Version 1.2.0.RC2
© 2012-2017 Pivotal Software, Inc.
Copies of this document may be made for your own use and for distribution to others, provided that you do not charge any fee for such copies and further provided that each copy contains this Copyright Notice, whether distributed in print or electronically.
Preface
1. About the documentation
The Spring Cloud Data Flow reference guide is available as html, pdf and epub documents. The latest copy is available at docs.spring.io/spring-cloud-dataflow/docs/current-SNAPSHOT/reference/html/.
Copies of this document may be made for your own use and for distribution to others, provided that you do not charge any fee for such copies and further provided that each copy contains this Copyright Notice, whether distributed in print or electronically.
2. Getting help
Having trouble with Spring Cloud Data Flow, We’d like to help!
-
Ask a question - we monitor stackoverflow.com for questions tagged with
spring-cloud-dataflow
. -
Report bugs with Spring Cloud Data Flow at github.com/spring-cloud/spring-cloud-dataflow/issues.
All of Spring Cloud Data Flow is open source, including the documentation! If you find problems with the docs; or if you just want to improve them, please get involved. |
Spring Cloud Data Flow Overview
This section provides a brief overview of the Spring Cloud Data Flow reference documentation. Think of it as map for the rest of the document. You can read this reference guide in a linear fashion, or you can skip sections if something doesn’t interest you.
3. Introducing Spring Cloud Data Flow
Spring Cloud Data Flow is a cloud-native orchestration service for composable microservice applications on modern runtimes. With Spring Cloud Data Flow, developers can create and orchestrate data pipelines for common use cases such as data ingest, real-time analytics, and data import/export.
Spring Cloud Data Flow is the cloud native redesign of Spring XD – a project that aimed to simplify development of Big Data applications. The stream and batch modules from Spring XD are refactored as Spring Boot based stream and task/batch microservice applications respectively. These applications are now autonomous deployment units and they can "natively" run in modern runtimes such as Cloud Foundry, Apache YARN, Apache Mesos, and Kubernetes.
Spring Cloud Data Flow offers a collection of patterns and best practices for microservices-based distributed streaming and task/batch data pipelines.
3.1. Features
-
Develop using DSL, REST-APIs, Dashboard, and the drag-and-drop GUI - Flo
-
Create, unit-test, troubleshoot and manage microservice applications in isolation
-
Build data pipelines rapidly using the out-of-the-box stream and task/batch applications
-
Consume microservice applications as maven or docker artifacts
-
Scale data pipelines without interrupting data flows
-
Orchestrate data-centric applications on a variety of modern runtime platforms including Cloud Foundry, Apache YARN, Apache Mesos, and Kubernetes
-
Take advantage of metrics, health checks, and the remote management of each microservice application
Architecture
4. Introduction
Spring Cloud Data Flow simplifies the development and deployment of applications focused on data processing use-cases. The major concepts of the architecture are Applications, the Data Flow Server, and the target runtime.
Applications come in two flavors
-
Long lived Stream applications where an unbounded amount of data is consumed or produced via messaging middleware.
-
Short lived Task applications that process a finite set of data and then terminate.
Depending on the runtime, applications can be packaged in two ways
-
Spring Boot uber-jar that is hosted in a maven repository, file, http or any other Spring resource implementation.
-
Docker
The runtime is the place where applications execute. The target runtimes for applications are platforms that you may already be using for other application deployments.
The supported runtimes are
-
Cloud Foundry
-
Apache YARN
-
Kubernetes
-
Apache Mesos
-
Local Server for development
There is a deployer Service Provider Interface (SPI) that enables you to extend Data Flow to deploy onto other runtimes, for example to support Docker Swarm. There are community implementations of Hashicorp’s Nomad and RedHat Openshift is available. We look forward to working with the community for further contributions!
The component that is responsible for deploying applications to a runtime is the Data Flow Server. There is a Data Flow Server executable jar provided for each of the target runtimes. The Data Flow server is responsible for interpreting
-
A stream DSL that describes the logical flow of data through multiple applications.
-
A deployment manifest that describes the mapping of applications onto the runtime. For example, to set the initial number of instances, memory requirements, and data partitioning.
As an example, the DSL to describe the flow of data from an http source to an Apache Cassandra sink would be written as “http | cassandra”. These names in the DSL are registered with the Data Flow Server and map onto application artifacts that can be hosted in Maven or Docker repositories. Many source, processor, and sink applications for common use-cases (e.g. jdbc, hdfs, http, router) are provided by the Spring Cloud Data Flow team. The pipe symbol represents the communication between the two applications via messaging middleware. The two messaging middleware brokers that are supported are
-
Apache Kafka
-
RabbitMQ
In the case of Kafka, when deploying the stream, the Data Flow server is responsible to create the topics that correspond to each pipe symbol and configure each application to produce or consume from the topics so the desired flow of data is achieved.
The interaction of the main components is shown below
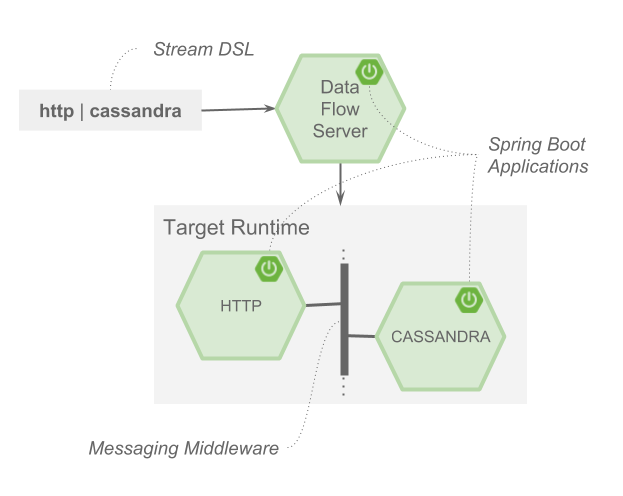
In this diagram a DSL description of a stream is POSTed to the Data Flow Server. Based on the mapping of DSL application names to Maven and Docker artifacts, the http-source and cassandra-sink applications are deployed on the target runtime.
5. Microservice Architectural Style
The Data Flow Server deploys applications onto the target runtime that conform to the microservice architectural style. For example, a stream represents a high level application that consists of multiple small microservice applications each running in their own process. Each microservice application can be scaled up or down independent of the other and each has their own versioning lifecycle.
Both Streaming and Task based microservice applications build upon Spring Boot as the foundational library. This gives all microservice applications functionality such as health checks, security, configurable logging, monitoring and management functionality, as well as executable JAR packaging.
It is important to emphasise that these microservice applications are ‘just apps’ that you can run by yourself using ‘java -jar’ and passing in appropriate configuration properties. We provide many common microservice applications for common operations so you don’t have to start from scratch when addressing common use-cases which build upon the rich ecosystem of Spring Projects, e.g Spring Integration, Spring Data, Spring Hadoop and Spring Batch. Creating your own microservice application is similar to creating other Spring Boot applications, you can start using the Spring Initialzr web site or the UI to create the basic scaffolding of either a Stream or Task based microservice.
In addition to passing in the appropriate configuration to the applications, the Data Flow server is responsible for preparing the target platform’s infrastructure so that the application can be deployed. For example, in Cloud Foundry it would be binding specified services to the applications and executing the ‘cf push’ command for each application. For Kubernetes it would be creating the replication controller, service, and load balancer.
The Data Flow Server helps simplify the deployment of multiple applications onto a target runtime, but one could also opt to deploy each of the microservice applications manually and not use Data Flow at all. This approach might be more appropriate to start out with for small scale deployments, gradually adopting the convenience and consistency of Data Flow as you develop more applications. Manual deployment of Stream and Task based microservices is also a useful educational exercise that will help you better understand some of the automatic applications configuration and platform targeting steps that the Data Flow Server provides.
5.1. Comparison to other Platform architectures
Spring Cloud Data Flow’s architectural style is different than other Stream and Batch processing platforms. For example in Apache Spark, Apache Flink, and Google Cloud Dataflow applications run on a dedicated compute engine cluster. The nature of the compute engine gives these platforms a richer environment for performing complex calculations on the data as compared to Spring Cloud Data Flow, but it introduces complexity of another execution environment that is often not needed when creating data centric applications. That doesn’t mean you cannot do real time data computations when using Spring Cloud Data Flow. Refer to the analytics section which describes the integration of Redis to handle common counting based use-cases as well as the RxJava integration for functional API driven analytics use-cases, such as time-sliding-window and moving-average among others.
Similarly, Apache Storm, Hortonworks DataFlow and Spring Cloud Data Flow’s predecessor, Spring XD, use a dedicated application execution cluster, unique to each product, that determines where your code should execute on the cluster and perform health checks to ensure that long lived applications are restarted if they fail. Often, framework specific interfaces are required to be used in order to correctly “plug in” to the cluster’s execution framework.
As we discovered during the evolution of Spring XD, the rise of multiple container frameworks in 2015 made creating our own runtime a duplication of efforts. There is no reason to build your own resource management mechanics, when there are multiple runtime platforms that offer this functionality already. Taking these considerations into account is what made us shift to the current architecture where we delegate the execution to popular runtimes, runtimes that you may already be using for other purposes. This is an advantage in that it reduces the cognitive distance for creating and managing data centric applications as many of the same skills used for deploying other end-user/web applications are applicable.
6. Streaming Applications
While Spring Boot provides the foundation for creating DevOps friendly microservice applications, other libraries in the Spring ecosystem help create Stream based microservice applications. The most important of these is Spring Cloud Stream.
The essence of the Spring Cloud Stream programming model is to provide an easy way to describe multiple inputs and outputs of an application that communicate over messaging middleware. These input and outputs map onto Kafka topics or Rabbit exchanges and queues. Common application configuration for a Source that generates data, a Process that consumes and produces data and a Sink that consumes data is provided as part of the library.
6.1. Imperative Programming Model
Spring Cloud Stream is most closely integrated with Spring Integration’s imperative "event at a time" programming model. This means you write code that handles a single event callback. For example,
@EnableBinding(Sink.class)
public class LoggingSink {
@StreamListener(Sink.INPUT)
public void log(String message) {
System.out.println(message);
}
}
In this case the String payload of a message coming on the input channel, is handed to the log method. The @EnableBinding
annotation is what is used to tie together the input channel to the external middleware.
