner.
When using Kerberos, follow the instructions in the reference documentation for creating and referencing the JAAS configuration.
Spring Cloud Stream supports passing JAAS configuration information to the application by using a JAAS configuration file and using Spring Boot properties.
Using JAAS Configuration Files
The JAAS and (optionally) krb5 file locations can be set for Spring Cloud Stream applications by using system properties. The following example shows how to launch a Spring Cloud Stream application with SASL and Kerberos by using a JAAS configuration file:
java -Djava.security.auth.login.config=/path.to/kafka_client_jaas.conf -jar log.jar \
--spring.cloud.stream.kafka.binder.brokers=secure.server:9092 \
--spring.cloud.stream.bindings.input.destination=stream.ticktock \
--spring.cloud.stream.kafka.binder.configuration.security.protocol=SASL_PLAINTEXT
Using Spring Boot Properties
As an alternative to having a JAAS configuration file, Spring Cloud Stream provides a mechanism for setting up the JAAS configuration for Spring Cloud Stream applications by using Spring Boot properties.
The following properties can be used to configure the login context of the Kafka client:
- spring.cloud.stream.kafka.binder.jaas.loginModule
-
The login module name. Not necessary to be set in normal cases.
Default:
com.sun.security.auth.module.Krb5LoginModule
. - spring.cloud.stream.kafka.binder.jaas.controlFlag
-
The control flag of the login module.
Default:
required
. - spring.cloud.stream.kafka.binder.jaas.options
-
Map with a key/value pair containing the login module options.
Default: Empty map.
The following example shows how to launch a Spring Cloud Stream application with SASL and Kerberos by using Spring Boot configuration properties:
java --spring.cloud.stream.kafka.binder.brokers=secure.server:9092 \
--spring.cloud.stream.bindings.input.destination=stream.ticktock \
--spring.cloud.stream.kafka.binder.autoCreateTopics=false \
--spring.cloud.stream.kafka.binder.configuration.security.protocol=SASL_PLAINTEXT \
--spring.cloud.stream.kafka.binder.jaas.options.useKeyTab=true \
--spring.cloud.stream.kafka.binder.jaas.options.storeKey=true \
--spring.cloud.stream.kafka.binder.jaas.options.keyTab=/etc/security/keytabs/kafka_client.keytab \
--spring.cloud.stream.kafka.binder.jaas.options.principal=kafka-client-1@EXAMPLE.COM
The preceding example represents the equivalent of the following JAAS file:
KafkaClient {
com.sun.security.auth.module.Krb5LoginModule required
useKeyTab=true
storeKey=true
keyTab="/etc/security/keytabs/kafka_client.keytab"
principal="[email protected]";
};
If the topics required already exist on the broker or will be created by an administrator, autocreation can be turned off and only client JAAS properties need to be sent.
Do not mix JAAS configuration files and Spring Boot properties in the same application.
If the -Djava.security.auth.login.config system property is already present, Spring Cloud Stream ignores the Spring Boot properties.
|
Be careful when using the autoCreateTopics and autoAddPartitions with Kerberos.
Usually, applications may use principals that do not have administrative rights in Kafka and Zookeeper.
Consequently, relying on Spring Cloud Stream to create/modify topics may fail.
In secure environments, we strongly recommend creating topics and managing ACLs administratively by using Kafka tooling.
|
Multi-binder configuration and JAAS
When connecting to multiple clusters in which each one requires separate JAAS configuration, then set the JAAS configuration using the property sasl.jaas.config
.
When this property is present in the applicaiton, it takes precedence over the other strategies mentioned above.
See this KIP-85 for more details.
For example, if you have two clusters in your application with separate JAAS configuration, then the following is a template that you can use:
spring.cloud.stream:
binders:
kafka1:
type: kafka
environment:
spring:
cloud:
stream:
kafka:
binder:
brokers: localhost:9092
configuration.sasl.jaas.config: "org.apache.kafka.common.security.plain.PlainLoginModule required username=\"admin\" password=\"admin-secret\";"
kafka2:
type: kafka
environment:
spring:
cloud:
stream:
kafka:
binder:
brokers: localhost:9093
configuration.sasl.jaas.config: "org.apache.kafka.common.security.plain.PlainLoginModule required username=\"user1\" password=\"user1-secret\";"
kafka.binder:
configuration:
security.protocol: SASL_PLAINTEXT
sasl.mechanism: PLAIN
Note that both the Kafka clusters, and the sasl.jaas.config
values for each of them are different in the above configuration.
See this sample application for more details on how to setup and run such an application.
Example: Pausing and Resuming the Consumer
If you wish to suspend consumption but not cause a partition rebalance, you can pause and resume the consumer.
This is facilitated by adding the Consumer
as a parameter to your @StreamListener
.
To resume, you need an ApplicationListener
for ListenerContainerIdleEvent
instances.
The frequency at which events are published is controlled by the idleEventInterval
property.
Since the consumer is not thread-safe, you must call these methods on the calling thread.
The following simple application shows how to pause and resume:
@SpringBootApplication
@EnableBinding(Sink.class)
public class Application {
public static void main(String[] args) {
SpringApplication.run(Application.class, args);
}
@StreamListener(Sink.INPUT)
public void in(String in, @Header(KafkaHeaders.CONSUMER) Consumer<?, ?> consumer) {
System.out.println(in);
consumer.pause(Collections.singleton(new TopicPartition("myTopic", 0)));
}
@Bean
public ApplicationListener<ListenerContainerIdleEvent> idleListener() {
return event -> {
System.out.println(event);
if (event.getConsumer().paused().size() > 0) {
event.getConsumer().resume(event.getConsumer().paused());
}
};
}
}
1.4. Transactional Binder
Enable transactions by setting spring.cloud.stream.kafka.binder.transaction.transactionIdPrefix
to a non-empty value, e.g. tx-
.
When used in a processor application, the consumer starts the transaction; any records sent on the consumer thread participate in the same transaction.
When the listener exits normally, the listener container will send the offset to the transaction and commit it.
A common producer factory is used for all producer bindings configured using spring.cloud.stream.kafka.binder.transaction.producer.*
properties; individual binding Kafka producer properties are ignored.
Normal binder retries (and dead lettering) are not supported with transactions because the retries will run in the original transaction, which may be rolled back and any published records will be rolled back too.
When retries are enabled (the common property maxAttempts is greater than zero) the retry properties are used to configure a DefaultAfterRollbackProcessor to enable retries at the container level.
Similarly, instead of publishing dead-letter records within the transaction, this functionality is moved to the listener container, again via the DefaultAfterRollbackProcessor which runs after the main transaction has rolled back.
|
If you wish to use transactions in a source application, or from some arbitrary thread for producer-only transaction (e.g. @Scheduled
method), you must get a reference to the transactional producer factory and define a KafkaTransactionManager
bean using it.
@Bean
public PlatformTransactionManager transactionManager(BinderFactory binders,
@Value("${unique.tx.id.per.instance}") String txId) {
ProducerFactory<byte[], byte[]> pf = ((KafkaMessageChannelBinder) binders.getBinder(null,
MessageChannel.class)).getTransactionalProducerFactory();
KafkaTransactionManager tm = new KafkaTransactionManager<>(pf);
tm.setTransactionId(txId)
return tm;
}
Notice that we get a reference to the binder using the BinderFactory
; use null
in the first argument when there is only one binder configured.
If more than one binder is configured, use the binder name to get the reference.
Once we have a reference to the binder, we can obtain a reference to the ProducerFactory
and create a transaction manager.
Then you would use normal Spring transaction support, e.g. TransactionTemplate
or @Transactional
, for example:
public static class Sender {
@Transactional
public void doInTransaction(MessageChannel output, List<String> stuffToSend) {
stuffToSend.forEach(stuff -> output.send(new GenericMessage<>(stuff)));
}
}
If you wish to synchronize producer-only transactions with those from some other transaction manager, use a ChainedTransactionManager
.
If you deploy multiple instances of your application, each instance needs a unique transactionIdPrefix .
|
1.5. Error Channels
Starting with version 1.3, the binder unconditionally sends exceptions to an error channel for each consumer destination and can also be configured to send async producer send failures to an error channel. See this section on error handling for more information.
The payload of the ErrorMessage
for a send failure is a KafkaSendFailureException
with properties:
-
failedMessage
: The Spring MessagingMessage<?>
that failed to be sent. -
record
: The rawProducerRecord
that was created from thefailedMessage
There is no automatic handling of producer exceptions (such as sending to a Dead-Letter queue). You can consume these exceptions with your own Spring Integration flow.
1.6. Kafka Metrics
Kafka binder module exposes the following metrics:
spring.cloud.stream.binder.kafka.offset
: This metric indicates how many messages have not been yet consumed from a given binder’s topic by a given consumer group.
The metrics provided are based on the Micrometer library.
The binder creates the KafkaBinderMetrics
bean if Micrometer is on the classpath and no other such beans provided by the application.
The metric contains the consumer group information, topic and the actual lag in committed offset from the latest offset on the topic.
This metric is particularly useful for providing auto-scaling feedback to a PaaS platform.
You can exclude KafkaBinderMetrics
from creating the necessary infrastructure like consumers and then reporting the metrics by providing the following component in the application.
@Component
class NoOpBindingMeters {
NoOpBindingMeters(MeterRegistry registry) {
registry.config().meterFilter(
MeterFilter.denyNameStartsWith(KafkaBinderMetrics.OFFSET_LAG_METRIC_NAME));
}
}
More details on how to suppress meters selectively can be found here.
1.7. Tombstone Records (null record values)
When using compacted topics, a record with a null
value (also called a tombstone record) represents the deletion of a key.
To receive such messages in a @StreamListener
method, the parameter must be marked as not required to receive a null
value argument.
@StreamListener(Sink.INPUT)
public void in(@Header(KafkaHeaders.RECEIVED_MESSAGE_KEY) byte[] key,
@Payload(required = false) Customer customer) {
// customer is null if a tombstone record
...
}
1.8. Using a KafkaBindingRebalanceListener
Applications may wish to seek topics/partitions to arbitrary offsets when the partitions are initially assigned, or perform other operations on the consumer.
Starting with version 2.1, if you provide a single KafkaBindingRebalanceListener
bean in the application context, it will be wired into all Kafka consumer bindings.
public interface KafkaBindingRebalanceListener {
/**
* Invoked by the container before any pending offsets are committed.
* @param bindingName the name of the binding.
* @param consumer the consumer.
* @param partitions the partitions.
*/
default void onPartitionsRevokedBeforeCommit(String bindingName, Consumer<?, ?> consumer,
Collection<TopicPartition> partitions) {
}
/**
* Invoked by the container after any pending offsets are committed.
* @param bindingName the name of the binding.
* @param consumer the consumer.
* @param partitions the partitions.
*/
default void onPartitionsRevokedAfterCommit(String bindingName, Consumer<?, ?> consumer, Collection<TopicPartition> partitions) {
}
/**
* Invoked when partitions are initially assigned or after a rebalance.
* Applications might only want to perform seek operations on an initial assignment.
* @param bindingName the name of the binding.
* @param consumer the consumer.
* @param partitions the partitions.
* @param initial true if this is the initial assignment.
*/
default void onPartitionsAssigned(String bindingName, Consumer<?, ?> consumer, Collection<TopicPartition> partitions,
boolean initial) {
}
}
You cannot set the resetOffsets
consumer property to true
when you provide a rebalance listener.
1.9. Customizing Consumer and Producer configuration
If you want advanced customization of consumer and producer configuration that is used for creating ConsumerFactory
and ProducerFactory
in Kafka,
you can implement the following customizers.
-
ConsumerConfigCustomizer
-
ProducerConfigCustomizer
Both of these interfaces provide a way to configure the config map used for consumer and producer properties.
For example, if you want to gain access to a bean that is defined at the application level, you can inject that in the implementation of the configure
method.
When the binder discovers that these customizers are available as beans, it will invoke the configure
method right before creating the consumer and producer factories.
Both of these interfaces also provide access to both the binding and destination names so that they can be accessed while customizing producer and consumer properties.
1.10. Customizing AdminClient Configuration
As with consumer and producer config customization above, applications can also customize the configuration for admin clients by providing an AdminClientConfigCustomizer
.
AdminClientConfigCustomizer’s configure method provides access to the admin client properties, using which you can define further customization.
Binder’s Kafka topic provisioner gives the highest precedence for the properties given through this customizer.
Here is an example of providing this customizer bean.
@Bean
public AdminClientConfigCustomizer adminClientConfigCustomizer() {
return props -> {
props.put(CommonClientConfigs.SECURITY_PROTOCOL_CONFIG, "SASL_SSL");
};
}
1.11. Dead-Letter Topic Processing
1.11.1. Dead-Letter Topic Partition Selection
By default, records are published to the Dead-Letter topic using the same partition as the original record. This means the Dead-Letter topic must have at least as many partitions as the original record.
To change this behavior, add a DlqPartitionFunction
implementation as a @Bean
to the application context.
Only one such bean can be present.
The function is provided with the consumer group, the failed ConsumerRecord
and the exception.
For example, if you always want to route to partition 0, you might use:
@Bean
public DlqPartitionFunction partitionFunction() {
return (group, record, ex) -> 0;
}
If you set a consumer binding’s dlqPartitions property to 1 (and the binder’s minPartitionCount is equal to 1 ), there is no need to supply a DlqPartitionFunction ; the framework will always use partition 0.