6.2. Functional Programming Model
However, Spring Cloud Stream can support other programming styles. The use of reactive APIs where incoming and outgoing data is handled as continuous data flows and it defines how each individual message should be handled. You can also use operators that describe functional transformations from inbound to outbound data flows. The upcoming versions will support Apache Kafka’s KStream API in the programming model.
7. Streams
7.1. Topologies
The Stream DSL describes linear sequences of data flowing through the system. For example, in the stream definition http | transformer | cassandra
, each pipe symbol connects the application on the left to the one on the right. Named channels can be used for routing and to fan out data to multiple messaging destinations.
Taps can be used to ‘listen in’ to the data that if flowing across any of the pipe symbols. Taps can be used as sources for new streams with an in independent life cycle.
7.2. Concurrency
For an application that will consume events, Spring Cloud stream exposes a concurrency setting that controls the size of a thread pool used for dispatching incoming messages. See the Consumer properties documentation for more information.
7.3. Partitioning
A common pattern in stream processing is to partition the data as it moves from one application to the next. Partitioning is a critical concept in stateful processing, for either performance or consistency reasons, to ensure that all related data is processed together. For example, in a time-windowed average calculation example, it is important that all measurements from any given sensor are processed by the same application instance. Alternatively, you may want to cache some data related to the incoming events so that it can be enriched without making a remote procedure call to retrieve the related data.
Spring Cloud Data Flow supports partitioning by configuring Spring Cloud Stream’s output and input bindings. Spring Cloud Stream provides a common abstraction for implementing partitioned processing use cases in a uniform fashion across different types of middleware. Partitioning can thus be used whether the broker itself is naturally partitioned (e.g., Kafka topics) or not (e.g., RabbitMQ). The following image shows how data could be partitioned into two buckets, such that each instance of the average processor application consumes a unique set of data.
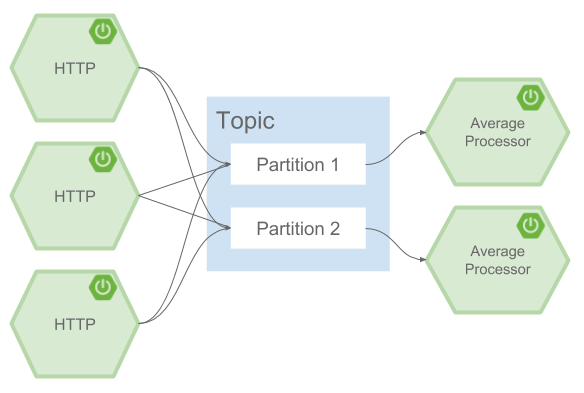
To use a simple partitioning strategy in Spring Cloud Data Flow, you only need set the instance count for each application in the stream and a partitionKeyExpression
producer property when deploying the stream. The partitionKeyExpression
identifies what part of the message will be used as the key to partition data in the underlying middleware. An ingest
stream can be defined as http | averageprocessor | cassandra
(Note that the Cassandra sink isn’t shown in the diagram above). Suppose the payload being sent to the http source was in JSON format and had a field called sensorId
. Deploying the stream with the shell command stream deploy ingest --propertiesFile ingestStream.properties
where the contents of the file ingestStream.properties
are
deployer.http.count=3
deployer.averageprocessor.count=2
app.http.producer.partitionKeyExpression=payload.sensorId
will deploy the stream such that all the input and output destinations are configured for data to flow through the applications but also ensure that a unique set of data is always delivered to each averageprocessor instance. In this case the default algorithm is to evaluate payload.sensorId % partitionCount
where the partitionCount
is the application count in the case of RabbitMQ and the partition count of the topic in the case of Kafka.
Please refer to Passing stream partition properties during stream deployment for additional strategies to partition streams during deployment and how they map onto the underlying Spring Cloud Stream Partitioning properties.
Also note, that you can’t currently scale partitioned streams. Read the section Scaling at runtime for more information.
7.4. Message Delivery Guarantees
Streams are composed of applications that use the Spring Cloud Stream library as the basis for communicating with the underlying messaging middleware product. Spring Cloud Stream also provides an opinionated configuration of middleware from several vendors, in particular providing persistent publish-subscribe semantics.
The Binder abstraction in Spring Cloud Stream is what connects the application to the middleware. There are several configuration properties of the binder that are portable across all binder implementations and some that are specific to the middleware.
For consumer applications there is a retry policy for exceptions generated during message handling. The retry policy is configured using the common consumer properties maxAttempts
, backOffInitialInterval
, backOffMaxInterval
, and backOffMultiplier
. The default values of these properties will retry the callback method invocation 3 times and wait one second for the first retry. A backoff multiplier of 2 is used for the second and third attempts.
When the number of retry attempts has exceeded the maxAttempts
value, the exception and the failed message will become the payload of a message and be sent to the application’s error channel. By default, the default message handler for this error channel logs the message. You can change the default behavior in your application by creating your own message handler that subscribes to the error channel.
Spring Cloud Stream also supports a configuration option for both Kafka and RabbitMQ binder implementations that will send the failed message and stack trace to a dead letter queue. The dead letter queue is a destination and its nature depends on the messaging middleware (e.g in the case of Kafka it is a dedicated topic). To enable this for RabbitMQ set the consumer properties republishtoDlq
and autoBindDlq
and the producer property autoBindDlq
to true when deploying the stream. To always apply these producer and consumer properties when deploying streams, configure them as common application properties when starting the Data Flow server.
Additional messaging delivery guarantees are those provided by the underlying messaging middleware that is chosen for the application for both producing and consuming applications. Refer to the Kafka Consumer and Producer and Rabbit Consumer and Producer documentation for more details. You will find extensive declarative support for all the native QOS options.
8. Analytics
Spring Cloud Data Flow is aware of certain Sink applications that will write counter data to Redis and provides an REST endpoint to read counter data. The types of counters supported are
-
Counter - Counts the number of messages it receives, optionally storing counts in a separate store such as redis.
-
Field Value Counter - Counts occurrences of unique values for a named field in a message payload
-
Aggregate Counter - Stores total counts but also retains the total count values for each minute, hour day and month.
It is important to note that the timestamp that is used in the aggregate counter can come from a field in the message itself so that out of order messages are properly accounted.
9. Task Applications
The Spring Cloud Task programming model provides:
-
Persistence of the Task’s lifecycle events and exit code status.
-
Lifecycle hooks to execute code before or after a task execution.
-
Emit task events to a stream (as a source) during the task lifecycle.
-
Integration with Spring Batch Jobs.
10. Data Flow Server
10.1. Endpoints
The Data Flow Server uses an embedded servlet container and exposes REST endpoints for creating, deploying, undeploying, and destroying streams and tasks, querying runtime state, analytics, and the like. The Data Flow Server is implemented using Spring’s MVC framework and the Spring HATEOAS library to create REST representations that follow the HATEOAS principle.
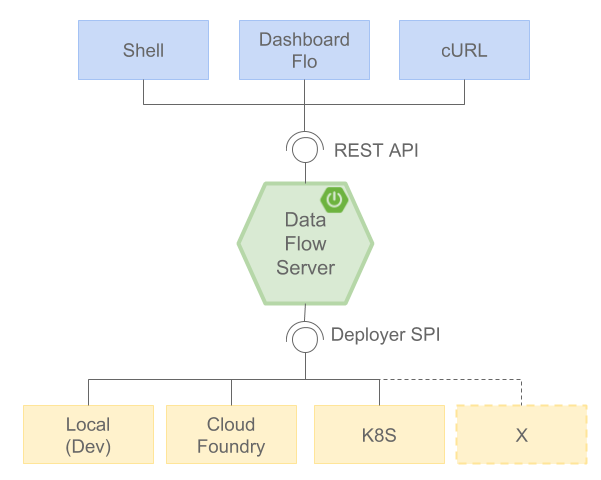
10.2. Customization
Each Data Flow Server executable jar targets a single runtime by delegating to the implementation of the deployer Service Provider Interface found on the classpath.
We provide a Data Flow Server executable jar that targets a single runtime. The Data Flow server delegates to the implementation of the deployer Service Provider Interface found on the classpath. In the current version, there are no endpoints specific to a target runtime, but may be available in future releases as a convenience to access runtime specific features
While we provide a server executable for each of the target runtimes you can also create your own customized server application using Spring Initialzr. This let’s you add or remove functionality relative to the executable jar we provide. For example, adding additional security implementations, custom endpoints, or removing Task or Analytics REST endpoints. You can also enable or disable some features through the use of feature toggles.
10.3. Security
The Data Flow Server executable jars support basic http, LDAP(S), File-based, and OAuth 2.0 authentication to access its endpoints. Refer to the security section for more information.
Authorization via groups is planned for a future release.
11. Runtime
11.1. Fault Tolerance
The target runtimes supported by Data Flow all have the ability to restart a long lived application should it fail. Spring Cloud Data Flow sets up whatever health probe is required by the runtime environment when deploying the application.
The collective state of all applications that comprise the stream is used to determine the state of the stream. If an application fails, the state of the stream will change from ‘deployed’ to ‘partial’.
11.2. Resource Management
Each target runtime lets you control the amount of memory, disk and CPU that is allocated to each application. These are passed as properties in the deployment manifest using key names that are unique to each runtime. Refer to the each platforms server documentation for more information.
11.3. Scaling at runtime
When deploying a stream, you can set the instance count for each individual application that comprises the stream. Once the stream is deployed, each target runtime lets you control the target number of instances for each individual application. Using the APIs, UIs, or command line tools for each runtime, you can scale up or down the number of instances as required. Future work will provide a portable command in the Data Flow Server to perform this operation.
Currently, this is not supported with the Kafka binder (based on the 0.8 simple consumer at the time of the release), as well as partitioned streams, for which the suggested workaround is redeploying the stream with an updated number of instances. Both cases require a static consumer set up based on information about the total instance count and current instance index, a limitation intended to be addressed in future releases. For example, Kafka 0.9 and higher provides good infrastructure for scaling applications dynamically and will be available as an alternative to the current Kafka 0.8 based binder in the near future. One specific concern regarding scaling partitioned streams is the handling of local state, which is typically reshuffled as the number of instances is changed. This is also intended to be addressed in the future versions, by providing first class support for local state management.
11.4. Application Versioning
Application versioning, that is upgrading or downgrading an application from one version to another, is not directly supported by Spring Cloud Data Flow. You must rely on specific target runtime features to perform these operational tasks.
The roadmap for Spring Cloud Data Flow will deploy applications that are compatible with Spinnaker to manage the complete application lifecycle. This also includes automated canary analysis backed by application metrics. Portable commands in the Data Flow server to trigger pipelines in Spinnaker are also planned.
Getting started
If you’re just getting started with Spring Cloud Data Flow, this is the section for you! Here we answer the basic “what?”, “how?” and “why?” questions. You’ll find a gentle introduction to Spring Cloud Data Flow along with installation instructions. We’ll then build our first Spring Cloud Data Flow application, discussing some core principles as we go.
12. System Requirements
You need Java installed (Java 8 or later), and to build, you need to have Maven installed as well.
You need to have an RDBMS for storing stream, task and app states in the database. The local
Data Flow server by default uses embedded H2 database for this.
You also need to have Redis running if you are running any streams that involve analytics applications. Redis may also be required run the unit/integration tests.
13. Deploying Spring Cloud Data Flow Local Server
-
Download the Spring Cloud Data Flow Server and Shell apps:
wget http://repo.spring.io/milestone/org/springframework/cloud/spring-cloud-dataflow-server-local/1.2.0.RC2/spring-cloud-dataflow-server-local-1.2.0.RC2.jar wget http://repo.spring.io/milestone/org/springframework/cloud/spring-cloud-dataflow-shell/1.2.0.RC2/spring-cloud-dataflow-shell-1.2.0.RC2.jar
-
Launch the Data Flow Server
-
Since the Data Flow Server is a Spring Boot application, you can run it just by using
java -jar
.$ java -jar spring-cloud-dataflow-server-local-1.2.0.RC2.jar
-
-
Launch the shell:
$ java -jar spring-cloud-dataflow-shell-1.2.0.RC2.jar
If the Data Flow Server and shell are not running on the same host, point the shell to the Data Flow server URL:
server-unknown:>dataflow config server http://198.51.100.0 Successfully targeted http://198.51.100.0 dataflow:>
By default, the application registry will be empty. If you would like to register all out-of-the-box stream applications built with the Kafka binder in bulk, you can with the following command. For more details, review how to register applications.
$ dataflow:>app import --uri http://bit.ly/Bacon-RELEASE-stream-applications-kafka-10-maven
Depending on your environment, you may need to configure the Data Flow Server to point to a custom Maven repository location or configure proxy settings. See Maven Configuration for more information. -
You can now use the shell commands to list available applications (source/processors/sink) and create streams. For example:
dataflow:> stream create --name httptest --definition "http --server.port=9000 | log" --deploy
You will need to wait a little while until the apps are actually deployed successfully before posting data. Look in the log file of the Data Flow server for the location of the log files for the http
andlog
applications. Tail the log file for each application to verify the application has started.Now post some data
dataflow:> http post --target http://localhost:9000 --data "hello world"
Look to see if hello world
ended up in log files for the log
application.
When deploying locally, each app (and each app instance, in case of |
In case you encounter unexpected errors when executing shell commands, you can
retrieve more detailed error information by setting the exception logging level
to
|
13.1. Maven Configuration
If you want to override specific maven configuration properties (remote repositories, proxies, etc.) and/or run the Data Flow Server behind a proxy, you need to specify those properties as command line arguments when starting the Data Flow Server. For example:
$ java -jar spring-cloud-dataflow-server-local-1.2.0.RC2.jar --maven.localRepository=mylocal
--maven.remote-repositories.repo1.url=https://repo1
--maven.remote-repositories.repo1.auth.username=user1
--maven.remote-repositories.repo1.auth.password=pass1
--maven.remote-repositories.repo2.url=https://repo2 --maven.proxy.host=proxy1
--maven.proxy.port=9010 --maven.proxy.auth.username=proxyuser1
--maven.proxy.auth.password=proxypass1
By default, the protocol is set to http
. You can omit the auth properties if the proxy doesn’t need a username and password.
By default, the maven localRepository
is set to ${user.home}/.m2/repository/
,
and repo.spring.io/libs-snapshot
will be the only remote repository. Like in the above example, the remote
repositories can be specified along with their authentication (if needed). If the remote repositories are behind a proxy,
then the proxy properties can be specified as above.
If you want to pass these properties as environment properties, then you need to use SPRING_APPLICATION_JSON
to set
these properties and pass SPRING_APPLICATION_JSON
as environment variable as below:
$ SPRING_APPLICATION_JSON='{ "maven": { "local-repository": null,
"remote-repositories": { "repo1": { "url": "https://repo1", "auth": { "username": "repo1user", "password": "repo1pass" } }, "repo2": { "url": "https://repo2" } },
"proxy": { "host": "proxyhost", "port": 9018, "auth": { "username": "proxyuser", "password": "proxypass" } } } }' java -jar spring-cloud-dataflow-server-local-{project-version}.jar
14. Application Configuration
You can use the following configuration properties of the Data Flow server to customize how applications are deployed.
spring.cloud.deployer.local.workingDirectoriesRoot=java.io.tmpdir # Directory in which all created processes will run and create log files.
spring.cloud.deployer.local.deleteFilesOnExit=true # Whether to delete created files and directories on JVM exit.
spring.cloud.deployer.local.envVarsToInherit=TMP,LANG,LANGUAGE,"LC_.*. # Array of regular expression patterns for environment variables that will be passed to launched applications.
spring.cloud.deployer.local.javaCmd=java # Command to run java.
spring.cloud.deployer.local.shutdownTimeout=30 # Max number of seconds to wait for app shutdown.
spring.cloud.deployer.local.javaOpts= # The Java options to pass to the JVM
When deploying the application you can also set deployer properties prefixed with deployer.<name of application>
, So for example to set Java options for the time application in the ticktock
stream, use the following stream deployment properties.
dataflow:> stream create --name ticktock --definition "time --server.port=9000 | log"
dataflow:> stream deploy --name ticktock --properties "deployer.time.local.javaOpts=-Xmx2048m -Dtest=foo"
As a convenience you can set the property deployer.memory
to set the Java option -Xmx
. So for example,
dataflow:> stream deploy --name ticktock --properties "deployer.time.memory=2048m"
At deployment time, if you specify an -Xmx
option in the deployer.<app>.local.javaOpts
property in addition to a value of the deployer.<app>.local.memory
option, the value in the javaOpts
property has precedence. Also, the javaOpts
property set when deploying the application has precedence over the Data Flow server’s spring.cloud.deployer.local.javaOpts
property.
Server Configuration
In this section you will learn how to configure Spring Cloud Data Flow server’s features such as the relational database to use and security.
15. Feature Toggles
Data Flow server offers specific set of features that can be enabled/disabled when launching. These features include all the lifecycle operations, REST endpoints (server, client implementations including Shell and the UI) for:
-
Streams
-
Tasks
-
Analytics
One can enable, disable these features by setting the following boolean properties when launching the Data Flow server:
-
spring.cloud.dataflow.features.streams-enabled
-
spring.cloud.dataflow.features.tasks-enabled
-
spring.cloud.dataflow.features.analytics-enabled
By default, all the features are enabled.
Note: Since analytics feature is enabled by default, the Data Flow server is expected to have a valid Redis store available as analytic repository as we provide a default implementation of analytics based on Redis.
This also means that the Data Flow server’s health
depends on the redis store availability as well.
If you do not want to enabled HTTP endpoints to read analytics data written to Redis, then disable the analytics feature using the property mentioned above.
The REST endpoint /features
provides information on the features enabled/disabled.
16. Database Configuration
Spring Cloud Data Flow provides schemas for H2, HSQLDB, MySQL, Oracle, Postgresql, DB2 and SqlServer that will be automatically created when the server starts.
The JDBC drivers for MySQL (via MariaDB driver), HSQLDB, PostgreSQL along with embedded H2 are available out of the box. If you are using any other database, then the corresponding JDBC driver jar needs to be on the classpath of the server.
The database properties can be passed as command-line arguments to the Data Flow Server.
For instance, If you are using MySQL:
java -jar spring-cloud-dataflow-server-local/target/spring-cloud-dataflow-server-local-1.0.0.BUILD-SNAPSHOT.jar \
--spring.datasource.url=jdbc:mysql:<db-info> \
--spring.datasource.username=<user> \
--spring.datasource.password=<password> \
--spring.datasource.driver-class-name=org.mariadb.jdbc.Driver &
For PostgreSQL:
java -jar spring-cloud-dataflow-server-local/target/spring-cloud-dataflow-server-local-1.0.0.BUILD-SNAPSHOT.jar \
--spring.datasource.url=jdbc:postgresql:<db-info> \
--spring.datasource.username=<user> \
--spring.datasource.password=<password> \
--spring.datasource.driver-class-name=org.postgresql.Driver &
For HSQLDB:
java -jar spring-cloud-dataflow-server-local/target/spring-cloud-dataflow-server-local-1.0.0.BUILD-SNAPSHOT.jar \
--spring.datasource.url=jdbc:hsqldb:<db-info> \
--spring.datasource.username=SA \
--spring.datasource.driver-class-name=org.hsqldb.jdbc.JDBCDriver &
There is a schema update to the Spring Cloud Data Flow datastore when
upgrading from version 1.0.x to 1.1.x . Migration scripts for specific
database types can be found
here.
|
If you wish to use an external H2 database instance instead of the one
embedded with Spring Cloud Data Flow set the
spring.dataflow.embedded.database.enabled property to false. If
spring.dataflow.embedded.database.enabled is set to false or a database
other than h2 is specified as the datasource the embedded database will not
start.
|
17. Security
By default, the Data Flow server is unsecured and runs on an unencrypted HTTP connection. You can secure your REST endpoints, as well as the Data Flow Dashboard by enabling HTTPS and requiring clients to authenticate using either:
-
Traditional Authentication (Incl. Basic Authentication)
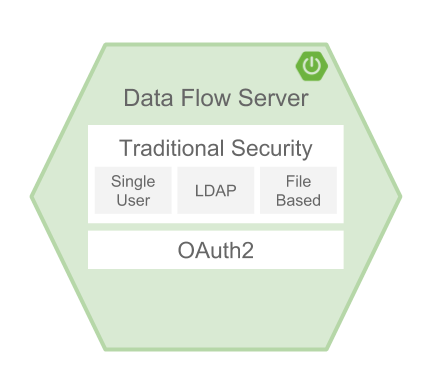
When choosing traditional authentication, the Spring Cloud Data Flow server will be the main authentication point, using Spring Security under the covers. When selecting this option, users then need to further define their preferred authentication mechanism aka select the desired authentication backing store:
When choosing between traditional authentication or OAuth2, keep in mind that both options are mutually exclusive. Please refer to the sub-chapters below for a more detailed discussion.