If you set a consumer binding’s dlqPartitions property to a value greater than 1 (or the binder’s minPartitionCount is greater than 1 ), you must provide a DlqPartitionFunction bean, even if the partition count is the same as the original topic’s.
|
It is also possible to define a custom name for the DLQ topic.
In order to do so, create an implementation of DlqDestinationResolver
as a @Bean
to the application context.
When the binder detects such a bean, that takes precedence, otherwise it will use the dlqName
property.
If neither of these are found, it will default to error.<destination>.<group>
.
Here is an example of DlqDestinationResolver
as a @Bean
.
@Bean
public DlqDestinationResolver dlqDestinationResolver() {
return (rec, ex) -> {
if (rec.topic().equals("word1")) {
return "topic1-dlq";
}
else {
return "topic2-dlq";
}
};
}
One important thing to keep in mind when providing an implementation for DlqDestinationResolver
is that the provisioner in the binder will not auto create topics for the application.
This is because there is no way for the binder to infer the names of all the DLQ topics the implementation might send to.
Therefore, if you provide DLQ names using this strategy, it is the application’s responsibility to ensure that those topics are created beforehand.
1.11.2. Handling Records in a Dead-Letter Topic
Because the framework cannot anticipate how users would want to dispose of dead-lettered messages, it does not provide any standard mechanism to handle them. If the reason for the dead-lettering is transient, you may wish to route the messages back to the original topic. However, if the problem is a permanent issue, that could cause an infinite loop. The sample Spring Boot application within this topic is an example of how to route those messages back to the original topic, but it moves them to a “parking lot” topic after three attempts. The application is another spring-cloud-stream application that reads from the dead-letter topic. It exits when no messages are received for 5 seconds.
The examples assume the original destination is so8400out
and the consumer group is so8400
.
There are a couple of strategies to consider:
-
Consider running the rerouting only when the main application is not running. Otherwise, the retries for transient errors are used up very quickly.
-
Alternatively, use a two-stage approach: Use this application to route to a third topic and another to route from there back to the main topic.
The following code listings show the sample application:
spring.cloud.stream.bindings.input.group=so8400replay
spring.cloud.stream.bindings.input.destination=error.so8400out.so8400
spring.cloud.stream.bindings.output.destination=so8400out
spring.cloud.stream.bindings.parkingLot.destination=so8400in.parkingLot
spring.cloud.stream.kafka.binder.configuration.auto.offset.reset=earliest
spring.cloud.stream.kafka.binder.headers=x-retries
@SpringBootApplication
@EnableBinding(TwoOutputProcessor.class)
public class ReRouteDlqKApplication implements CommandLineRunner {
private static final String X_RETRIES_HEADER = "x-retries";
public static void main(String[] args) {
SpringApplication.run(ReRouteDlqKApplication.class, args).close();
}
private final AtomicInteger processed = new AtomicInteger();
@Autowired
private MessageChannel parkingLot;
@StreamListener(Processor.INPUT)
@SendTo(Processor.OUTPUT)
public Message<?> reRoute(Message<?> failed) {
processed.incrementAndGet();
Integer retries = failed.getHeaders().get(X_RETRIES_HEADER, Integer.class);
if (retries == null) {
System.out.println("First retry for " + failed);
return MessageBuilder.fromMessage(failed)
.setHeader(X_RETRIES_HEADER, new Integer(1))
.setHeader(BinderHeaders.PARTITION_OVERRIDE,
failed.getHeaders().get(KafkaHeaders.RECEIVED_PARTITION_ID))
.build();
}
else if (retries.intValue() < 3) {
System.out.println("Another retry for " + failed);
return MessageBuilder.fromMessage(failed)
.setHeader(X_RETRIES_HEADER, new Integer(retries.intValue() + 1))
.setHeader(BinderHeaders.PARTITION_OVERRIDE,
failed.getHeaders().get(KafkaHeaders.RECEIVED_PARTITION_ID))
.build();
}
else {
System.out.println("Retries exhausted for " + failed);
parkingLot.send(MessageBuilder.fromMessage(failed)
.setHeader(BinderHeaders.PARTITION_OVERRIDE,
failed.getHeaders().get(KafkaHeaders.RECEIVED_PARTITION_ID))
.build());
}
return null;
}
@Override
public void run(String... args) throws Exception {
while (true) {
int count = this.processed.get();
Thread.sleep(5000);
if (count == this.processed.get()) {
System.out.println("Idle, exiting");
return;
}
}
}
public interface TwoOutputProcessor extends Processor {
@Output("parkingLot")
MessageChannel parkingLot();
}
}
1.12. Partitioning with the Kafka Binder
Apache Kafka supports topic partitioning natively.
Sometimes it is advantageous to send data to specific partitions — for example, when you want to strictly order message processing (all messages for a particular customer should go to the same partition).
The following example shows how to configure the producer and consumer side:
@SpringBootApplication
@EnableBinding(Source.class)
public class KafkaPartitionProducerApplication {
private static final Random RANDOM = new Random(System.currentTimeMillis());
private static final String[] data = new String[] {
"foo1", "bar1", "qux1",
"foo2", "bar2", "qux2",
"foo3", "bar3", "qux3",
"foo4", "bar4", "qux4",
};
public static void main(String[] args) {
new SpringApplicationBuilder(KafkaPartitionProducerApplication.class)
.web(false)
.run(args);
}
@InboundChannelAdapter(channel = Source.OUTPUT, poller = @Poller(fixedRate = "5000"))
public Message<?> generate() {
String value = data[RANDOM.nextInt(data.length)];
System.out.println("Sending: " + value);
return MessageBuilder.withPayload(value)
.setHeader("partitionKey", value)
.build();
}
}
spring:
cloud:
stream:
bindings:
output:
destination: partitioned.topic
producer:
partition-key-expression: headers['partitionKey']
partition-count: 12
The topic must be provisioned to have enough partitions to achieve the desired concurrency for all consumer groups.
The above configuration supports up to 12 consumer instances (6 if their concurrency is 2, 4 if their concurrency is 3, and so on).
It is generally best to “over-provision” the partitions to allow for future increases in consumers or concurrency.
|
The preceding configuration uses the default partitioning (key.hashCode() % partitionCount ).
This may or may not provide a suitably balanced algorithm, depending on the key values.
You can override this default by using the partitionSelectorExpression or partitionSelectorClass properties.
|
Since partitions are natively handled by Kafka, no special configuration is needed on the consumer side. Kafka allocates partitions across the instances.
The following Spring Boot application listens to a Kafka stream and prints (to the console) the partition ID to which each message goes:
@SpringBootApplication
@EnableBinding(Sink.class)
public class KafkaPartitionConsumerApplication {
public static void main(String[] args) {
new SpringApplicationBuilder(KafkaPartitionConsumerApplication.class)
.web(false)
.run(args);
}
@StreamListener(Sink.INPUT)
public void listen(@Payload String in, @Header(KafkaHeaders.RECEIVED_PARTITION_ID) int partition) {
System.out.println(in + " received from partition " + partition);
}
}
spring:
cloud:
stream:
bindings:
input:
destination: partitioned.topic
group: myGroup
You can add instances as needed.
Kafka rebalances the partition allocations.
If the instance count (or instance count * concurrency
) exceeds the number of partitions, some consumers are idle.
2. Kafka Streams Binder
2.1. Usage
For using the Kafka Streams binder, you just need to add it to your Spring Cloud Stream application, using the following maven coordinates:
<dependency>
<groupId>org.springframework.cloud</groupId>
<artifactId>spring-cloud-stream-binder-kafka-streams</artifactId>
</dependency>
A quick way to bootstrap a new project for Kafka Streams binder is to use Spring Initializr and then select "Cloud Streams" and "Spring for Kafka Streams" as shown below
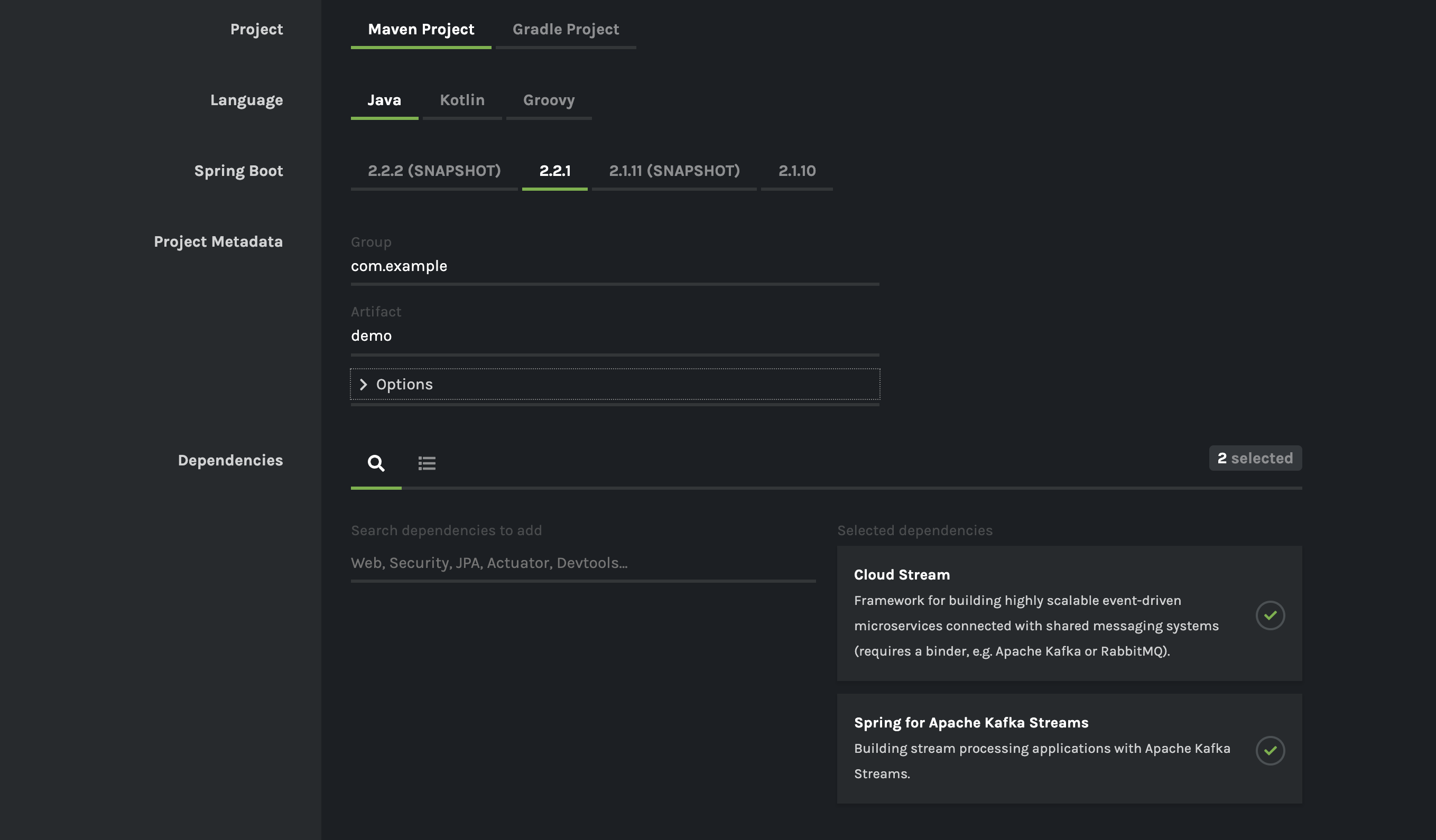
2.2. Overview
Spring Cloud Stream includes a binder implementation designed explicitly for Apache Kafka Streams binding. With this native integration, a Spring Cloud Stream "processor" application can directly use the Apache Kafka Streams APIs in the core business logic.
Kafka Streams binder implementation builds on the foundations provided by the Spring for Apache Kafka project.
Kafka Streams binder provides binding capabilities for the three major types in Kafka Streams - KStream
, KTable
and GlobalKTable
.
Kafka Streams applications typically follow a model in which the records are read from an inbound topic, apply business logic, and then write the transformed records to an outbound topic. Alternatively, a Processor application with no outbound destination can be defined as well.
In the following sections, we are going to look at the details of Spring Cloud Stream’s integration with Kafka Streams.
2.3. Programming Model
When using the programming model provided by Kafka Streams binder, both the high-level Streams DSL and a mix of both the higher level and the lower level Processor-API can be used as options.
When mixing both higher and lower level API’s, this is usually achieved by invoking transform
or process
API methods on KStream
.
2.3.1. Functional Style
Starting with Spring Cloud Stream 3.0.0
, Kafka Streams binder allows the applications to be designed and developed using the functional programming style that is available in Java 8.
This means that the applications can be concisely represented as a lambda expression of types java.util.function.Function
or java.util.function.Consumer
.
Let’s take a very basic example.
@SpringBootApplication
public class SimpleConsumerApplication {
@Bean
public java.util.function.Consumer<KStream<Object, String>> process() {
return input ->
input.foreach((key, value) -> {
System.out.println("Key: " + key + " Value: " + value);
});
}
}
Albeit simple, this is a complete standalone Spring Boot application that is leveraging Kafka Streams for stream processing.