By default, the REST endpoints (administration, management and health), as well as the Dashboard UI do not require authenticated access. |
17.1. Enabling HTTPS
By default, the dashboard, management, and health endpoints use HTTP as a transport.
You can switch to HTTPS easily, by adding a certificate to your configuration in
application.yml
.
server:
port: 8443 (1)
ssl:
key-alias: yourKeyAlias (2)
key-store: path/to/keystore (3)
key-store-password: yourKeyStorePassword (4)
key-password: yourKeyPassword (5)
trust-store: path/to/trust-store (6)
trust-store-password: yourTrustStorePassword (7)
1 | As the default port is 9393 , you may choose to change the port to a more common HTTPs-typical port. |
2 | The alias (or name) under which the key is stored in the keystore. |
3 | The path to the keystore file. Classpath resources may also be specified, by using the classpath prefix: classpath:path/to/keystore |
4 | The password of the keystore. |
5 | The password of the key. |
6 | The path to the truststore file. Classpath resources may also be specified, by using the classpath prefix: classpath:path/to/trust-store |
7 | The password of the trust store. |
If HTTPS is enabled, it will completely replace HTTP as the protocol over which the REST endpoints and the Data Flow Dashboard interact. Plain HTTP requests will fail - therefore, make sure that you configure your Shell accordingly. |
17.1.1. Using Self-Signed Certificates
For testing purposes or during development it might be convenient to create self-signed certificates. To get started, execute the following command to create a certificate:
$ keytool -genkey -alias dataflow -keyalg RSA -keystore dataflow.keystore \
-validity 3650 -storetype JKS \
-dname "CN=localhost, OU=Spring, O=Pivotal, L=Kailua-Kona, ST=HI, C=US" (1)
-keypass dataflow -storepass dataflow
1 | CN is the only important parameter here. It should match the domain you are trying to access, e.g. localhost . |
Then add the following to your application.yml
file:
server:
port: 8443
ssl:
enabled: true
key-alias: dataflow
key-store: "/your/path/to/dataflow.keystore"
key-store-type: jks
key-store-password: dataflow
key-password: dataflow
This is all that’s needed for the Data Flow Server. Once you start the server, you should be able to access it via https://localhost:8443/. As this is a self-signed certificate, you will hit a warning in your browser, that you need to ignore.
17.1.2. Self-Signed Certificates and the Shell
By default self-signed certificates are an issue for the Shell and additional steps are necessary to make the Shell work with self-signed certificates. Two options are available:
-
Add the self-signed certificate to the JVM truststore
-
Skip certificate validation
Add the self-signed certificate to the JVM truststore
In order to use the JVM truststore option, we need to export the previously created certificate from the keystore:
$ keytool -export -alias dataflow -keystore dataflow.keystore -file dataflow_cert -storepass dataflow
Next, we need to create a truststore which the Shell will use:
$ keytool -importcert -keystore dataflow.truststore -alias dataflow -storepass dataflow -file dataflow_cert -noprompt
Now, you are ready to launch the Data Flow Shell using the following JVM arguments:
$ java -Djavax.net.ssl.trustStorePassword=dataflow \
-Djavax.net.ssl.trustStore=/path/to/dataflow.truststore \
-Djavax.net.ssl.trustStoreType=jks \
-jar spring-cloud-dataflow-shell-1.2.0.RC2.jar
In case you run into trouble establishing a connection via SSL, you can enable additional
logging by using and setting the |
Don’t forget to target the Data Flow Server with:
dataflow:> dataflow config server https://localhost:8443/
Skip Certificate Validation
Alternatively, you can also bypass the certification validation by providing the
optional command-line parameter --dataflow.skip-ssl-validation=true
.
Using this command-line parameter, the shell will accept any (self-signed) SSL certificate.
If possible you should avoid using this option. Disabling the trust manager defeats the purpose of SSL and makes you vulnerable to man-in-the-middle attacks. |
17.2. Traditional Authentication
When using traditional authentication Spring Cloud Data Flow will be the sole authentication provider. In that case Dataflow REST API users would use Basic Authentication to access the endpoints.
When using that option, users have a choice of 3 backing stores for authentication details:
-
Single User Authentication using Spring Boot properties
-
File-based authentication for multiple users using a Yaml file
-
Ldap Authentication
17.2.1. Single User Authentication
This is the simplest option and mimics the behavior of the default Spring Boot user
user-experience. It can be enabled by adding the following to application.yml
or via
environment variables:
security:
basic:
enabled: true (1)
realm: Spring Cloud Data Flow (2)
1 | Enables basic authentication. Must be set to true for security to be enabled. |
2 | (Optional) The realm for Basic authentication. Will default to Spring if not explicitly set. |
Current versions of Chrome do not display the realm. Please see the following Chromium issue ticket for more information. |
In this use-case, the underlying Spring Boot will auto-create a user called user with an auto-generated password which will be printed out to the console upon startup.
With this setup, the generated user will have all main roles assigned:
-
VIEW
-
CREATE
-
MANAGE

You can customize the user yourself using the following properties:
security.user.name=user # Default user name.
security.user.password= # Password for the default user name. A random password is logged on startup by default.
security.user.role=VIEW, CREATE, MANAGE # Granted roles for the default user name.
Please be aware of inherent issues of Basic Authentication and logging out, since the credentials are cached by the browser and simply browsing back to application pages will log you back in. |
Of course, you can also pass in credentials using system properties, environment variables or command-line arguments as this is standard Spring Boot behavior. For instance in the following example, command-line arguments are used to specify the user credentials:
$ java -jar spring-cloud-dataflow-server-local-1.2.0.RC2.jar\
--security.basic.enabled=true \
--security.user.name=test \
--security.user.password=pass \
--security.user.role=VIEW
If you need to define more than one file-based user account, please take a look at File based authentication.
17.2.2. File based authentication
By default Spring Boot allows you to only specify one single user. Spring Cloud Data Flow also supports the listing of more than one user in a configuration file, as described below. Each user must be assigned a password and one or more roles:
security:
basic:
enabled: true
realm: Spring Cloud Data Flow
spring:
cloud:
dataflow:
security:
authentication:
file:
enabled: true (1)
users: (2)
bob: bobspassword, ROLE_MANAGE (3)
alice: alicepwd, ROLE_VIEW, ROLE_CREATE
1 | Enables file based authentication |
2 | This is a yaml map of username to password |
3 | Each map value is made of a corresponding password and role(s), comma separated |
17.2.3. LDAP Authentication
Spring Cloud Data Flow also supports authentication against an LDAP server (Lightweight Directory Access Protocol), providing support for the following 2 modes:
-
Direct bind
-
Search and bind
When the LDAP authentication option is activated, the default single user mode is turned off.
In direct bind mode, a pattern is defined for the user’s distinguished name (DN), using a placeholder for the username. The authentication process derives the distinguished name of the user by replacing the placeholder and use it to authenticate a user against the LDAP server, along with the supplied password. You can set up LDAP direct bind as follows:
security:
basic:
enabled: true
realm: Spring Cloud Data Flow
spring:
cloud:
dataflow:
security:
authentication:
ldap:
enabled: true (1)
url: ldap://ldap.example.com:3309 (2)
userDnPattern: uid={0},ou=people,dc=example,dc=com (3)
1 | Enables LDAP authentication |
2 | The URL for the LDAP server |
3 | The distinguished name (DN) pattern for authenticating against the server |
The search and bind mode involves connecting to an LDAP server, either anonymously or with a fixed account, and searching for the distinguished name of the authenticating user based on its username, and then using the resulting value and the supplied password for binding to the LDAP server. This option is configured as follows:
security:
basic:
enabled: true
realm: Spring Cloud Data Flow
spring:
cloud:
dataflow:
security:
authentication:
ldap:
enabled: true (1)
url: ldap://localhost:10389 (2)
managerDn: uid=admin,ou=system (3)
managerPassword: secret (4)
userSearchBase: ou=otherpeople,dc=example,dc=com (5)
userSearchFilter: uid={0} (6)
1 | Enables LDAP integration |
2 | The URL of the LDAP server |
3 | A DN for to authenticate to the LDAP server, if anonymous searches are not supported (optional, required together with next option) |
4 | A password to authenticate to the LDAP server, if anonymous searches are not supported (optional, required together with previous option) |
5 | The base for searching the DN of the authenticating user (serves to restrict the scope of the search) |
6 | The search filter for the DN of the authenticating user |
For more information, please also see the chapter LDAP Authentication of the Spring Security reference guide. |
LDAP Transport Security
When connecting to an LDAP server, you typically (In the LDAP world) have 2 options in order to establish a connection to an LDAP server securely:
-
LDAP over SSL (LDAPs)
-
Start Transport Layer Security (Start TLS is defined in RFC2830)
As of Spring Cloud Data Flow 1.1.0 only LDAPs is supported out-of-the-box. When using
official certificates no special configuration is necessary, in order to connect
to an LDAP Server via LDAPs. Just change the url format to ldaps, e.g. ldaps://localhost:636
.