This is a consumer application with no outbound binding and only a single inbound binding.
The application consumes data and it simply logs the information from the KStream
key and value on the standard output.
The application contains the SpringBootApplication
annotation and a method that is marked as Bean
.
The bean method is of type java.util.function.Consumer
which is parameterized with KStream
.
Then in the implementation, we are returning a Consumer object that is essentially a lambda expression.
Inside the lambda expression, the code for processing the data is provided.
In this application, there is a single input binding that is of type KStream
.
The binder creates this binding for the application with a name process-in-0
, i.e. the name of the function bean name followed by a dash character (-
) and the literal in
followed by another dash and then the ordinal position of the parameter.
You use this binding name to set other properties such as destination.
For example, spring.cloud.stream.bindings.process-in-0.destination=my-topic
.
If the destination property is not set on the binding, a topic is created with the same name as the binding (if there are sufficient privileges for the application) or that topic is expected to be already available. |
Once built as a uber-jar (e.g., kstream-consumer-app.jar
), you can run the above example like the following.
If the applications choose to define the functional beans using Spring’s Component
annotation, the binder also suppports that model.
The above functional bean could be rewritten as below.
@Component(name = "process")
public class SimpleConsumer implements java.util.function.Consumer<KStream<Object, String>> {
@Override
public void accept(KStream<Object, String> input) {
input.foreach((key, value) -> {
System.out.println("Key: " + key + " Value: " + value);
});
}
}
java -jar kstream-consumer-app.jar --spring.cloud.stream.bindings.process-in-0.destination=my-topic
Here is another example, where it is a full processor with both input and output bindings. This is the classic word-count example in which the application receives data from a topic, the number of occurrences for each word is then computed in a tumbling time-window.
@SpringBootApplication
public class WordCountProcessorApplication {
@Bean
public Function<KStream<Object, String>, KStream<?, WordCount>> process() {
return input -> input
.flatMapValues(value -> Arrays.asList(value.toLowerCase().split("\\W+")))
.map((key, value) -> new KeyValue<>(value, value))
.groupByKey(Serialized.with(Serdes.String(), Serdes.String()))
.windowedBy(TimeWindows.of(5000))
.count(Materialized.as("word-counts-state-store"))
.toStream()
.map((key, value) -> new KeyValue<>(key.key(), new WordCount(key.key(), value,
new Date(key.window().start()), new Date(key.window().end()))));
}
public static void main(String[] args) {
SpringApplication.run(WordCountProcessorApplication.class, args);
}
}
Here again, this is a complete Spring Boot application. The difference here from the first application is that the bean method is of type java.util.function.Function
.
The first parameterized type for the Function
is for the input KStream
and the second one is for the output.
In the method body, a lambda expression is provided that is of type Function
and as implementation, the actual business logic is given.
Similar to the previously discussed Consumer based application, the input binding here is named as process-in-0
by default. For the output, the binding name is automatically also set to process-out-0
.
Once built as an uber-jar (e.g., wordcount-processor.jar
), you can run the above example like the following.
java -jar wordcount-processor.jar --spring.cloud.stream.bindings.process-in-0.destination=words --spring.cloud.stream.bindings.process-out-0.destination=counts
This application will consume messages from the Kafka topic words
and the computed results are published to an output
topic counts
.
Spring Cloud Stream will ensure that the messages from both the incoming and outgoing topics are automatically bound as KStream objects. As a developer, you can exclusively focus on the business aspects of the code, i.e. writing the logic required in the processor. Setting up Kafka Streams specific configuration required by the Kafka Streams infrastructure is automatically handled by the framework.
The two examples we saw above have a single KStream
input binding. In both cases, the bindings received the records from a single topic.
If you want to multiplex multiple topics into a single KStream
binding, you can provide comma separated Kafka topics as destinations below.
spring.cloud.stream.bindings.process-in-0.destination=topic-1,topic-2,topic-3
In addition, you can also provide topic patterns as destinations if you want to match topics against a regular exression.
spring.cloud.stream.bindings.process-in-0.destination=input.*
Multiple Input Bindings
Many non-trivial Kafka Streams applications often consume data from more than one topic through multiple bindings.
For instance, one topic is consumed as Kstream
and another as KTable
or GlobalKTable
.
There are many reasons why an application might want to receive data as a table type.
Think of a use-case where the underlying topic is populated through a change data capture (CDC) mechanism from a database or perhaps the application only cares about the latest updates for downstream processing.
If the application specifies that the data needs to be bound as KTable
or GlobalKTable
, then Kafka Streams binder will properly bind the destination to a KTable
or GlobalKTable
and make them available for the application to operate upon.
We will look at a few different scenarios how multiple input bindings are handled in the Kafka Streams binder.
BiFunction in Kafka Streams Binder
Here is an example where we have two inputs and an output. In this case, the application can leverage on java.util.function.BiFunction
.
@Bean
public BiFunction<KStream<String, Long>, KTable<String, String>, KStream<String, Long>> process() {
return (userClicksStream, userRegionsTable) -> (userClicksStream
.leftJoin(userRegionsTable, (clicks, region) -> new RegionWithClicks(region == null ?
"UNKNOWN" : region, clicks),
Joined.with(Serdes.String(), Serdes.Long(), null))
.map((user, regionWithClicks) -> new KeyValue<>(regionWithClicks.getRegion(),
regionWithClicks.getClicks()))
.groupByKey(Grouped.with(Serdes.String(), Serdes.Long()))
.reduce(Long::sum)
.toStream());
}
Here again, the basic theme is the same as in the previous examples, but here we have two inputs.
Java’s BiFunction
support is used to bind the inputs to the desired destinations.
The default binding names generated by the binder for the inputs are process-in-0
and process-in-1
respectively. The default output binding is process-out-0
.
In this example, the first parameter of BiFunction
is bound as a KStream
for the first input and the second parameter is bound as a KTable
for the second input.
BiConsumer in Kafka Streams Binder
If there are two inputs, but no outputs, in that case we can use java.util.function.BiConsumer
as shown below.
@Bean
public BiConsumer<KStream<String, Long>, KTable<String, String>> process() {
return (userClicksStream, userRegionsTable) -> {}
}
Beyond two inputs
What if you have more than two inputs? There are situations in which you need more than two inputs. In that case, the binder allows you to chain partial functions. In functional programming jargon, this technique is generally known as currying. With the functional programming support added as part of Java 8, Java now enables you to write curried functions. Spring Cloud Stream Kafka Streams binder can make use of this feature to enable multiple input bindings.
Let’s see an example.
@Bean
public Function<KStream<Long, Order>,
Function<GlobalKTable<Long, Customer>,
Function<GlobalKTable<Long, Product>, KStream<Long, EnrichedOrder>>>> enrichOrder() {
return orders -> (
customers -> (
products -> (
orders.join(customers,
(orderId, order) -> order.getCustomerId(),
(order, customer) -> new CustomerOrder(customer, order))
.join(products,
(orderId, customerOrder) -> customerOrder
.productId(),
(customerOrder, product) -> {
EnrichedOrder enrichedOrder = new EnrichedOrder();
enrichedOrder.setProduct(product);
enrichedOrder.setCustomer(customerOrder.customer);
enrichedOrder.setOrder(customerOrder.order);
return enrichedOrder;
})
)
)
);
}
Let’s look at the details of the binding model presented above.
In this model, we have 3 partially applied functions on the inbound. Let’s call them as f(x)
, f(y)
and f(z)
.
If we expand these functions in the sense of true mathematical functions, it will look like these: f(x) → (fy) → f(z) → KStream<Long, EnrichedOrder>
.
The x
variable stands for KStream<Long, Order>
, the y
variable stands for GlobalKTable<Long, Customer>
and the z
variable stands for GlobalKTable<Long, Product>
.
The first function f(x)
has the first input binding of the application (KStream<Long, Order>
) and its output is the function, f(y).
The function f(y)
has the second input binding for the application (GlobalKTable<Long, Customer>
) and its output is yet another function, f(z)
.
The input for the function f(z)
is the third input for the application (GlobalKTable<Long, Product>
) and its output is KStream<Long, EnrichedOrder>
which is the final output binding for the application.
The input from the three partial functions which are KStream
, GlobalKTable
, GlobalKTable
respectively are available for you in the method body for implementing the business logic as part of the lambda expression.
Input bindings are named as enrichOrder-in-0
, enrichOrder-in-1
and enrichOrder-in-2
respectively. Output binding is named as enrichOrder-out-0
.
With curried functions, you can virtually have any number of inputs. However, keep in mind that, anything more than a smaller number of inputs and partially applied functions for them as above in Java might lead to unreadable code. Therefore if your Kafka Streams application requires more than a reasonably smaller number of input bindings, and you want to use this functional model, then you may want to rethink your design and decompose the application appropriately.
Output Bindings
Kafka Streams binder allows types of either KStream
or KTable
as output bindings.
Behind the scenes, the binder uses the to
method on KStream
to send the resultant records to the output topic.
If the application provides a KTable
as output in the function, the binder still uses this technique by delegating to the to
method of KStream
.
For example both functions below will work:
@Bean
public Function<KStream<String, String>, KTable<String, String>> foo() {
return KStream::toTable;
};
}
@Bean
public Function<KTable<String, String>, KStream<String, String>> bar() {
return KTable::toStream;
}
Multiple Output Bindings
Kafka Streams allows to write outbound data into multiple topics. This feature is known as branching in Kafka Streams.
When using multiple output bindings, you need to provide an array of KStream (KStream[]
) as the outbound return type.
Here is an example:
@Bean
public Function<KStream<Object, String>, KStream<?, WordCount>[]> process() {
Predicate<Object, WordCount> isEnglish = (k, v) -> v.word.equals("english");
Predicate<Object, WordCount> isFrench = (k, v) -> v.word.equals("french");
Predicate<Object, WordCount> isSpanish = (k, v) -> v.word.equals("spanish");
return input -> input
.flatMapValues(value -> Arrays.asList(value.toLowerCase().split("\\W+")))
.groupBy((key, value) -> value)
.windowedBy(TimeWindows.of(5000))
.count(Materialized.as("WordCounts-branch"))
.toStream()
.map((key, value) -> new KeyValue<>(null, new WordCount(key.key(), value,
new Date(key.window().start()), new Date(key.window().end()))))
.branch(isEnglish, isFrench, isSpanish);
}
The programming model remains the same, however the outbound parameterized type is KStream[]
.
The default output binding names are process-out-0
, process-out-1
, process-out-2
respectively.
The reason why the binder generates three output bindings is because it detects the length of the returned KStream
array.
Summary of Function based Programming Styles for Kafka Streams
In summary, the following table shows the various options that can be used in the functional paradigm.
Number of Inputs | Number of Outputs | Component to use |
---|---|---|
1 |
0 |
java.util.function.Consumer |
2 |
0 |
java.util.function.BiConsumer |
1 |
1..n |
java.util.function.Function |
2 |
1..n |
java.util.function.BiFunction |
>= 3 |
0..n |
Use curried functions |
-
In the case of more than one output in this table, the type simply becomes
KStream[]
.
Function composition in Kafka Streams binder
Kafka Streams binder supports minimal forms of functional composition for linear topologies.
Using the Java functional API support, you can write multiple functions and then compose them on your own using the andThen
method.
For example, assume that you have the following two functions.
@Bean
public Function<KStream<String, String>, KStream<String, String>> foo() {
return input -> input.peek((s, s2) -> {});
}
@Bean
public Function<KStream<String, String>, KStream<String, Long>> bar() {
return input -> input.peek((s, s2) -> {});
}
Even without the functional composition support in the binder, you can compose these two functions as below.
@Bean
pubic Funcion<KStream<String, String>, KStream<String, Long>> composed() {
foo().andThen(bar());
}
Then you can provide deefinitions of the form spring.cloud.stream.function.definition=foo;bar;composed
.
With the functional composition support in the binder, you don’t need to write this third function in which you are doing explicit function composition.
You can simply do this instead:
spring.cloud.stream.function.definition=foo|bar
You can even do this:
spring.cloud.stream.function.definition=foo|bar;foo;bar
The composed function’s default binding names in this example becomes foobar-in-0
and foobar-out-0
.
Limitations of functional composition in Kafka Streams bincer
When you have java.util.function.Function
bean, that can be composed with another function or multiple functions.
The same function bean can be composed with a java.util.function.Consumer
as well. In this case, consumer is the last component composed.
A function can be composed with multiple functions, then end with a java.util.function.Consumer
bean as well.
When composing the beans of type java.util.function.BiFunction
, the BiFunction
must be the first function in the definition.
The composed entities must be either of type java.util.function.Function
or java.util.funciton.Consumer
.
In other words, you cannot take a BiFunction
bean and then compose with another BiFunction
.
You cannot compose with types of BiConsumer
or definitions where Consumer
is the first component.
You cannot also compose with functions where the output is an array (KStream[]
for branching) unless this is the last component in the definition.
The very first Function
of BiFunction
in the function definition may use a curried form also.
For example, the following is possible.
@Bean
public Function<KStream<String, String>, Function<KTable<String, String>, KStream<String, String>>> curriedFoo() {
return a -> b ->
a.join(b, (value1, value2) -> value1 + value2);
}
@Bean
public Function<KStream<String, String>, KStream<String, String>> bar() {
return input -> input.mapValues(value -> value + "From-anotherFooFunc");
}
and the function definition could be curriedFoo|bar
.
Behind the scenes, the binder will create two input bindings for the curried function, and an output binding based on the final function in the definition.
The default input bindings in this case are going to be curriedFoobar-in-0
and curriedFoobar-in-1
.
The default output binding for this example becomes curriedFoobar-out-0
.
Special note on using KTable
as output in function composition
Lets say you have the following two functions.
@Bean
public Function<KStream<String, String>, KTable<String, String>> foo() {
return KStream::toTable;
};
}
@Bean
public Function<KTable<String, String>, KStream<String, String>> bar() {
return KTable::toStream;
}
You can compose them as foo|bar
, but keep in mind that the second function (bar
in this case) must have a KTable
as input since the first function (foo
) has KTable
as output.
2.3.2. Imperative programming model.
Starting with 3.1.0
version of the binder, we recommend using the functional programming model described above for Kafka Streams binder based applications.
The support for StreamListener
is deprecated starting with 3.1.0
of Spring Cloud Stream.
Below, we are providing some details on the StreamListener
based Kafka Streams processors as a reference.
Following is the equivalent of the Word count example using StreamListener
.
@SpringBootApplication
@EnableBinding(KafkaStreamsProcessor.class)
public class WordCountProcessorApplication {
@StreamListener("input")
@SendTo("output")
public KStream<?, WordCount> process(KStream<?, String> input) {
return input
.flatMapValues(value -> Arrays.asList(value.toLowerCase().split("\\W+")))
.groupBy((key, value) -> value)
.windowedBy(TimeWindows.of(5000))
.count(Materialized.as("WordCounts-multi"))
.toStream()
.map((key, value) -> new KeyValue<>(null, new WordCount(key.key(), value, new Date(key.window().start()), new Date(key.window().end()))));
}
public static void main(String[] args) {
SpringApplication.run(WordCountProcessorApplication.class, args);
}
As you can see, this is a bit more verbose since you need to provide EnableBinding
and the other extra annotations like StreamListener
and SendTo
to make it a complete application.
EnableBinding
is where you specify your binding interface that contains your bindings.
In this case, we are using the stock KafkaStreamsProcessor
binding interface that has the following contracts.
public interface KafkaStreamsProcessor {
@Input("input")
KStream<?, ?> input();
@Output("output")
KStream<?, ?> output();
}
Binder will create bindings for the input KStream
and output KStream
since you are using a binding interface that contains those declarations.
In addition to the obvious differences in the programming model offered in the functional style, one particular thing that needs to be mentioned here is that the binding names are what you specify in the binding interface.
For example, in the above application, since we are using KafkaStreamsProcessor
, the binding names are input
and output
.
Binding properties need to use those names. For instance spring.cloud.stream.bindings.input.destination
, spring.cloud.stream.bindings.output.destination
etc.
Keep in mind that this is fundamentally different from the functional style since there the binder generates binding names for the application.
This is because the application does not provide any binding interfaces in the functional model using EnableBinding
.
Here is another example of a sink where we have two inputs.
@EnableBinding(KStreamKTableBinding.class)
.....
.....
@StreamListener
public void process(@Input("inputStream") KStream<String, PlayEvent> playEvents,
@Input("inputTable") KTable<Long, Song> songTable) {
....
....
}
interface KStreamKTableBinding {
@Input("inputStream")
KStream<?, ?> inputStream();
@Input("inputTable")
KTable<?, ?> inputTable();
}
Following is the StreamListener
equivalent of the same BiFunction
based processor that we saw above.
@EnableBinding(KStreamKTableBinding.class)
....
....
@StreamListener
@SendTo("output")
public KStream<String, Long> process(@Input("input") KStream<String, Long> userClicksStream,
@Input("inputTable") KTable<String, String> userRegionsTable) {
....
....
}
interface KStreamKTableBinding extends KafkaStreamsProcessor {
@Input("inputX")
KTable<?, ?> inputTable();
}
Finally, here is the StreamListener
equivalent of the application with three inputs and curried functions.
@EnableBinding(CustomGlobalKTableProcessor.class)
...
...
@StreamListener
@SendTo("output")
public KStream<Long, EnrichedOrder> process(
@Input("input-1") KStream<Long, Order> ordersStream,
@Input("input-2") GlobalKTable<Long, Customer> customers,
@Input("input-3") GlobalKTable<Long, Product> products) {
KStream<Long, CustomerOrder> customerOrdersStream = ordersStream.join(
customers, (orderId, order) -> order.getCustomerId(),
(order, customer) -> new CustomerOrder(customer, order));
return customerOrdersStream.join(products,
(orderId, customerOrder) -> customerOrder.productId(),
(customerOrder, product) -> {
EnrichedOrder enrichedOrder = new EnrichedOrder();
enrichedOrder.setProduct(product);
enrichedOrder.setCustomer(customerOrder.customer);
enrichedOrder.setOrder(customerOrder.order);
return enrichedOrder;
});
}
interface CustomGlobalKTableProcessor {
@Input("input-1")
KStream<?, ?> input1();
@Input("input-2")
GlobalKTable<?, ?> input2();
@Input("input-3")
GlobalKTable<?, ?> input3();
@Output("output")
KStream<?, ?> output();
}
You might notice that the above two examples are even more verbose since in addition to provide EnableBinding
, you also need to write your own custom binding interface as well.
Using the functional model, you can avoid all those ceremonial details.
Before we move on from looking at the general programming model offered by Kafka Streams binder, here is the StreamListener
version of multiple output bindings.
EnableBinding(KStreamProcessorWithBranches.class)
public static class WordCountProcessorApplication {
@Autowired
private TimeWindows timeWindows;
@StreamListener("input")
@SendTo({"output1","output2","output3"})
public KStream<?, WordCount>[] process(KStream<Object, String> input) {
Predicate<Object, WordCount> isEnglish = (k, v) -> v.word.equals("english");
Predicate<Object, WordCount> isFrench = (k, v) -> v.word.equals("french");
Predicate<Object, WordCount> isSpanish = (k, v) -> v.word.equals("spanish");
return input
.flatMapValues(value -> Arrays.asList(value.toLowerCase().split("\\W+")))
.groupBy((key, value) -> value)
.windowedBy(timeWindows)
.count(Materialized.as("WordCounts-1"))
.toStream()
.map((key, value) -> new KeyValue<>(null, new WordCount(key.key(), value, new Date(key.window().start()), new Date(key.window().end()))))
.branch(isEnglish, isFrench, isSpanish);
}
interface KStreamProcessorWithBranches {
@Input("input")
KStream<?, ?> input();
@Output("output1")
KStream<?, ?> output1();
@Output("output2")
KStream<?, ?> output2();
@Output("output3")
KStream<?, ?> output3();
}
}
To recap, we have reviewed the various programming model choices when using the Kafka Streams binder.
The binder provides binding capabilities for KStream
, KTable
and GlobalKTable
on the input.
KTable
and GlobalKTable
bindings are only available on the input.
Binder supports both input and output bindings for KStream
.
The upshot of the programming model of Kafka Streams binder is that the binder provides you the flexibility of going with a fully functional programming model or using the StreamListener
based imperative approach.
2.4. Ancillaries to the programming model
2.4.1. Multiple Kafka Streams processors within a single application
Binder allows to have multiple Kafka Streams processors within a single Spring Cloud Stream application. You can have an application as below.
@Bean
public java.util.function.Function<KStream<Object, String>, KStream<Object, String>> process() {
...
}
@Bean
public java.util.function.Consumer<KStream<Object, String>> anotherProcess() {
...
}
@Bean
public java.util.function.BiFunction<KStream<Object, String>, KTable<Integer, String>, KStream<Object, String>> yetAnotherProcess() {
...
}
In this case, the binder will create 3 separate Kafka Streams objects with different application ID’s (more on this below). However, if you have more than one processor in the application, you have to tell Spring Cloud Stream, which functions need to be activated. Here is how you activate the functions.
spring.cloud.stream.function.definition: process;anotherProcess;yetAnotherProcess
If you want certain functions to be not activated right away, you can remove that from this list.
This is also true when you have a single Kafka Streams processor and other types of Function
beans in the same application that is handled through a different binder (for e.g., a function bean that is based on the regular Kafka Message Channel binder)
2.4.2. Kafka Streams Application ID
Application id is a mandatory property that you need to provide for a Kafka Streams application. Spring Cloud Stream Kafka Streams binder allows you to configure this application id in multiple ways.
If you only have one single processor or StreamListener
in the application, then you can set this at the binder level using the following property:
spring.cloud.stream.kafka.streams.binder.applicationId
.
As a convenience, if you only have a single processor, you can also use spring.application.name
as the property to delegate the application id.
If you have multiple Kafka Streams processors in the application, then you need to set the application id per processor. In the case of the functional model, you can attach it to each function as a property.
For e.g. imagine that you have the following functions.
@Bean
public java.util.function.Consumer<KStream<Object, String>> process() {
...
}
and
@Bean
public java.util.function.Consumer<KStream<Object, String>> anotherProcess() {
...
}
Then you can set the application id for each, using the following binder level properties.
spring.cloud.stream.kafka.streams.binder.functions.process.applicationId
and
spring.cloud.stream.kafka.streams.binder.functions.anotherProcess.applicationId
In the case of StreamListener
, you need to set this on the first input binding on the processor.
For e.g. imagine that you have the following two StreamListener
based processors.
@StreamListener
@SendTo("output")
public KStream<String, String> process(@Input("input") <KStream<Object, String>> input) {
...
}
@StreamListener
@SendTo("anotherOutput")
public KStream<String, String> anotherProcess(@Input("anotherInput") <KStream<Object, String>> input) {
...
}
Then you must set the application id for this using the following binding property.
spring.cloud.stream.kafka.streams.bindings.input.consumer.applicationId
and
spring.cloud.stream.kafka.streams.bindings.anotherInput.consumer.applicationId
For function based model also, this approach of setting application id at the binding level will work. However, setting per function at the binder level as we have seen above is much easier if you are using the functional model.
For production deployments, it is highly recommended to explicitly specify the application ID through configuration. This is especially going to be very critical if you are auto scaling your application in which case you need to make sure that you are deploying each instance with the same application ID.
If the application does not provide an application ID, then in that case the binder will auto generate a static application ID for you.
This is convenient in development scenarios as it avoids the need for explicitly providing the application ID.
The generated application ID in this manner will be static over application restarts.
In the case of functional model, the generated application ID will be the function bean name followed by the literal applicationID
, for e.g process-applicationID
if process
if the function bean name.
In the case of StreamListener
, instead of using the function bean name, the generated application ID will be use the containing class name followed by the method name followed by the literal applicationId
.
Summary of setting Application ID
-
By default, binder will auto generate the application ID per function or
StreamListener
methods. -
If you have a single processor, then you can use
spring.kafka.streams.applicationId
,spring.application.name
orspring.cloud.stream.kafka.streams.binder.applicationId
. -
If you have multiple processors, then application ID can be set per function using the property -
spring.cloud.stream.kafka.streams.binder.functions.<function-name>.applicationId
. In the case ofStreamListener
, this can be done usingspring.cloud.stream.kafka.streams.bindings.input.applicationId
, assuming that the input binding name isinput
.
2.4.3. Overriding the default binding names generated by the binder with the functional style
By default, the binder uses the strategy discussed above to generate the binding name when using the functional style, i.e. <function-bean-name>-<in>|<out>-[0..n], for e.g. process-in-0, process-out-0 etc. If you want to override those binding names, you can do that by specifying the following properties.
spring.cloud.stream.function.bindings.<default binding name>
. Default binding name is the original binding name generated by the binder.
For e.g. lets say, you have this function.
@Bean
public BiFunction<KStream<String, Long>, KTable<String, String>, KStream<String, Long>> process() {
...
}
Binder will generate bindings with names, process-in-0
, process-in-1
and process-out-0
.
Now, if you want to change them to something else completely, maybe more domain specific binding names, then you can do so as below.
spring.cloud.stream.function.bindings.process-in-0=users
spring.cloud.stream.function.bindings.process-in-0=regions
and
spring.cloud.stream.function.bindings.process-out-0=clicks
After that, you must set all the binding level properties on these new binding names.
Please keep in mind that with the functional programming model described above, adhering to the default binding names make sense in most situations. The only reason you may still want to do this overriding is when you have larger number of configuration properties and you want to map the bindings to something more domain friendly.
2.4.4. Setting up bootstrap server configuration
When running Kafka Streams applications, you must provide the Kafka broker server information.
If you don’t provide this information, the binder expects that you are running the broker at the default localhost:9092
.
If that is not the case, then you need to override that. There are a couple of ways to do that.
-
Using the boot property -
spring.kafka.bootstrapServers
-
Binder level property -
spring.cloud.stream.kafka.streams.binder.brokers
When it comes to the binder level property, it doesn’t matter if you use the broker property provided through the regular Kafka binder - spring.cloud.stream.kafka.binder.brokers
.
Kafka Streams binder will first check if Kafka Streams binder specific broker property is set (spring.cloud.stream.kafka.streams.binder.brokers
) and if not found, it looks for spring.cloud.stream.kafka.binder.brokers
.
2.5. Record serialization and deserialization
Kafka Streams binder allows you to serialize and deserialize records in two ways. One is the native serialization and deserialization facilities provided by Kafka and the other one is the message conversion capabilities of Spring Cloud Stream framework. Lets look at some details.