In case of using self-signed certificates, the setup for your Spring Cloud Data Flow server becomes slightly more complex. The setup is very similar to Using Self-Signed Certificates (Please read first) and Spring Cloud Data Flow needs to reference a trustStore in order to work with your self-signed certificates.
While useful during development and testing, please never use self-signed certificates in production! |
Ultimately you have to provide a set of system properties to reference the trustStore and its credentials when starting the server:
$ java -Djavax.net.ssl.trustStorePassword=dataflow \
-Djavax.net.ssl.trustStore=/path/to/dataflow.truststore \
-Djavax.net.ssl.trustStoreType=jks \
-jar spring-cloud-starter-dataflow-server-local-1.2.0.RC2.jar
As mentioned above, another option to connect to an LDAP server securely is via Start TLS. In the LDAP world, LDAPs is technically even considered deprecated in favor of Start TLS. However, this option is currently not supported out-of-the-box by Spring Cloud Data Flow.
Please follow the following issue tracker ticket to track its implementation. You may also want to look at the Spring LDAP reference documentation chapter on Custom DirContext Authentication Processing for further details.
17.2.4. Shell Authentication
When using traditional authentication with the Data Flow Shell, you typically provide a username and password using command-line arguments, e.g.:
$ java -jar target/spring-cloud-dataflow-shell-1.2.0.RC2.jar \
--dataflow.username=myuser \ (1)
--dataflow.password=mysecret (2)
1 | If authentication is enabled the username must be provided |
2 | If the password is not provided, the shell will prompt for it |
Alternatively, you can target a Data Flow server also from within the Shell:
server-unknown:>dataflow config server
--uri http://localhost:9393 \ (1)
--username myuser \ (2)
--password mysecret \ (3)
--skip-ssl-validation true \ (4)
1 | Optional, defaults to localhost:9393 |
2 | Mandatory if security is enabled |
3 | If security is enabled, and the password is not provided, the user will be promted for it |
4 | Optional, ignores certificate errors (When using self-signed certificates). Use cautiously! |

17.2.5. Customizing authorization
All of the above deals with authentication, i.e. how to assess the identity of the user. Irrespective of the option chosen, you can also customize authorization i.e. who can do what.
The default scheme uses three roles to protect the REST endpoints that Spring Cloud Data Flow exposes:
-
ROLE_VIEW for anything that relates to retrieving state
-
ROLE_CREATE for anything that involves creating, deleting or mutating the state of the system
-
ROLE_MANAGE for boot management endpoints.
All of those defaults are specified in dataflow-server-defaults.yml
which is
part of the Spring Cloud Data Flow Core Module. Nonetheless, you can
override those, if desired, e.g. in application.yml
. The configuration takes
the form of a YAML list (as some rules may have precedence over others) and so
you’ll need to copy/paste the whole list and tailor it to your needs (as there is no way to merge lists). Always refer to your version of application.yml
, as the snippet reproduced below may be out-dated. The default rules are as such:
spring:
cloud:
dataflow:
security:
authorization:
enabled: true
rules:
# Metrics
- GET /metrics/streams => hasRole('ROLE_VIEW')
# About
- GET /about => hasRole('ROLE_VIEW')
# Metrics
- GET /metrics/** => hasRole('ROLE_VIEW')
- DELETE /metrics/** => hasRole('ROLE_CREATE')
# Boot Endpoints
- GET /management/** => hasRole('ROLE_MANAGE')
# Apps
- GET /apps => hasRole('ROLE_VIEW')
- GET /apps/** => hasRole('ROLE_VIEW')
- DELETE /apps/** => hasRole('ROLE_CREATE')
- POST /apps => hasRole('ROLE_CREATE')
- POST /apps/** => hasRole('ROLE_CREATE')
# Completions
- GET /completions/** => hasRole('ROLE_CREATE')
# Job Executions & Batch Job Execution Steps && Job Step Execution Progress
- GET /jobs/executions => hasRole('ROLE_VIEW')
- PUT /jobs/executions/** => hasRole('ROLE_CREATE')
- GET /jobs/executions/** => hasRole('ROLE_VIEW')
# Batch Job Instances
- GET /jobs/instances => hasRole('ROLE_VIEW')
- GET /jobs/instances/* => hasRole('ROLE_VIEW')
# Running Applications
- GET /runtime/apps => hasRole('ROLE_VIEW')
- GET /runtime/apps/** => hasRole('ROLE_VIEW')
# Stream Definitions
- GET /streams/definitions => hasRole('ROLE_VIEW')
- GET /streams/definitions/* => hasRole('ROLE_VIEW')
- GET /streams/definitions/*/related => hasRole('ROLE_VIEW')
- POST /streams/definitions => hasRole('ROLE_CREATE')
- DELETE /streams/definitions/* => hasRole('ROLE_CREATE')
- DELETE /streams/definitions => hasRole('ROLE_CREATE')
# Stream Deployments
- DELETE /streams/deployments/* => hasRole('ROLE_CREATE')
- DELETE /streams/deployments => hasRole('ROLE_CREATE')
- POST /streams/deployments/* => hasRole('ROLE_CREATE')
# Task Definitions
- POST /tasks/definitions => hasRole('ROLE_CREATE')
- DELETE /tasks/definitions/* => hasRole('ROLE_CREATE')
- GET /tasks/definitions => hasRole('ROLE_VIEW')
- GET /tasks/definitions/* => hasRole('ROLE_VIEW')
# Task Executions
- GET /tasks/executions => hasRole('ROLE_VIEW')
- GET /tasks/executions/* => hasRole('ROLE_VIEW')
- POST /tasks/executions => hasRole('ROLE_CREATE')
- DELETE /tasks/executions/* => hasRole('ROLE_CREATE')
The format of each line is the following:
HTTP_METHOD URL_PATTERN '=>' SECURITY_ATTRIBUTE
where
-
HTTP_METHOD is one http method, capital case
-
URL_PATTERN is an Ant style URL pattern
-
SECURITY_ATTRIBUTE is a SpEL expression (see docs.spring.io/spring-security/site/docs/current/reference/htmlsingle/#el-access)
-
each of those separated by one or several blank characters (spaces, tabs, etc.)
Be mindful that the above is indeed a YAML list, not a map (thus the use of '-' dashes at the start of each line) that lives under the spring.cloud.dataflow.security.authorization.rules
key.
In case you are solely interested in authentication but not authorization,
for instance every user shall have have access to all endpoints, then you can also
set |
If you are using basic security configuration by using security properties then it is important to set the roles for the users.
For instance,
java -jar spring-cloud-dataflow-server-local/target/spring-cloud-dataflow-server-local-1.2.0.RC2.jar \
--security.basic.enabled=true \
--security.user.name=test \
--security.user.password=pass \
--security.user.role=VIEW
17.2.6. Authorization - Shell and Dashboard Behavior
When authorization is enabled, the Dashboard and the Shell will be role-aware, meaning that depending on the assigned role(s), not all functionality may be visible.
For instance, Shell commands, for which the user does not have the necessary roles for, will be marked as unavailable.
Currently, the Shell’s |
Similarly for the Dashboard, the UI will not show pages, or page elements, for which the user is not authorized for.
17.2.7. Authorization with Ldap
When configuring Ldap for authentication, you can also specify the group-role-attribute
in conjunction with group-search-base
and group-search-filter
.
The group role attribure contains the name of the role. If not specified, the
ROLE_MANAGE
role is populated by default.
For further information, please refer to Configuring an LDAP Server of the Spring Security reference guide.
17.3. OAuth 2.0
OAuth 2.0 allows you to integrate Spring Cloud Data Flow into Single Sign On (SSO) environments. The following 3 OAuth2 Grant Types will be used:
-
Authorization Code - Used for the GUI (Browser) integration. You will be redirected to your OAuth Service for authentication
-
Password - Used by the shell (And the REST integration), so you can login using username and password
-
Client Credentials - Retrieve an Access Token directly from your OAuth provider and pass it to the Dataflow server using the Authorization Http header.
The REST endpoints can be accessed using 2 ways:
-
Basic Authentication which will use the Password Grant Type under the covers to authenticate with your OAuth2 service
-
Access Token which will use the Client Credentials Grant Type under the covers
When authentication is set up, it is strongly recommended to enable HTTPS as well, especially in production environments. |
You can turn on OAuth2 authentication by adding the following to application.yml
or via
environment variables:
security:
oauth2:
client:
client-id: myclient (1)
client-secret: mysecret
access-token-uri: http://127.0.0.1:9999/oauth/token
user-authorization-uri: http://127.0.0.1:9999/oauth/authorize
resource:
user-info-uri: http://127.0.0.1:9999/me
1 | Providing the Client Id in the OAuth Configuration Section will activate OAuth2 security |
As of the current version, Spring Cloud Data Flow does not provide finer-grained authorization when OAUTH is used as authentication mechanism. Thus, once you are logged in, you have full access to all functionality. |
You can verify that basic authentication is working properly using curl:
$ curl -u myusername:mypassword http://localhost:9393/
As a result you should see a list of available REST endpoints.
Besides Basic Authentication, you can also provide an Access Token in order to access the REST Api. In order to make that happen, you would retrieve an OAuth2 Access Token from your OAuth2 provider first, and then pass that Access Token to the REST Api using the Authorization Http header:
$ curl -H "Authorization: Bearer <ACCESS_TOKEN>" http://localhost:9393/
17.3.1. OAuth Authentication using the Spring Cloud Data Flow Shell
If your OAuth2 provider supports the Password Grant Type you can start the Data Flow Shell with:
$ java -jar spring-cloud-dataflow-shell-1.2.0.RC2.jar \
--dataflow.uri=http://localhost:9393 \
--dataflow.username=my_username --dataflow.password=my_password
Keep in mind that when authentication for Spring Cloud Data Flow is enabled, the underlying OAuth2 provider must support the Password OAuth2 Grant Type, if you want to use the Shell. |
From within the Data Flow Shell you can also provide credentials using:
dataflow config server --uri http://localhost:9393 --username my_username --password my_password
Once successfully targeted, you should see the following output:
dataflow:>dataflow config info
dataflow config info
╔═══════════╤═══════════════════════════════════════╗
║Credentials│[username='my_username, password=****']║
╠═══════════╪═══════════════════════════════════════╣
║Result │ ║
║Target │http://localhost:9393 ║
╚═══════════╧═══════════════════════════════════════╝
17.3.2. OAuth2 Authentication Examples
Local OAuth2 Server
With Spring Security OAuth you can easily create your own OAuth2 Server with the following 2 simple annotations:
-
@EnableResourceServer
-
@EnableAuthorizationServer
A working example application can be found at:
Simply clone the project, built and start it. Furthermore configure Spring Cloud Data Flow with the respective Client Id and Client Secret.