2.5.1. Inbound deserialization
Keys are always deserialized using native Serdes.
For values, by default, deserialization on the inbound is natively performed by Kafka. Please note that this is a major change on default behavior from previous versions of Kafka Streams binder where the deserialization was done by the framework.
Kafka Streams binder will try to infer matching Serde
types by looking at the type signature of java.util.function.Function|Consumer
or StreamListener
.
Here is the order that it matches the Serdes.
-
If the application provides a bean of type
Serde
and if the return type is parameterized with the actual type of the incoming key or value type, then it will use thatSerde
for inbound deserialization. For e.g. if you have the following in the application, the binder detects that the incoming value type for theKStream
matches with a type that is parameterized on aSerde
bean. It will use that for inbound deserialization.
@Bean
public Serde<Foo() customSerde{
...
}
@Bean
public Function<KStream<String, Foo>, KStream<String, Foo>> process() {
}
-
Next, it looks at the types and see if they are one of the types exposed by Kafka Streams. If so, use them. Here are the Serde types that the binder will try to match from Kafka Streams.
Integer, Long, Short, Double, Float, byte[], UUID and String.
-
If none of the Serdes provided by Kafka Streams don’t match the types, then it will use JsonSerde provided by Spring Kafka. In this case, the binder assumes that the types are JSON friendly. This is useful if you have multiple value objects as inputs since the binder will internally infer them to correct Java types. Before falling back to the
JsonSerde
though, the binder checks at the defaultSerde`s set in the Kafka Streams configuration to see if it is a `Serde
that it can match with the incoming KStream’s types.
If none of the above strategies worked, then the applications must provide the `Serde`s through configuration. This can be configured in two ways - binding or default.
First the binder will look if a Serde
is provided at the binding level.
For e.g. if you have the following processor,
@Bean
public BiFunction<KStream<CustomKey, AvroIn1>, KTable<CustomKey, AvroIn2>, KStream<CustomKey, AvroOutput>> process() {...}
then, you can provide a binding level Serde
using the following:
spring.cloud.stream.kafka.streams.bindings.process-in-0.consumer.keySerde=CustomKeySerde
spring.cloud.stream.kafka.streams.bindings.process-in-0.consumer.valueSerde=io.confluent.kafka.streams.serdes.avro.SpecificAvroSerde
spring.cloud.stream.kafka.streams.bindings.process-in-1.consumer.keySerde=CustomKeySerde
spring.cloud.stream.kafka.streams.bindings.process-in-1.consumer.valueSerde=io.confluent.kafka.streams.serdes.avro.SpecificAvroSerde
If you provide Serde as abover per input binding, then that will takes higher precedence and the binder will stay away from any Serde inference.
|
If you want the default key/value Serdes to be used for inbound deserialization, you can do so at the binder level.
spring.cloud.stream.kafka.streams.binder.configuration.default.key.serde
spring.cloud.stream.kafka.streams.binder.configuration.default.value.serde
If you don’t want the native decoding provided by Kafka, you can rely on the message conversion features that Spring Cloud Stream provides. Since native decoding is the default, in order to let Spring Cloud Stream deserialize the inbound value object, you need to explicitly disable native decoding.
For e.g. if you have the same BiFunction processor as above, then spring.cloud.stream.bindings.process-in-0.consumer.nativeDecoding: false
You need to disable native decoding for all the inputs individually. Otherwise, native decoding will still be applied for those you do not disable.
By default, Spring Cloud Stream will use application/json
as the content type and use an appropriate json message converter.
You can use custom message converters by using the following property and an appropriate MessageConverter
bean.
spring.cloud.stream.bindings.process-in-0.contentType
2.5.2. Outbound serialization
Outbound serialization pretty much follows the same rules as above for inbound deserialization. As with the inbound deserialization, one major change from the previous versions of Spring Cloud Stream is that the serialization on the outbound is handled by Kafka natively. Before 3.0 versions of the binder, this was done by the framework itself.
Keys on the outbound are always serialized by Kafka using a matching Serde
that is inferred by the binder.
If it can’t infer the type of the key, then that needs to be specified using configuration.
Value serdes are inferred using the same rules used for inbound deserialization.
First it matches to see if the outbound type is from a provided bean in the application.
If not, it checks to see if it matches with a Serde
exposed by Kafka such as - Integer
, Long
, Short
, Double
, Float
, byte[]
, UUID
and String
.
If that doesnt’t work, then it falls back to JsonSerde
provided by the Spring Kafka project, but first look at the default Serde
configuration to see if there is a match.
Keep in mind that all these happen transparently to the application.
If none of these work, then the user has to provide the Serde
to use by configuration.
Lets say you are using the same BiFunction
processor as above. Then you can configure outbound key/value Serdes as following.
spring.cloud.stream.kafka.streams.bindings.process-out-0.producer.keySerde=CustomKeySerde
spring.cloud.stream.kafka.streams.bindings.process-out-0.producer.valueSerde=io.confluent.kafka.streams.serdes.avro.SpecificAvroSerde
If Serde inference fails, and no binding level Serdes are provided, then the binder falls back to the JsonSerde
, but look at the default Serdes for a match.
Default serdes are configured in the same way as above where it is described under deserialization.
spring.cloud.stream.kafka.streams.binder.configuration.default.key.serde
spring.cloud.stream.kafka.streams.binder.configuration.default.value.serde
If your application uses the branching feature and has multiple output bindings, then these have to be configured per binding.
Once again, if the binder is capable of inferring the Serde
types, you don’t need to do this configuration.
If you don’t want the native encoding provided by Kafka, but want to use the framework provided message conversion, then you need to explicitly disable native encoding since since native encoding is the default.
For e.g. if you have the same BiFunction processor as above, then spring.cloud.stream.bindings.process-out-0.producer.nativeEncoding: false
You need to disable native encoding for all the output individually in the case of branching. Otherwise, native encoding will still be applied for those you don’t disable.
When conversion is done by Spring Cloud Stream, by default, it will use application/json
as the content type and use an appropriate json message converter.
You can use custom message converters by using the following property and a corresponding MessageConverter
bean.
spring.cloud.stream.bindings.process-out-0.contentType
When native encoding/decoding is disabled, binder will not do any inference as in the case of native Serdes.
Applications need to explicitly provide all the configuration options.
For that reason, it is generally advised to stay with the default options for de/serialization and stick with native de/serialization provided by Kafka Streams when you write Spring Cloud Stream Kafka Streams applications.
The one scenario in which you must use message conversion capabilities provided by the framework is when your upstream producer is using a specific serialization strategy.
In that case, you want to use a matching deserialization strategy as native mechanisms may fail.
When relying on the default Serde
mechanism, the applications must ensure that the binder has a way forward with correctly map the inbound and outbound with a proper Serde
, as otherwise things might fail.
It is worth to mention that the data de/serialization approaches outlined above are only applicable on the edges of your processors, i.e. - inbound and outbound.
Your business logic might still need to call Kafka Streams API’s that explicitly need Serde
objects.
Those are still the responsibility of the application and must be handled accordingly by the developer.
2.6. Error Handling
Apache Kafka Streams provides the capability for natively handling exceptions from deserialization errors.
For details on this support, please see this.
Out of the box, Apache Kafka Streams provides two kinds of deserialization exception handlers - LogAndContinueExceptionHandler
and LogAndFailExceptionHandler
.
As the name indicates, the former will log the error and continue processing the next records and the latter will log the error and fail. LogAndFailExceptionHandler
is the default deserialization exception handler.
2.6.1. Handling Deserialization Exceptions in the Binder
Kafka Streams binder allows to specify the deserialization exception handlers above using the following property.
spring.cloud.stream.kafka.streams.binder.deserializationExceptionHandler: logAndContinue
or
spring.cloud.stream.kafka.streams.binder.deserializationExceptionHandler: logAndFail
In addition to the above two deserialization exception handlers, the binder also provides a third one for sending the erroneous records (poison pills) to a DLQ (dead letter queue) topic. Here is how you enable this DLQ exception handler.
spring.cloud.stream.kafka.streams.binder.deserializationExceptionHandler: sendToDlq
When the above property is set, all the records in deserialization error are automatically sent to the DLQ topic.
You can set the topic name where the DLQ messages are published as below.
You can provide an implementation for DlqDestinationResolver
which is a functional interface.
DlqDestinationResolver
takes ConsumerRecord
and the exception as inputs and then allows to specify a topic name as the output.
By gaining access to the Kafka ConsumerRecord
, the header records can be introspected in the implementation of the BiFunction
.
Here is an example of providing an implementation for DlqDestinationResolver
.
@Bean
public DlqDestinationResolver dlqDestinationResolver() {
return (rec, ex) -> {
if (rec.topic().equals("word1")) {
return "topic1-dlq";
}
else {
return "topic2-dlq";
}
};
}
One important thing to keep in mind when providing an implementation for DlqDestinationResolver
is that the provisioner in the binder will not auto create topics for the application.
This is because there is no way for the binder to infer the names of all the DLQ topics the implementation might send to.
Therefore, if you provide DLQ names using this strategy, it is the application’s responsibility to ensure that those topics are created beforehand.
If DlqDestinationResolver
is present in the application as a bean, that takes higher precedence.
If you do not want to follow this approach and rather provide a static DLQ name using configuration, you can set the following property.
spring.cloud.stream.kafka.streams.bindings.process-in-0.consumer.dlqName: custom-dlq (Change the binding name accordingly)
If this is set, then the error records are sent to the topic custom-dlq
.
If the application is not using either of the above strategies, then it will create a DLQ topic with the name error.<input-topic-name>.<application-id>
.
For instance, if your binding’s destination topic is inputTopic
and the application ID is process-applicationId
, then the default DLQ topic is error.inputTopic.process-applicationId
.
It is always recommended to explicitly create a DLQ topic for each input binding if it is your intention to enable DLQ.
2.6.2. DLQ per input consumer binding
The property spring.cloud.stream.kafka.streams.binder.deserializationExceptionHandler
is applicable for the entire application.
This implies that if there are multiple functions or StreamListener
methods in the same application, this property is applied to all of them.
However, if you have multiple processors or multiple input bindings within a single processor, then you can use the finer-grained DLQ control that the binder provides per input consumer binding.
If you have the following processor,
@Bean
public BiFunction<KStream<String, Long>, KTable<String, String>, KStream<String, Long>> process() {
...
}
and you only want to enable DLQ on the first input binding and skipAndContinue on the second binding, then you can do so on the consumer as below.
spring.cloud.stream.kafka.streams.bindings.process-in-0.consumer.deserializationExceptionHandler: sendToDlq
spring.cloud.stream.kafka.streams.bindings.process-in-1.consumer.deserializationExceptionHandler: skipAndContinue
Setting deserialization exception handlers this way has a higher precedence than setting at the binder level.
2.6.3. DLQ partitioning
By default, records are published to the Dead-Letter topic using the same partition as the original record. This means the Dead-Letter topic must have at least as many partitions as the original record.
To change this behavior, add a DlqPartitionFunction
implementation as a @Bean
to the application context.
Only one such bean can be present.
The function is provided with the consumer group (which is the same as the application ID in most situations), the failed ConsumerRecord
and the exception.
For example, if you always want to route to partition 0, you might use:
@Bean
public DlqPartitionFunction partitionFunction() {
return (group, record, ex) -> 0;
}
If you set a consumer binding’s dlqPartitions property to 1 (and the binder’s minPartitionCount is equal to 1 ), there is no need to supply a DlqPartitionFunction ; the framework will always use partition 0.
If you set a consumer binding’s dlqPartitions property to a value greater than 1 (or the binder’s minPartitionCount is greater than 1 ), you must provide a DlqPartitionFunction bean, even if the partition count is the same as the original topic’s.
|
A couple of things to keep in mind when using the exception handling feature in Kafka Streams binder.
-
The property
spring.cloud.stream.kafka.streams.binder.deserializationExceptionHandler
is applicable for the entire application. This implies that if there are multiple functions orStreamListener
methods in the same application, this property is applied to all of them. -
The exception handling for deserialization works consistently with native deserialization and framework provided message conversion.
2.6.4. Handling Production Exceptions in the Binder
Unlike the support for deserialization exception handlers as described above, the binder does not provide such first class mechanisms for handling production exceptions.
However, you still can configure production exception handlers using the StreamsBuilderFactoryBean
customizer which you can find more details about, in a subsequent section below.
2.7. Retrying critical business logic
There are scenarios in which you might want to retry parts of your business logic that are critical to the application.
There maybe an external call to a relational database or invoking a REST endpoint from the Kafka Streams processor.
These calls can fail for various reasons such as network issues or remote service unavailability.
More often, these failures may self resolve if you can try them again.
By default, Kafka Streams binder creates RetryTemplate
beans for all the input bindings.
If the function has the following signature,
@Bean
public java.util.function.Consumer<KStream<Object, String>> process()
and with default binding name, the RetryTemplate
will be registered as process-in-0-RetryTemplate
.
This is following the convention of binding name (process-in-0
) followed by the literal -RetryTemplate
.
In the case of multiple input bindings, there will be a separate RetryTemplate
bean available per binding.
If there is a custom RetryTemplate
bean available in the application and provided through spring.cloud.stream.bindings.<binding-name>.consumer.retryTemplateName
, then that takes precedence over any input binding level retry template configuration properties.