Authentication using GitHub
If you rather like to use an existing OAuth2 provider, here is an example for GitHub. First you need to Register a new application under your GitHub account at:
When running a default version of Spring Cloud Data Flow locally, your GitHub configuration should look like the following:
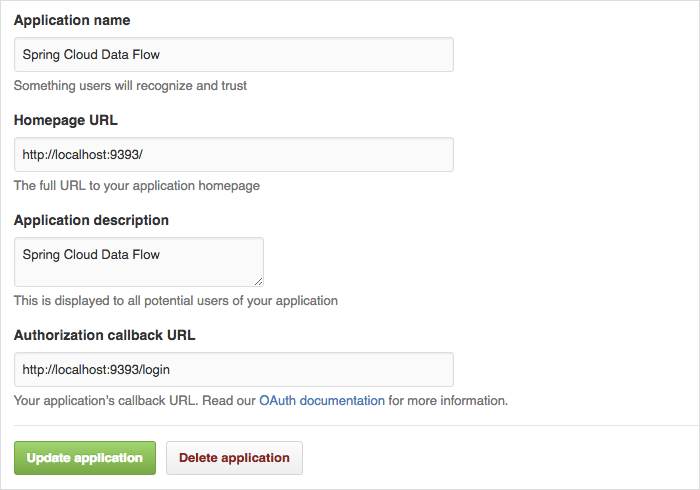
For the Authorization callback URL you will enter Spring Cloud Data Flow’s Login URL, e.g. localhost:9393/login .
|
Configure Spring Cloud Data Flow with the GitHub relevant Client Id and Secret:
security:
oauth2:
client:
client-id: your-github-client-id
client-secret: your-github-client-secret
access-token-uri: https://github.com/login/oauth/access_token
user-authorization-uri: https://github.com/login/oauth/authorize
resource:
user-info-uri: https://api.github.com/user
GitHub does not support the OAuth2 password grant type. As such you cannot use the Spring Cloud Data Flow Shell in conjunction with GitHub. |
17.4. Securing the Spring Boot Management Endpoints
When enabling security, please also make sure that the Spring Boot HTTP Management Endpoints
are secured as well. You can enable security for the management endpoints by adding the following to application.yml
:
management:
contextPath: /management
security:
enabled: true
If you don’t explicitly enable security for the management endpoints,
you may end up having unsecured REST endpoints, despite security.basic.enabled
being set to true .
|
18. Monitoring and Management
The Spring Cloud Data Flow server is a Spring Boot application that includes the Actuator library, which adds several production ready features to help you monitor and manage your application.
The Actuator library adds http endpoints under the context path /management
that is also
a discovery page for available endpoints. For example, there is a health
endpoint
that shows application health information and an env
that lists properties from
Spring’s ConfigurableEnvironment
. By default only the health and application info
endpoints are accessible. The other endpoints are considered to be sensitive
and need to be enabled explicitly via configuration. If you are enabling
sensitive endpoints then you should also
secure the Data Flow server’s endpoints so that
information is not inadvertently exposed to unauthenticated users. The local Data Flow server has security disabled by default, so all actuator endpoints are available.
The Data Flow server requires a relational database and if the feature toggled for
analytics is enabled, a Redis server is also required. The Data Flow server will
autoconfigure the DataSourceHealthIndicator and RedisHealthIndicator if needed. The health of these two services is incorporated to the overall health status of the server through the health
endpoint.
18.1. Spring Boot Admin
A nice way to visualize and interact with actuator endpoints is to incorporate the Spring Boot Admin client library into the Spring Cloud Data Flow server. You can create the Spring Boot Admin application by following a few simple steps.
A simple way to have the Spring Cloud Data Flow server be a client to the Spring Boot Admin Server is by adding a dependency to the Data Flow server’s Maven pom.xml file and an additional configuration property as documented in Registering Client Applications. You will need to clone the github repository for the Spring Cloud Data Flow server in order to modify the Maven pom. There are tags in the repository for each release.
Adding this dependency will result in a UI with tabs for each of the actuator endpoints.
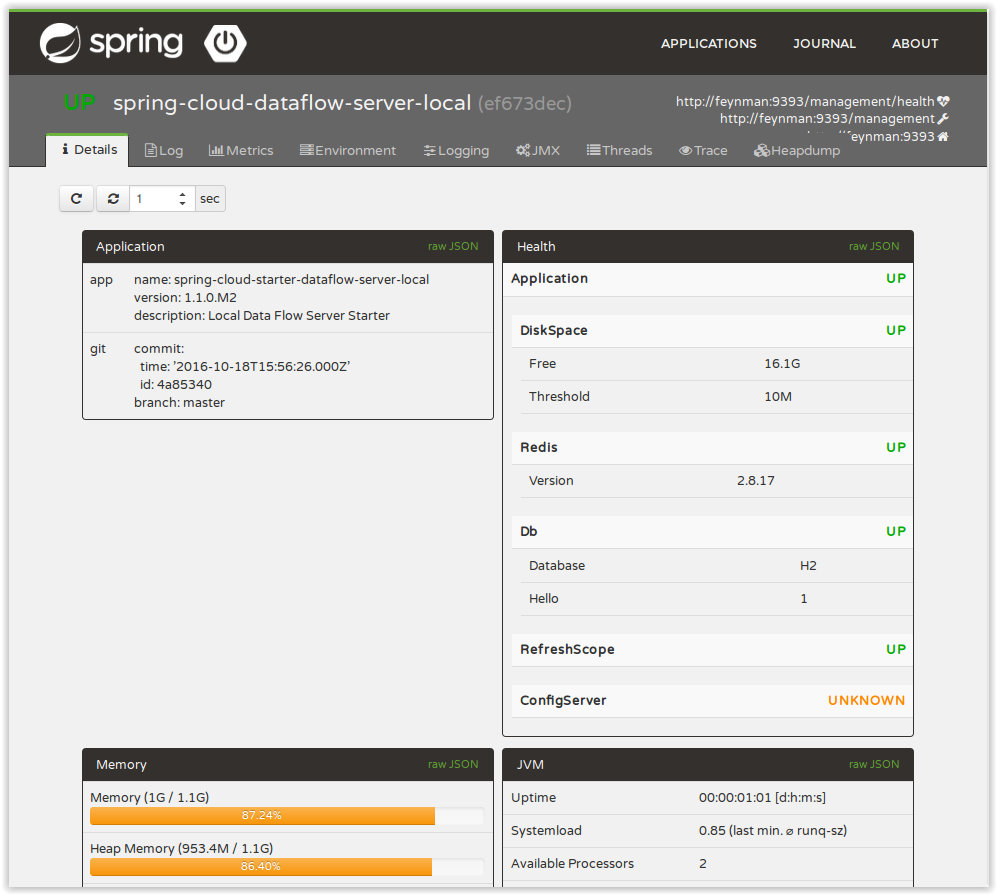
Additional configuration is required to interact with JMX beans and logging levels. Refer
to the Spring Boot admin documentation for more information. As only the info
and health
endpoints are available to unauthenticated users, you should enable security on
the Data Flow Server and also configure Spring Boot Admin server’s security so that it
can securely access the actuator endpoints.
18.2. Monitoring Deployed Applications
The applications that are deployed by Spring Cloud Data Flow are based on Spring Boot which contains several features for monitoring your application in production. Each deployed application contains several web endpoints for monitoring and interacting with Stream and Task applications.
In particular, the /metrics
endpoint contains counters
and gauges for HTTP requests, System Metrics (such as JVM stats), DataSource Metrics and Message Channel Metrics (such as message rates).
Spring Boot lets you add your own metrics to the /metrics
endpoint either by registering an implementation of the PublicMetrics
interface or through it’s integration with Dropwizard.
The Spring Boot interfaces MetricWriter
and Exporter
are used to send the
metrics data to a place where they can be displayed and analyzed. There are
implementations in Spring Boot to export metrics to Redis, Open TSDB, Statsd,
and JMX.
There are a few additional Spring projects that provide support for sending metrics data to external systems.
-
Spring Cloud Stream provides
ApplicationMetricsExporter
which publishes metrics via an Emitter to a messaging middleware destination. -
Spring Cloud Data Flow Metrics Collector subscribes to the metrics destination and aggregates metric messages published by the Spring Cloud Stream applications. It has an HTTP endpoint to access the aggregated metrics.
-
Spring Cloud Data Flow Metrics provides
LogMetricWriter
that writes to the log. -
Spring Cloud Data Flow Metrics Datadog Metrics provides
DatadogMetricWriter
that writes to Datadog.
The Spring Cloud Stream Emitter is used by the Spring Cloud Stream App Starters project that provides the most commonly used applications when creating Data Flow Streams.
The architecture when using Spring Cloud Stream’s Emitter
, the
Data Flow Metrics Collector, and the Data Flow server is shown below.
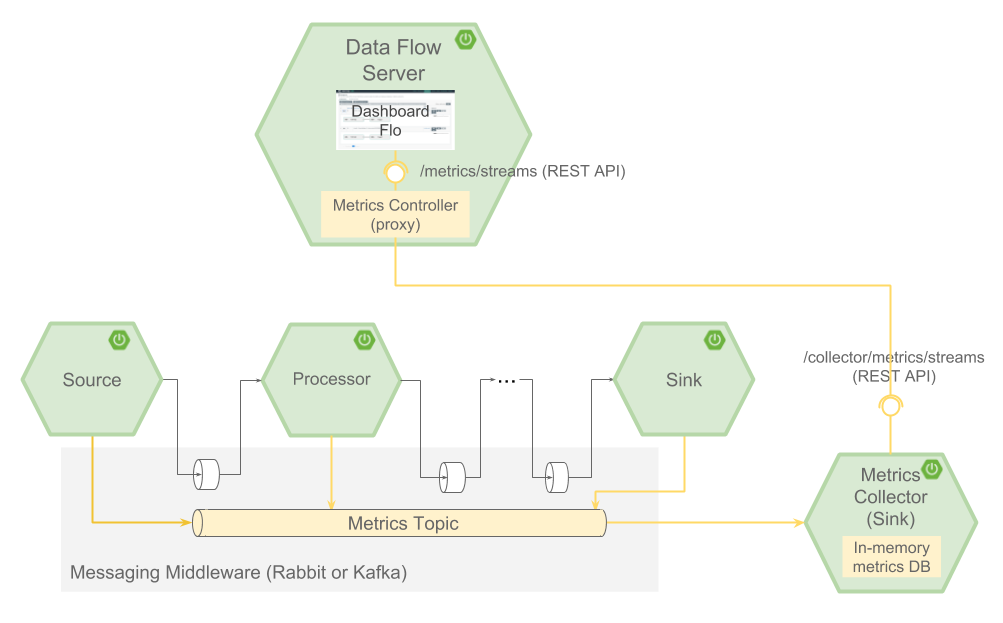
As with the App Starters, there is a Spring Boot uber jar artifact of the Metrics Collector for all of the supported binders. You can find more information on building and running the Metrics Collector on its project page.
The dataflow server now accepts an optional property spring.cloud.dataflow.metrics.collector.uri
, this property should point to the URI of your deployed
metrics collector app. For example, if you are running the metrics collector locally on port 8080
then start the server (local example) with the following command:
$ java -jar spring-cloud-dataflow-server-local-1.2.0.RC2.jar --spring.cloud.dataflow.metrics.collector.uri=http://localhost:8080
The Metrics Collector can be secured with 'basic' authentication that requires a username and password. To set the username and password, use the properties spring.cloud.dataflow.metrics.collector.username
and spring.cloud.dataflow.metrics.collector.password
.
The metrics
for each application are published when the property spring.cloud.stream.bindings.applicationMetrics.destination
is set. This can be set as any other
application property when deploying an application in Data Flow. Since
it is quite common to want all applications in a stream to emit metrics,
setting it at the Data Flow server level is a good way to achieve that.
spring.cloud.dataflow.applicationProperties.stream.spring.cloud.stream.bindings.applicationMetrics.destination=metrics
Using the destination name metrics
is a good choice as the Metrics
Collector subscribes to that name by default.
The next most common way to configure the metrics destination is using
deployment properties. Here is an example for the ticktock
stream that
uses the App Starters time
and log
applications.
app register --name time --type source --uri maven://org.springframework.cloud.stream.app:time-source-rabbit:1.2.0.RELEASE
app register --name log --type sink --uri maven://org.springframework.cloud.stream.app:log-sink-rabbit:1.2.0.RELEASE
stream create --name foostream --definition "time | log"
stream deploy --name foostream --properties "app.*.spring.cloud.stream.bindings.applicationMetrics.destination=metrics,deployer.*.count=2"
The Metrics Collector exposes aggregated metrics under the HTTP endpoint
/collector/metrics
in JSON format. The Data Flow server accesses this
endpoint in two distinct ways. The first is by exposing a /metrics/streams
HTTP endpoint that acts as a proxy to the Metrics Collector endpoint. This
is accessed by the UI when overlaying message rates on the Flo diagrams for
each stream. It is also accessed to enrich the Data Flow /runtime/apps
endpoint that is exposed in the UI via the Runtime
tab and in the shell
through the runtime apps
command with message rates.
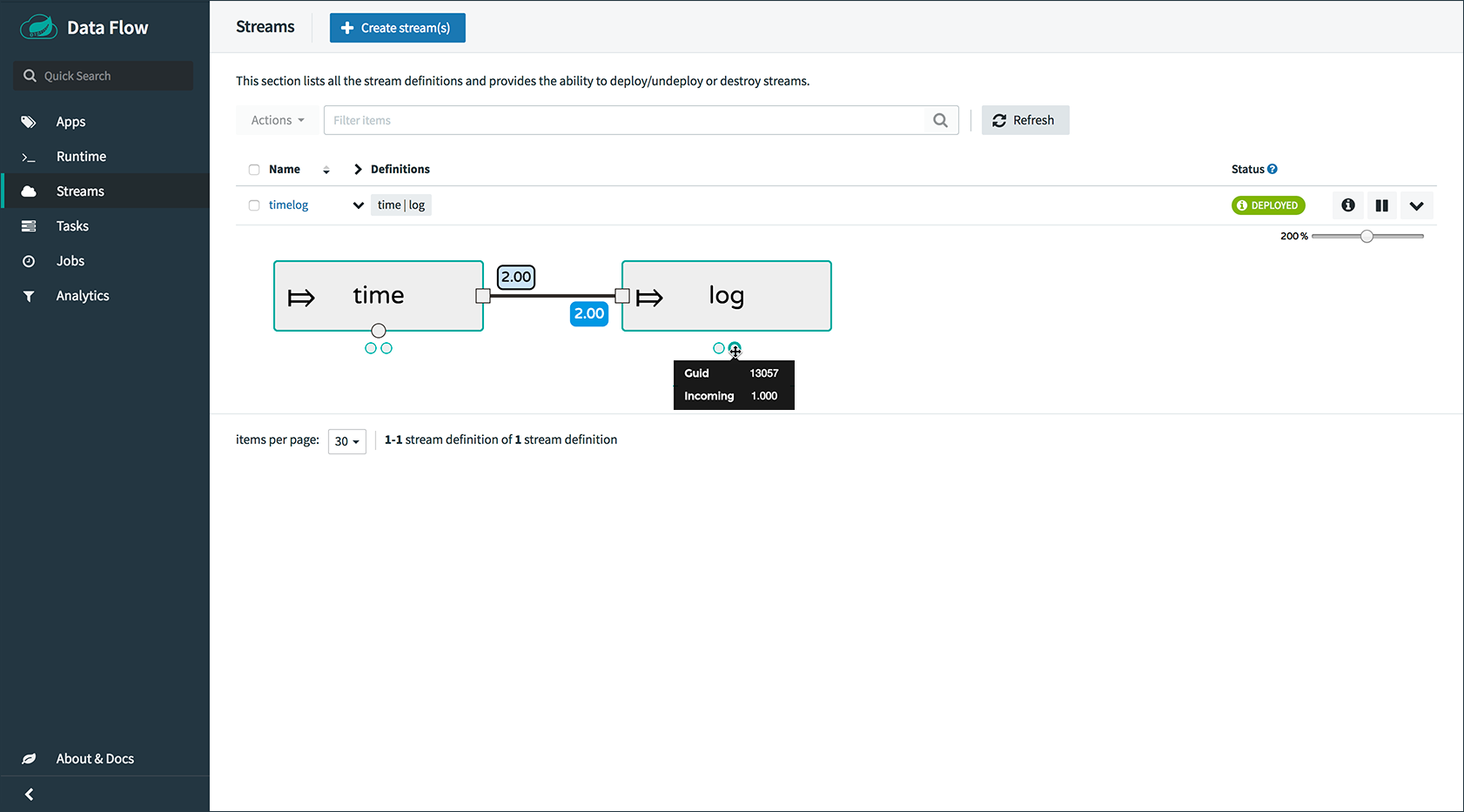
By default, Data Flow will set the property
spring.cloud.stream.metrics.properties=spring.application.name,spring.application.index,spring.cloud.application.*,spring.cloud.dataflow.*
Which is the set of application properties values needed to perform aggregation. It will also set the property
spring.metrics.export.triggers.application.includes=integration**`
since Data Flow will only display instantaneous input and output channel
message rates. By default, all metric values in the /metric
endpoint
are sent so restricting it reduces the size of the message payload without
impacting the functionality. Data Flow also exposes a guid
property when
displaying metric data which is used track back to the specific application
instance that generated the metric. The guid
value is platform dependent.
Note that you can override these defaults by setting then as you would any application property value.
Data Flow will not provide it’s own implementation to store and visualize historical metrics data. We will integrate with existing ALM system by providing an Exporter application that consumes messages from the same destination as the Metrics Collector and writes them to an existing ALM system. Which specific ALM system we will support is driven by user demand. However, to serve as an example, we will develop an Elastic Search exporter with a Grafana front end since it is open source.
18.3. Log and DataDog MetricWriter
If you prefer to have deployed applications bypass the centralized collection of metrics via the Metrics Collector, you can use the MetricWriters in Spring Cloud Data Flow Metrics and Spring Cloud Data Flow Metrics Datadog Metrics.
The Data Flow Metrics project provides the foundation for exporting Spring Boot
metrics via MetricWriters. It provides Spring Boots AutoConfiguration to setup
the writing process and common functionality such as defining a metric name
prefix appropriate for your environement. For example, you may want to
includes the region where the application is running in addition to the
application’s name and stream/task to which it belongs. It also includes a
LogMetricWriter
so that metrics can be stored into the log file. While very
simple in approach, log files are often ingested into application monitoring
tools (such as Splunk) where they can be further processed to create dashboards
of an application’s performance.
To make use of this functionality, you will need to add additional dependencies into your Stream and Task applications. To customize the "out of the box" Task and Stream applications you can use the Data Flow Initializr to generate a project and then add to the generated Maven pom file the MetricWriter implementation you want to use. The documentation on the Data Flow Metrics project pages provides the additional information you need to get started.
Streams
In this section you will learn all about Streams and how to use them with Spring Cloud Data Flow.
19. Introduction
In Spring Cloud Data Flow, a basic stream defines the ingestion of event data from a source to a sink that passes through any number of processors. Streams are composed of Spring Cloud Stream applications and the deployment of stream definitions is done via the Data Flow Server (REST API). The Getting Started section shows you how to start the server and how to start and use the Spring Cloud Data Flow shell.