Once the RetryTemplate
from the binding is injected into the application, it can be used to retry any critical sections of the application.
Here is an example:
@Bean
public java.util.function.Consumer<KStream<Object, String>> process(@Lazy @Qualifier("process-in-0-RetryTemplate") RetryTemplate retryTemplate) {
return input -> input
.process(() -> new Processor<Object, String>() {
@Override
public void init(ProcessorContext processorContext) {
}
@Override
public void process(Object o, String s) {
retryTemplate.execute(context -> {
//Critical business logic goes here.
});
}
@Override
public void close() {
}
});
}
Or you can use a custom RetryTemplate
as below.
@EnableAutoConfiguration
public static class CustomRetryTemplateApp {
@Bean
@StreamRetryTemplate
RetryTemplate fooRetryTemplate() {
RetryTemplate retryTemplate = new RetryTemplate();
RetryPolicy retryPolicy = new SimpleRetryPolicy(4);
FixedBackOffPolicy backOffPolicy = new FixedBackOffPolicy();
backOffPolicy.setBackOffPeriod(1);
retryTemplate.setBackOffPolicy(backOffPolicy);
retryTemplate.setRetryPolicy(retryPolicy);
return retryTemplate;
}
@Bean
public java.util.function.Consumer<KStream<Object, String>> process() {
return input -> input
.process(() -> new Processor<Object, String>() {
@Override
public void init(ProcessorContext processorContext) {
}
@Override
public void process(Object o, String s) {
fooRetryTemplate().execute(context -> {
//Critical business logic goes here.
});
}
@Override
public void close() {
}
});
}
}
Note that when retries are exhausted, by default, the last exception will be thrown, causing the processor to terminate.
If you wish to handle the exception and continue processing, you can add a RecoveryCallback to the execute
method:
Here is an example.
retryTemplate.execute(context -> {
//Critical business logic goes here.
}, context -> {
//Recovery logic goes here.
return null;
));
Refer to the Spring Retry project for more information about the RetryTemplate, retry policies, backoff policies and more.
2.8. State Store
State stores are created automatically by Kafka Streams when the high level DSL is used and appropriate calls are made those trigger a state store.
If you want to materialize an incoming KTable
binding as a named state store, then you can do so by using the following strategy.
Lets say you have the following function.
@Bean
public BiFunction<KStream<String, Long>, KTable<String, String>, KStream<String, Long>> process() {
...
}
Then by setting the following property, the incoming KTable
data will be materialized in to the named state store.
spring.cloud.stream.kafka.streams.bindings.process-in-1.consumer.materializedAs: incoming-store
You can define custom state stores as beans in your application and those will be detected and added to the Kafka Streams builder by the binder. Especially when the processor API is used, you need to register a state store manually. In order to do so, you can create the StateStore as a bean in the application. Here are examples of defining such beans.
@Bean
public StoreBuilder myStore() {
return Stores.keyValueStoreBuilder(
Stores.persistentKeyValueStore("my-store"), Serdes.Long(),
Serdes.Long());
}
@Bean
public StoreBuilder otherStore() {
return Stores.windowStoreBuilder(
Stores.persistentWindowStore("other-store",
1L, 3, 3L, false), Serdes.Long(),
Serdes.Long());
}
These state stores can be then accessed by the applications directly.
During the bootstrap, the above beans will be processed by the binder and passed on to the Streams builder object.
Accessing the state store:
Processor<Object, Product>() {
WindowStore<Object, String> state;
@Override
public void init(ProcessorContext processorContext) {
state = (WindowStore)processorContext.getStateStore("mystate");
}
...
}
This will not work when it comes to registering global state stores.
In order to register a global state store, please see the section below on customizing StreamsBuilderFactoryBean
.
2.9. Interactive Queries
Kafka Streams binder API exposes a class called InteractiveQueryService
to interactively query the state stores.
You can access this as a Spring bean in your application. An easy way to get access to this bean from your application is to autowire
the bean.
@Autowired
private InteractiveQueryService interactiveQueryService;
Once you gain access to this bean, then you can query for the particular state-store that you are interested. See below.
ReadOnlyKeyValueStore<Object, Object> keyValueStore =
interactiveQueryService.getQueryableStoreType("my-store", QueryableStoreTypes.keyValueStore());
During the startup, the above method call to retrieve the store might fail. For e.g it might still be in the middle of initializing the state store. In such cases, it will be useful to retry this operation. Kafka Streams binder provides a simple retry mechanism to accommodate this.
Following are the two properties that you can use to control this retrying.
-
spring.cloud.stream.kafka.streams.binder.stateStoreRetry.maxAttempts - Default is
1
. -
spring.cloud.stream.kafka.streams.binder.stateStoreRetry.backOffInterval - Default is
1000
milliseconds.
If there are multiple instances of the kafka streams application running, then before you can query them interactively, you need to identify which application instance hosts the particular key that you are querying.
InteractiveQueryService
API provides methods for identifying the host information.
In order for this to work, you must configure the property application.server
as below:
spring.cloud.stream.kafka.streams.binder.configuration.application.server: <server>:<port>
Here are some code snippets:
org.apache.kafka.streams.state.HostInfo hostInfo = interactiveQueryService.getHostInfo("store-name",
key, keySerializer);
if (interactiveQueryService.getCurrentHostInfo().equals(hostInfo)) {
//query from the store that is locally available
}
else {
//query from the remote host
}
2.9.1. Other API methods available through the InteractiveQueryService
Use the following API method to retrieve the KeyQueryMetadata
object associated with the combination of given store and key.
public <K> KeyQueryMetadata getKeyQueryMetadata(String store, K key, Serializer<K> serializer)
Use the following API method to retrieve the KakfaStreams
object associated with the combination of given store and key.
public <K> KafkaStreams getKafkaStreams(String store, K key, Serializer<K> serializer)
2.10. Health Indicator
The health indicator requires the dependency spring-boot-starter-actuator
. For maven use:
<dependency>
<groupId>org.springframework.boot</groupId>
<artifactId>spring-boot-starter-actuator</artifactId>
</dependency>
Spring Cloud Stream Kafka Streams Binder provides a health indicator to check the state of the underlying streams threads.
Spring Cloud Stream defines a property management.health.binders.enabled
to enable the health indicator. See the
Spring Cloud Stream documentation.
The health indicator provides the following details for each stream thread’s metadata:
-
Thread name
-
Thread state:
CREATED
,RUNNING
,PARTITIONS_REVOKED
,PARTITIONS_ASSIGNED
,PENDING_SHUTDOWN
orDEAD
-
Active tasks: task ID and partitions
-
Standby tasks: task ID and partitions
By default, only the global status is visible (UP
or DOWN
). To show the details, the property management.endpoint.health.show-details
must be set to ALWAYS
or WHEN_AUTHORIZED
.
For more details about the health information, see the
Spring Boot Actuator documentation.
The status of the health indicator is UP if all the Kafka threads registered are in the RUNNING state.
|
Since there are three individual binders in Kafka Streams binder (KStream
, KTable
and GlobalKTable
), all of them will report the health status.
When enabling show-details
, some of the information reported may be redundant.
When there are multiple Kafka Streams processors present in the same application, then the health checks will be reported for all of them and will be categorized by the application ID of Kafka Streams.
2.11. Accessing Kafka Streams Metrics
Spring Cloud Stream Kafka Streams binder provides Kafka Streams metrics which can be exported through a Micrometer MeterRegistry
.
For Spring Boot version 2.2.x, the metrics support is provided through a custom Micrometer metrics implementation by the binder. For Spring Boot version 2.3.x, the Kafka Streams metrics support is provided natively through Micrometer.
When accessing metrics through the Boot actuator endpoint, make sure to add metrics
to the property management.endpoints.web.exposure.include
.
Then you can access /acutator/metrics
to get a list of all the available metrics, which then can be individually accessed through the same URI (/actuator/metrics/<metric-name>
).
2.12. Mixing high level DSL and low level Processor API
Kafka Streams provides two variants of APIs.
It has a higher level DSL like API where you can chain various operations that maybe familiar to a lot of functional programmers.
Kafka Streams also gives access to a low level Processor API.
The processor API, although very powerful and gives the ability to control things in a much lower level, is imperative in nature.
Kafka Streams binder for Spring Cloud Stream, allows you to use either the high level DSL or mixing both the DSL and the processor API.
Mixing both of these variants give you a lot of options to control various use cases in an application.
Applications can use the transform
or process
method API calls to get access to the processor API.
Here is a look at how one may combine both the DSL and the processor API in a Spring Cloud Stream application using the process
API.
@Bean
public Consumer<KStream<Object, String>> process() {
return input ->
input.process(() -> new Processor<Object, String>() {
@Override
@SuppressWarnings("unchecked")
public void init(ProcessorContext context) {
this.context = context;
}
@Override
public void process(Object key, String value) {
//business logic
}
@Override
public void close() {
});
}
Here is an example using the transform
API.
@Bean
public Consumer<KStream<Object, String>> process() {
return (input, a) ->
input.transform(() -> new Transformer<Object, String, KeyValue<Object, String>>() {
@Override
public void init(ProcessorContext context) {
}
@Override
public void close() {
}
@Override
public KeyValue<Object, String> transform(Object key, String value) {
// business logic - return transformed KStream;
}
});
}
The process
API method call is a terminal operation while the transform
API is non terminal and gives you a potentially transformed KStream
using which you can continue further processing using either the DSL or the processor API.
2.13. Partition support on the outbound
A Kafka Streams processor usually sends the processed output into an outbound Kafka topic.
If the outbound topic is partitioned and the processor needs to send the outgoing data into particular partitions, the applications needs to provide a bean of type StreamPartitioner
.
See StreamPartitioner for more details.
Let’s see some examples.
This is the same processor we already saw multiple times,
@Bean
public Function<KStream<Object, String>, KStream<?, WordCount>> process() {
...
}
Here is the output binding destination:
spring.cloud.stream.bindings.process-out-0.destination: outputTopic
If the topic outputTopic
has 4 partitions, if you don’t provide a partitioning strategy, Kafka Streams will use default partitioning strategy which may not be the outcome you want depending on the particular use case.
Let’s say, you want to send any key that matches to spring
to partition 0, cloud
to partition 1, stream
to partition 2, and everything else to partition 3.
This is what you need to do in the application.
@Bean
public StreamPartitioner<String, WordCount> streamPartitioner() {
return (t, k, v, n) -> {
if (k.equals("spring")) {
return 0;
}
else if (k.equals("cloud")) {
return 1;
}
else if (k.equals("stream")) {
return 2;
}
else {
return 3;
}
};
}
This is a rudimentary implementation, however, you have access to the key/value of the record, the topic name and the total number of partitions. Therefore, you can implement complex partitioning strategies if need be.
You also need to provide this bean name along with the application configuration.
spring.cloud.stream.kafka.streams.bindings.process-out-0.producer.streamPartitionerBeanName: streamPartitioner
Each output topic in the application needs to be configured separately like this.
2.14. StreamsBuilderFactoryBean customizer
It is often required to customize the StreamsBuilderFactoryBean
that creates the KafkaStreams
objects.
Based on the underlying support provided by Spring Kafka, the binder allows you to customize the StreamsBuilderFactoryBean
.
You can use the StreamsBuilderFactoryBeanCustomizer
to customize the StreamsBuilderFactoryBean
itself.
Then, once you get access to the StreamsBuilderFactoryBean
through this customizer, you can customize the corresponding KafkaStreams
using KafkaStreamsCustomzier
.
Both of these customizers are part of the Spring for Apache Kafka project.
Here is an example of using the StreamsBuilderFactoryBeanCustomizer
.
@Bean
public StreamsBuilderFactoryBeanCustomizer streamsBuilderFactoryBeanCustomizer() {
return sfb -> sfb.setStateListener((newState, oldState) -> {
//Do some action here!
});
}
The above is shown as an illustration of the things you can do to customize the StreamsBuilderFactoryBean
.
You can essentially call any available mutation operations from StreamsBuilderFactoryBean
to customize it.
This customizer will be invoked by the binder right before the factory bean is started.
Once you get access to the StreamsBuilderFactoryBean
, you can also customize the underlying KafkaStreams
object.
Here is a blueprint for doing so.
@Bean
public StreamsBuilderFactoryBeanCustomizer streamsBuilderFactoryBeanCustomizer() {
return factoryBean -> {
factoryBean.setKafkaStreamsCustomizer(new KafkaStreamsCustomizer() {
@Override
public void customize(KafkaStreams kafkaStreams) {
kafkaStreams.setUncaughtExceptionHandler((t, e) -> {
});
}
});
};
}
KafkaStreamsCustomizer
will be called by the StreamsBuilderFactoryBeabn
right before the underlying KafkaStreams
gets started.
There can only be one StreamsBuilderFactoryBeanCustomizer
in the entire application.
Then how do we account for multiple Kafka Streams processors as each of them are backed up by individual StreamsBuilderFactoryBean
objects?
In that case, if the customization needs to be different for those processors, then the application needs to apply some filter based on the application ID.
For e.g,
@Bean
public StreamsBuilderFactoryBeanCustomizer streamsBuilderFactoryBeanCustomizer() {
return factoryBean -> {
if (factoryBean.getStreamsConfiguration().getProperty(StreamsConfig.APPLICATION_ID_CONFIG)
.equals("processor1-application-id")) {
factoryBean.setKafkaStreamsCustomizer(new KafkaStreamsCustomizer() {
@Override
public void customize(KafkaStreams kafkaStreams) {
kafkaStreams.setUncaughtExceptionHandler((t, e) -> {
});
}
});
}
};
2.14.1. Using Customizer to register a global state store
As mentioned above, the binder does not provide a first class way to register global state stores as a feature. For that, you need to use the customizer. Here is how that can be done.
@Bean
public StreamsBuilderFactoryBeanCustomizer customizer() {
return fb -> {
try {
final StreamsBuilder streamsBuilder = fb.getObject();
streamsBuilder.addGlobalStore(...);
}
catch (Exception e) {
}
};
}
Again, if you have multiple processors, you want to attach the global state store to the right StreamsBuilder
by filtering out the other StreamsBuilderFactoryBean
objects using the application id as outlined above.
2.14.2. Using customizer to register a production exception handler
In the error handling section, we indicated that the binder does not provide a first class way to deal with production exceptions.
Though that is the case, you can still use the StreamsBuilderFacotryBean
customizer to register production exception handlers. See below.
@Bean
public StreamsBuilderFactoryBeanCustomizer customizer() {
return fb -> {
fb.getStreamsConfiguration().put(StreamsConfig.DEFAULT_PRODUCTION_EXCEPTION_HANDLER_CLASS_CONFIG,
CustomProductionExceptionHandler.class);
};
}
Once again, if you have multiple processors, you may want to set it appropriately against the correct StreamsBuilderFactoryBean
.
You may also add such production exception handlers using the configuration property (See below for more on that), but this is an option if you choose to go with a programmatic approach.
2.15. Timestamp extractor
Kafka Streams allows you to control the processing of the consumer records based on various notions of timestamp.
By default, Kafka Streams extracts the timestamp metadata embedded in the consumer record.
You can change this default behavior by providing a different TimestampExtractor
implementation per input binding.
Here are some details on how that can be done.
@Bean
public Function<KStream<Long, Order>,
Function<KTable<Long, Customer>,
Function<GlobalKTable<Long, Product>, KStream<Long, Order>>>> process() {
return orderStream ->
customers ->
products -> orderStream;
}
@Bean
public TimestampExtractor timestampExtractor() {
return new WallclockTimestampExtractor();
}
Then you set the above TimestampExtractor
bean name per consumer binding.
spring.cloud.stream.kafka.streams.bindings.process-in-0.consumer.timestampExtractorBeanName=timestampExtractor
spring.cloud.stream.kafka.streams.bindings.process-in-1.consumer.timestampExtractorBeanName=timestampExtractor
spring.cloud.stream.kafka.streams.bindings.process-in-2.consumer.timestampExtractorBeanName=timestampExtractor"
If you skip an input consumer binding for setting a custom timestamp extractor, that consumer will use the default settings.
2.16. Multi binders with Kafka Streams based binders and regular Kafka Binder
You can have an application where you have both a function/consumer/supplier that is based on the regular Kafka binder and a Kafka Streams based processor. However, you cannot mix both of them within a single function or consumer.
Here is an example, where you have both binder based components within the same application.
@Bean
public Function<String, String> process() {
return s -> s;
}
@Bean
public Function<KStream<Object, String>, KStream<?, WordCount>> kstreamProcess() {
return input -> input;
}
This is the relevant parts from the configuration:
spring.cloud.stream.function.definition=process;kstreamProcess
spring.cloud.stream.bindings.process-in-0.destination=foo
spring.cloud.stream.bindings.process-out-0.destination=bar
spring.cloud.stream.bindings.kstreamProcess-in-0.destination=bar
spring.cloud.stream.bindings.kstreamProcess-out-0.destination=foobar
Things become a bit more complex if you have the same application as above, but is dealing with two different Kafka clusters, for e.g. the regular process
is acting upon both Kafka cluster 1 and cluster 2 (receiving data from cluster-1 and sending to cluster-2) and the Kafka Streams processor is acting upon Kafka cluster 2.
Then you have to use the multi binder facilities provided by Spring Cloud Stream.
Here is how your configuration may change in that scenario.
# multi binder configuration
spring.cloud.stream.binders.kafka1.type: kafka
spring.cloud.stream.binders.kafka1.environment.spring.cloud.stream.kafka.streams.binder.brokers=${kafkaCluster-1} #Replace kafkaCluster-1 with the approprate IP of the cluster
spring.cloud.stream.binders.kafka2.type: kafka
spring.cloud.stream.binders.kafka2.environment.spring.cloud.stream.kafka.streams.binder.brokers=${kafkaCluster-2} #Replace kafkaCluster-2 with the approprate IP of the cluster
spring.cloud.stream.binders.kafka3.type: kstream
spring.cloud.stream.binders.kafka3.environment.spring.cloud.stream.kafka.streams.binder.brokers=${kafkaCluster-2} #Replace kafkaCluster-2 with the approprate IP of the cluster
spring.cloud.stream.function.definition=process;kstreamProcess
# From cluster 1 to cluster 2 with regular process function
spring.cloud.stream.bindings.process-in-0.destination=foo
spring.cloud.stream.bindings.process-in-0.binder=kafka1 # source from cluster 1
spring.cloud.stream.bindings.process-out-0.destination=bar
spring.cloud.stream.bindings.process-out-0.binder=kafka2 # send to cluster 2
# Kafka Streams processor on cluster 2
spring.cloud.stream.bindings.kstreamProcess-in-0.destination=bar
spring.cloud.stream.bindings.kstreamProcess-in-0.binder=kafka3
spring.cloud.stream.bindings.kstreamProcess-out-0.destination=foobar
spring.cloud.stream.bindings.kstreamProcess-out-0.binder=kafka3
Pay attention to the above configuration.
We have two kinds of binders, but 3 binders all in all, first one is the regular Kafka binder based on cluster 1 (kafka1
), then another Kafka binder based on cluster 2 (kafka2
) and finally the kstream
one (kafka3
).
The first processor in the application receives data from kafka1
and publishes to kafka2
where both binders are based on regular Kafka binder but differnt clusters.
The second processor, which is a Kafka Streams processor consumes data from kafka3
which is the same cluster as kafka2
, but a different binder type.
Since there are three different binder types available in the Kafka Streams family of binders - kstream
, ktable
and globalktable
- if your application has multiple bindings based on any of these binders, that needs to be explicitly provided as the binder type.
For e.g if you have a processor as below,
@Bean
public Function<KStream<Long, Order>,
Function<KTable<Long, Customer>,
Function<GlobalKTable<Long, Product>, KStream<Long, EnrichedOrder>>>> enrichOrder() {
...
}
then, this has to be configured in a multi binder scenario as the following. Please note that this is only needed if you have a true multi-binder scenario where there are multiple processors dealing with multiple clusters within a single application. In that case, the binders need to be explicitly provided with the bindings to distinguish from other processor’s binder types and clusters.
spring.cloud.stream.binders.kafka1.type: kstream
spring.cloud.stream.binders.kafka1.environment.spring.cloud.stream.kafka.streams.binder.brokers=${kafkaCluster-2}
spring.cloud.stream.binders.kafka2.type: ktable
spring.cloud.stream.binders.kafka2.environment.spring.cloud.stream.kafka.streams.binder.brokers=${kafkaCluster-2}
spring.cloud.stream.binders.kafka3.type: globalktable
spring.cloud.stream.binders.kafka3.environment.spring.cloud.stream.kafka.streams.binder.brokers=${kafkaCluster-2}
spring.cloud.stream.bindings.enrichOrder-in-0.binder=kafka1 #kstream
spring.cloud.stream.bindings.enrichOrder-in-1.binder=kafka2 #ktablr
spring.cloud.stream.bindings.enrichOrder-in-2.binder=kafka3 #globalktable
spring.cloud.stream.bindings.enrichOrder-out-0.binder=kafka1 #kstream
# rest of the configuration is omitted.
2.17. State Cleanup
By default, the Kafkastreams.cleanup()
method is called when the binding is stopped.
See the Spring Kafka documentation.
To modify this behavior simply add a single CleanupConfig
@Bean
(configured to clean up on start, stop, or neither) to the application context; the bean will be detected and wired into the factory bean.
2.18. Kafka Streams topology visualization
Kafka Streams binder provides the following actuator endpoints for retrieving the topology description using which you can visualize the topology using external tools.
/actuator/kafkastreamstopology
/actuator/kafkastreamstopology/<application-id of the processor>
You need to include the actuator and web dependencies from Spring Boot to access these endpoints.
Further, you also need to add kafkastreamstopology
to management.endpoints.web.exposure.include
property.
By default, the kafkastreamstopology
endpoint is disabled.
2.19. Event type based routing in Kafka Streams applications
Routing functions available in regular message channel based binders are not supported in Kafka Streams binder. However, Kafka Streams binder still provides routing capabilities through the event type record header on the inbound records.
To enable routing based on event types, the application must provide the following property.
spring.cloud.stream.kafka.streams.bindings.<binding-name>.consumer.eventTypes
.
This can be a comma separated value.
For example, lets assume we have this function:
@Bean
public Function<KStream<Integer, Foo>, KStream<Integer, Foo>> process() {
return input -> input;
}
Let us also assume that we only want the business logic in this function to be executed, if the incoming record has event types as foo
or bar
.
That can be expressed as below using the eventTypes
property on the binding.
spring.cloud.stream.kafka.streams.bindings.process-in-0.consumer.eventTypes=foo,bar
Now, when the application runs, the binder checks each incoming records for the header event_type
and see if it has value set as foo
or bar
.
If it does not find either of them, then the function execution will be skipped.
By default, the binder expects the record header key to be event_type
, but that can be changed per binding.
For instance, if we want to change the header key on this binding to my_event
instead of the default, that can be changed as below.
spring.cloud.stream.kafka.streams.bindings.process-in-0.consumer.eventTypeHeaderKey=my_event
.
2.20. Binding visualization and control in Kafka Streams binder
Starting with version 3.1.2, Kafka Streams binder supports binding visualization and control.
The only two lifecycle phases supported are STOPPED
and STARTED
.
The lifecycle phases PAUSED
and RESUMED
are not available in Kafka Streams binder.
In order to activate binding visualization and control, the application needs to include the following two dependencies.
<dependency>
<groupId>org.springframework.boot</groupId>
<artifactId>spring-boot-starter-actuator</artifactId>
</dependency>
<dependency>
<groupId>org.springframework.boot</groupId>
<artifactId>spring-boot-starter-web</artifactId>
</dependency>
If you prefer using webflux, you can then include spring-boot-starter-webflux
instead of the standard web dependency.
In addition, you also need to set the following property:
management.endpoints.web.exposure.include=bindings
To illustrate this feature further, let us use the following application as a guide:
@SpringBootApplication
public class KafkaStreamsApplication {
public static void main(String[] args) {
SpringApplication.run(KafkaStreamsApplication.class, args);
}
@Bean
public Consumer<KStream<String, String>> consumer() {
return s -> s.foreach((key, value) -> System.out.println(value));
}
@Bean
public Function<KStream<String, String>, KStream<String, String>> function() {
return ks -> ks;
}
}
As we can see, the application has two Kafka Streams functions - one, a consumer and another a function.
The consumer binding is named by default as consumer-in-0
.
Similarly, for the function, the input binding is function-in-0
and the output binding is function-out-0
.
Once the application is started, we can find details about the bindings using the following bindings endpoint.
curl http://localhost:8080/actuator/bindings | jq .
[
{
"bindingName": "consumer-in-0",
"name": "consumer-in-0",
"group": "consumer-applicationId",
"pausable": false,
"state": "running",
"paused": false,
"input": true,
"extendedInfo": {}
},
{
"bindingName": "function-in-0",
"name": "function-in-0",
"group": "function-applicationId",
"pausable": false,
"state": "running",
"paused": false,
"input": true,
"extendedInfo": {}
},
{
"bindingName": "function-out-0",
"name": "function-out-0",
"group": "function-applicationId",
"pausable": false,
"state": "running",
"paused": false,
"input": false,
"extendedInfo": {}
}
]
The details about all three bindings can be found above.
Let us now stop the consumer-in-0 binding.
curl -d '{"state":"STOPPED"}' -H "Content-Type: application/json" -X POST http://localhost:8080/actuator/bindings/consumer-in-0
At this point, no records will be received through this binding.
Start the binding again.
curl -d '{"state":"STARTED"}' -H "Content-Type: application/json" -X POST http://localhost:8080/actuator/bindings/consumer-in-0
When there are multiple bindings present on a single function, invoking these operations on any of those bindings will work.
This is because all the bindings on a single function are backed by the same StreamsBuilderFactoryBean
.
Therefore, for the function above, either function-in-0
or function-out-0
will work.
2.21. Tracing using Spring Cloud Sleuth
When Spring Cloud Sleuth is on the classpath of a Spring Cloud Stream Kafka Streams binder based application, both its consumer and producer are automatically instrumented with tracing information.
However, in order to trace any application specific operations, those need to be explicitly instrumented by the user code.
This can be done by injecting the KafkaStreamsTracing
bean from Spring Cloud Sleuth in the application and then invoke various Kafka Streams operations through this injected bean.
Here are some examples of using it.
@Bean
public BiFunction<KStream<String, Long>, KTable<String, String>, KStream<String, Long>> clicks(KafkaStreamsTracing kafkaStreamsTracing) {
return (userClicksStream, userRegionsTable) -> (userClicksStream
.transformValues(kafkaStreamsTracing.peek("span-1", (key, value) -> LOG.info("key/value: " + key + "/" + value)))
.leftJoin(userRegionsTable, (clicks, region) -> new RegionWithClicks(region == null ?
"UNKNOWN" : region, clicks),
Joined.with(Serdes.String(), Serdes.Long(), null))
.transform(kafkaStreamsTracing.map("span-2", (key, value) -> {
LOG.info("Click Info: " + value.getRegion() + "/" + value.getClicks());
return new KeyValue<>(value.getRegion(),
value.getClicks());
}))
.groupByKey(Grouped.with(Serdes.String(), Serdes.Long()))
.reduce(Long::sum, Materialized.as(CLICK_UPDATES))
.toStream());
}
In the example above, there are two places where it adds explicit tracing instrumentation.
First, we are logging the key/value information from the incoming KStream
.
When this information is logged, the associated span and trace IDs get logged as well so that a monitoring system can track them and correlate with the same span id.
Second, when we call a map
operation, instead of calling it directly on the KStream
class, we wrap it inside a transform
operation and then call map
from KafkaStreamsTracing
.
In this case also, the logged message will contain the span ID and trace ID.
Here is another example, where we use the low-level transformer API for accessing the various Kafka Streams headers. When spring-cloud-sleuth is on the classpath, all the tracing headers can also be accessed like this.
@Bean
public Function<KStream<String, String>, KStream<String, String>> process(KafkaStreamsTracing kafkaStreamsTracing) {
return input -> input.transform(kafkaStreamsTracing.transformer(
"transformer-1",
() -> new Transformer<String, String, KeyValue<String, String>>() {
ProcessorContext context;
@Override
public void init(ProcessorContext context) {
this.context = context;
}
@Override
public KeyValue<String, String> transform(String key, String value) {
LOG.info("Headers: " + this.context.headers());
LOG.info("K/V:" + key + "/" + value);
// More transformations, business logic execution, etc. go here.
return KeyValue.pair(key, value);
}
@Override
public void close() {
}
}));
}
2.22. Configuration Options
This section contains the configuration options used by the Kafka Streams binder.
For common configuration options and properties pertaining to binder, refer to the core documentation.
2.22.1. Kafka Streams Binder Properties
The following properties are available at the binder level and must be prefixed with spring.cloud.stream.kafka.streams.binder.
Any Kafka binder provided properties re-used in Kafka Streams binder must be prefixed with spring.cloud.stream.kafka.streams.binder
instead of spring.cloud.stream.kafka.binder
.
The only exception to this rule is when defining the Kafka bootstrap server property in which case either prefix works.
- configuration
-
Map with a key/value pair containing properties pertaining to Apache Kafka Streams API. This property must be prefixed with
spring.cloud.stream.kafka.streams.binder.
. Following are some examples of using this property.
spring.cloud.stream.kafka.streams.binder.configuration.default.key.serde=org.apache.kafka.common.serialization.Serdes$StringSerde
spring.cloud.stream.kafka.streams.binder.configuration.default.value.serde=org.apache.kafka.common.serialization.Serdes$StringSerde
spring.cloud.stream.kafka.streams.binder.configuration.commit.interval.ms=1000
For more information about all the properties that may go into streams configuration, see StreamsConfig
JavaDocs in Apache Kafka Streams docs.
All configuration that you can set from StreamsConfig
can be set through this.
When using this property, it is applicable against the entire application since this is a binder level property.
If you have more than processors in the application, all of them will acquire these properties.
In the case of properties like application.id
, this will become problematic and therefore you have to carefully examine how the properties from StreamsConfig
are mapped using this binder level configuration
property.
- functions.<function-bean-name>.applicationId
-
Applicable only for functional style processors. This can be used for setting application ID per function in the application. In the case of multiple functions, this is a handy way to set the application ID.
- functions.<function-bean-name>.configuration
-
Applicable only for functional style processors. Map with a key/value pair containing properties pertaining to Apache Kafka Streams API. This is similar to the binder level
configuration
property describe above, but this level ofconfiguration
property is restricted only against the named function. When you have multiple processors and you want to restrict access to the configuration based on particular functions, you might want to use this. AllStreamsConfig
properties can be used here. - brokers
-
Broker URL
Default:
localhost
- zkNodes
-
Zookeeper URL
Default:
localhost
- deserializationExceptionHandler
-
Deserialization error handler type. This handler is applied at the binder level and thus applied against all input binding in the application. There is a way to control it in a more fine-grained way at the consumer binding level. Possible values are -
logAndContinue
,logAndFail
,skipAndContinue
orsendToDlq
Default:
logAndFail
- applicationId
-
Convenient way to set the application.id for the Kafka Streams application globally at the binder level. If the application contains multiple functions or
StreamListener
methods, then the application id should be set differently. See above where setting the application id is discussed in detail.Default: application will generate a static application ID. See the application ID section for more details.
- stateStoreRetry.maxAttempts
-
Max attempts for trying to connect to a state store.
Default: 1
- stateStoreRetry.backoffPeriod
-
Backoff period when trying to connect to a state store on a retry.
Default: 1000 ms
- consumerProperties
-
Arbitrary consumer properties at the binder level.
- producerProperties
-
Arbitrary producer properties at the binder level.
2.22.2. Kafka Streams Producer Properties
The following properties are only available for Kafka Streams producers and must be prefixed with spring.cloud.stream.kafka.streams.bindings.<binding name>.producer.
For convenience, if there are multiple output bindings and they all require a common value, that can be configured by using the prefix spring.cloud.stream.kafka.streams.default.producer.
.
- keySerde
-
key serde to use
Default: See the above discussion on message de/serialization
- valueSerde
-
value serde to use
Default: See the above discussion on message de/serialization
- useNativeEncoding
-
flag to enable/disable native encoding
Default:
true
. - streamPartitionerBeanName
-
Custom outbound partitioner bean name to be used at the consumer. Applications can provide custom
StreamPartitioner
as a Spring bean and the name of this bean can be provided to the producer to use instead of the default one.Default: See the discussion above on outbound partition support.
- producedAs
-
Custom name for the sink component to which the processor is producing to.
Deafult:
none
(generated by Kafka Streams)
2.22.3. Kafka Streams Consumer Properties
The following properties are available for Kafka Streams consumers and must be prefixed with spring.cloud.stream.kafka.streams.bindings.<binding-name>.consumer.
For convenience, if there are multiple input bindings and they all require a common value, that can be configured by using the prefix spring.cloud.stream.kafka.streams.default.consumer.
.
- applicationId
-
Setting application.id per input binding. This is only preferred for
StreamListener
based processors, for function based processors see other approaches outlined above.Default: See above.
- keySerde
-
key serde to use
Default: See the above discussion on message de/serialization
- valueSerde
-
value serde to use
Default: See the above discussion on message de/serialization
- materializedAs
-
state store to materialize when using incoming KTable types
Default:
none
. - useNativeDecoding
-
flag to enable/disable native decoding
Default:
true
. - dlqName
-
DLQ topic name.
Default: See above on the discussion of error handling and DLQ.
- startOffset
-
Offset to start from if there is no committed offset to consume from. This is mostly used when the consumer is consuming from a topic for the first time. Kafka Streams uses
earliest
as the default strategy and the binder uses the same default. This can be overridden tolatest
using this property.Default:
earliest
.
Note: Using resetOffsets
on the consumer does not have any effect on Kafka Streams binder.
Unlike the message channel based binder, Kafka Streams binder does not seek to beginning or end on demand.
- deserializationExceptionHandler
-
Deserialization error handler type. This handler is applied per consumer binding as opposed to the binder level property described before. Possible values are -
logAndContinue
,logAndFail
,skipAndContinue
orsendToDlq
Default:
logAndFail
- timestampExtractorBeanName
-
Specific time stamp extractor bean name to be used at the consumer. Applications can provide
TimestampExtractor
as a Spring bean and the name of this bean can be provided to the consumer to use instead of the default one.Default: See the discussion above on timestamp extractors.
- eventTypes
-
Comma separated list of supported event types for this binding.
Default:
none
- eventTypeHeaderKey
-
Event type header key on each incoming records through this binding.
Default:
event_type
- consumedAs
-
Custom name for the source component from which the processor is consuming from.
Deafult:
none
(generated by Kafka Streams)
2.22.4. Special note on concurrency
In Kafka Streams, you can control of the number of threads a processor can create using the num.stream.threads
property.
This, you can do using the various configuration
options described above under binder, functions, producer or consumer level.
You can also use the concurrency
property that core Spring Cloud Stream provides for this purpose.
When using this, you need to use it on the consumer.
When you have more than one input bindings either in a function or StreamListener
, set this on the first input binding.
For e.g. when setting spring.cloud.stream.bindings.process-in-0.consumer.concurrency
, it will be translated as num.stream.threads
by the binder.
If you have multiple processors and one processor defines binding level concurrency, but not the others, those ones with no binding level concurrency will default back to the binder wide property specified through
spring.cloud.stream.kafka.streams.binder.configuration.num.stream.threads
.
If this binder configuration is not available, then the application will use the default set by Kafka Streams.
Appendices
Appendix A: Building
A.1. Basic Compile and Test
To build the source you will need to install JDK 1.7.
The build uses the Maven wrapper so you don’t have to install a specific version of Maven. To enable the tests, you should have Kafka server 0.9 or above running before building. See below for more information on running the servers.
The main build command is
$ ./mvnw clean install
You can also add '-DskipTests' if you like, to avoid running the tests.
You can also install Maven (>=3.3.3) yourself and run the mvn command
in place of ./mvnw in the examples below. If you do that you also
might need to add -P spring if your local Maven settings do not
contain repository declarations for spring pre-release artifacts.
|
Be aware that you might need to increase the amount of memory
available to Maven by setting a MAVEN_OPTS environment variable with
a value like -Xmx512m -XX:MaxPermSize=128m . We try to cover this in
the .mvn configuration, so if you find you have to do it to make a
build succeed, please raise a ticket to get the settings added to
source control.
|
The projects that require middleware generally include a
docker-compose.yml
, so consider using
Docker Compose to run the middeware servers
in Docker containers.
A.2. Documentation
There is a "full" profile that will generate documentation.
A.3. Working with the code
If you don’t have an IDE preference we would recommend that you use Spring Tools Suite or Eclipse when working with the code. We use the m2eclipe eclipse plugin for maven support. Other IDEs and tools should also work without issue.
A.3.1. Importing into eclipse with m2eclipse
We recommend the m2eclipe eclipse plugin when working with eclipse. If you don’t already have m2eclipse installed it is available from the "eclipse marketplace".
Unfortunately m2e does not yet support Maven 3.3, so once the projects
are imported into Eclipse you will also need to tell m2eclipse to use
the .settings.xml
file for the projects. If you do not do this you
may see many different errors related to the POMs in the
projects. Open your Eclipse preferences, expand the Maven
preferences, and select User Settings. In the User Settings field
click Browse and navigate to the Spring Cloud project you imported
selecting the .settings.xml
file in that project. Click Apply and
then OK to save the preference changes.
Alternatively you can copy the repository settings from .settings.xml into your own ~/.m2/settings.xml .
|
A.3.2. Importing into eclipse without m2eclipse
If you prefer not to use m2eclipse you can generate eclipse project metadata using the following command:
$ ./mvnw eclipse:eclipse
The generated eclipse projects can be imported by selecting import existing projects
from the file
menu.
[[contributing] == Contributing
Spring Cloud is released under the non-restrictive Apache 2.0 license, and follows a very standard Github development process, using Github tracker for issues and merging pull requests into master. If you want to contribute even something trivial please do not hesitate, but follow the guidelines below.
A.4. Sign the Contributor License Agreement
Before we accept a non-trivial patch or pull request we will need you to sign the contributor’s agreement. Signing the contributor’s agreement does not grant anyone commit rights to the main repository, but it does mean that we can accept your contributions, and you will get an author credit if we do. Active contributors might be asked to join the core team, and given the ability to merge pull requests.
A.5. Code Conventions and Housekeeping
None of these is essential for a pull request, but they will all help. They can also be added after the original pull request but before a merge.
-
Use the Spring Framework code format conventions. If you use Eclipse you can import formatter settings using the
eclipse-code-formatter.xml
file from the Spring Cloud Build project. If using IntelliJ, you can use the Eclipse Code Formatter Plugin to import the same file. -
Make sure all new
.java
files to have a simple Javadoc class comment with at least an@author
tag identifying you, and preferably at least a paragraph on what the class is for. -
Add the ASF license header comment to all new
.java
files (copy from existing files in the project) -
Add yourself as an
@author
to the .java files that you modify substantially (more than cosmetic changes). -
Add some Javadocs and, if you change the namespace, some XSD doc elements.
-
A few unit tests would help a lot as well — someone has to do it.
-
If no-one else is using your branch, please rebase it against the current master (or other target branch in the main project).
-
When writing a commit message please follow these conventions, if you are fixing an existing issue please add
Fixes gh-XXXX
at the end of the commit message (where XXXX is the issue number).