A high level DSL is used to create stream definitions. The DSL to define a stream that has an http source and a file sink (with no processors) is shown below
http | file
The DSL mimics UNIX pipes and filters syntax. Default values for ports and filenames are used in this example but can be overridden using --
options, such as
http --server.port=8091 | file --directory=/tmp/httpdata/
To create these stream definitions you use the shell or make an HTTP POST request to the Spring Cloud Data Flow Server. For more information on making HTTP request directly to the server, consult the REST API Guide.
20. Stream DSL
In the example above, we connected a source to a sink using the pipe symbol |
. You can also pass properties to the source and sink configurations. The property names will depend on the individual app implementations, but as an example, the http
source app exposes a server.port
setting and it allows you to change the data ingestion port from the default value. To create the stream using port 8000, we would use
dataflow:> stream create --definition "http --server.port=8000 | log" --name myhttpstream
The shell provides tab completion for application properties and also the shell command app info <appType>:<appName>
provides additional documentation for all the supported properties.
Supported Stream <appType>'s are: source, processor, and sink |
21. Register a Stream App
Register a Stream App with the App Registry using the Spring Cloud Data Flow Shell
app register
command. You must provide a unique name, application type, and a URI that can be
resolved to the app artifact. For the type, specify "source", "processor", or "sink".
Here are a few examples:
dataflow:>app register --name mysource --type source --uri maven://com.example:mysource:0.0.1-SNAPSHOT
dataflow:>app register --name myprocessor --type processor --uri file:///Users/example/myprocessor-1.2.3.jar
dataflow:>app register --name mysink --type sink --uri http://example.com/mysink-2.0.1.jar
When providing a URI with the maven
scheme, the format should conform to the following:
maven://<groupId>:<artifactId>[:<extension>[:<classifier>]]:<version>
For example, if you would like to register the snapshot versions of the http
and log
applications built with the RabbitMQ binder, you could do the following:
dataflow:>app register --name http --type source --uri maven://org.springframework.cloud.stream.app:http-source-rabbit:1.2.1.BUILD-SNAPSHOT
dataflow:>app register --name log --type sink --uri maven://org.springframework.cloud.stream.app:log-sink-rabbit:1.2.1.BUILD-SNAPSHOT
If you would like to register multiple apps at one time, you can store them in a properties file
where the keys are formatted as <type>.<name>
and the values are the URIs.
For example, if you would like to register the snapshot versions of the http
and log
applications built with the RabbitMQ binder, you could have the following in a properties file [eg: stream-apps.properties]:
source.http=maven://org.springframework.cloud.stream.app:http-source-rabbit:1.2.1.BUILD-SNAPSHOT
sink.log=maven://org.springframework.cloud.stream.app:log-sink-rabbit:1.2.1.BUILD-SNAPSHOT
Then to import the apps in bulk, use the app import
command and provide the location of the properties file via --uri
:
dataflow:>app import --uri file:///<YOUR_FILE_LOCATION>/stream-apps.properties
For convenience, we have the static files with application-URIs (for both maven and docker) available for all the out-of-the-box stream and task/batch app-starters. You can point to this file and import all the application-URIs in bulk. Otherwise, as explained in previous paragraphs, you can register them individually or have your own custom property file with only the required application-URIs in it. It is recommended, however, to have a "focused" list of desired application-URIs in a custom property file.
List of available Stream Application Starters:
Artifact Type | Stable Release | SNAPSHOT Release |
---|---|---|
RabbitMQ + Maven |
bit.ly/Bacon-BUILD-SNAPSHOT-stream-applications-rabbit-maven |
|
RabbitMQ + Docker |
N/A |
|
Kafka 0.9 + Maven |
bit.ly/Bacon-BUILD-SNAPSHOT-stream-applications-kafka-09-maven |
|
Kafka 0.9 + Docker |
N/A |
|
Kafka 0.10 + Maven |
bit.ly/Bacon-BUILD-SNAPSHOT-stream-applications-kafka-10-maven |
|
Kafka 0.10 + Docker |
N/A |
List of available Task Application Starters:
Artifact Type | Stable Release | SNAPSHOT Release |
---|---|---|
Maven |
||
Docker |
N/A |
You can find more information about the available task starters in the Task App Starters Project Page and related reference documentation. For more information about the available stream starters look at the Stream App Starters Project Page and related reference documentation.
As an example, if you would like to register all out-of-the-box stream applications built with the RabbitMQ binder in bulk, you can with the following command.
dataflow:>app import --uri http://bit.ly/Bacon-RELEASE-stream-applications-rabbit-maven
You can also pass the --local
option (which is true
by default) to indicate whether the
properties file location should be resolved within the shell process itself. If the location should
be resolved from the Data Flow Server process, specify --local false
.
When using either Note however that once downloaded, applications may be cached locally on the Data Flow server, based on the resource
location. If the resource location doesn’t change (even though the actual resource bytes may be different), then it
won’t be re-downloaded. When using Moreover, if a stream is already deployed and using some version of a registered app, then (forcibly) re-registering a different app will have no effect until the stream is deployed anew. |
In some cases the Resource is resolved on the server side, whereas in others the URI will be passed to a runtime container instance where it is resolved. Consult the specific documentation of each Data Flow Server for more detail. |
21.1. Whitelisting application properties
Stream and Task applications are Spring Boot applications which are aware of many Common application properties, e.g. server.port
but also families of properties such as those with the prefix spring.jmx
and logging
. When creating your own application it is desirable to whitelist properties so that the shell and the UI can display them first as primary properties when presenting options via TAB completion or in drop-down boxes.
To whitelist application properties create a file named spring-configuration-metadata-whitelist.properties
in the META-INF
resource directory. There are two property keys that can be used inside this file. The first key is named configuration-properties.classes
. The value is a comma separated list of fully qualified @ConfigurationProperty
class names. The second key is configuration-properties.names
whose value is a comma separated list of property names. This can contain the full name of property, such as server.port
or a partial name to whitelist a category of property names, e.g. spring.jmx
.
The Spring Cloud Stream application starters are a good place to look for examples of usage. Here is a simple example of the file sink’s spring-configuration-metadata-whitelist.properties
file
configuration-properties.classes=org.springframework.cloud.stream.app.file.sink.FileSinkProperties
If we also wanted to add server.port
to be white listed, then it would look like this:
configuration-properties.classes=org.springframework.cloud.stream.app.file.sink.FileSinkProperties
configuration-properties.names=server.port
Make sure to add 'spring-boot-configuration-processor' as an optional dependency to generate configuration metadata file for the properties.
|
21.2. Creating and using a dedicated metadata artifact
You can go a step further in the process of describing the main properties that your stream or task app supports by creating a so-called metadata companion artifact. This simple jar file contains only the Spring boot JSON file about configuration properties metadata, as well as the whitelisting file described in the previous section.
Here is the contents of such an artifact, for the canonical log
sink:
$ jar tvf log-sink-rabbit-1.2.1.BUILD-SNAPSHOT-metadata.jar
373848 META-INF/spring-configuration-metadata.json
174 META-INF/spring-configuration-metadata-whitelist.properties
Note that the spring-configuration-metadata.json
file is quite large. This is because it contains the concatenation of all the properties that
are available at runtime to the log
sink (some of them come from spring-boot-actuator.jar
, some of them come from
spring-boot-autoconfigure.jar
, even some more from spring-cloud-starter-stream-sink-log.jar
, etc.) Data Flow
always relies on all those properties, even when a companion artifact is not available, but here all have been merged
into a single file.
To help with that (as a matter of fact, you don’t want to try to craft this giant JSON file by hand), you can use the following plugin in your build:
<plugin>
<groupId>org.springframework.cloud</groupId>
<artifactId>spring-cloud-app-starter-metadata-maven-plugin</artifactId>
<executions>
<execution>
<id>aggregate-metadata</id>
<phase>compile</phase>
<goals>
<goal>aggregate-metadata</goal>
</goals>
</execution>
</executions>
</plugin>
This plugin comes in addition to the spring-boot-configuration-processor that creates the individual JSON files.
Be sure to configure the two!
|
The benefits of a companion artifact are manifold:
-
being way lighter (usually a few kilobytes, as opposed to megabytes for the actual app), they are quicker to download, allowing quicker feedback when using e.g.
app info
or the Dashboard UI -
as a consequence of the above, they can be used in resource constrained environments (such as PaaS) when metadata is the only piece of information needed
-
finally, for environments that don’t deal with boot uberjars directly (for example, Docker-based runtimes such as Kubernetes or Mesos), this is the only way to provide metadata about the properties supported by the app.
Remember though, that this is entirely optional when dealing with uberjars. The uberjar itself also includes the metadata in it already.
21.2.1. Using the companion artifact
Once you have a companion artifact at hand, you need to make the system aware of it so that it can be used.
When registering a single app via app register
, you can use the optional --metadata-uri
option in the shell, like so:
dataflow:>app register --name log --type sink
--uri maven://org.springframework.cloud.stream.app:log-sink-kafka-10:1.2.1.BUILD-SNAPSHOT
--metadata-uri=maven://org.springframework.cloud.stream.app:log-sink-kafka-10:jar:metadata:1.2.1.BUILD-SNAPSHOT
When registering several files using the app import
command, the file should contain a <type>.<name>.metadata
line
in addition to each <type>.<name>
line. This is optional (i.e. if some apps have it but some others don’t, that’s fine).
Here is an example for a Dockerized app, where the metadata artifact is being hosted in a Maven repository (but retrieving
it via http://
or file://
would be equally possible).
...
source.http=docker:springcloudstream/http-source-rabbit:latest
source.http.metadata=maven://org.springframework.cloud.stream.app:http-source-rabbit:jar:metadata:1.2.1.BUILD-SNAPSHOT
...
22. Creating custom applications
While there are out of the box source, processor, sink applications available, one can extend these applications or write a custom Spring Cloud Stream application.
The process of creating Spring Cloud Stream applications via Spring Initializr is detailed in the Spring Cloud Stream documentation. It is possible to include multiple binders to an application. If doing so, refer the instructions in Passing Spring Cloud Stream properties for the application on how to configure them.
For supporting property whitelisting, Spring Cloud Stream applications running in Spring Cloud Data Flow may include the Spring Boot configuration-processor
as an optional dependency, as in the following example.
<dependencies>
<!-- other dependencies -->
<dependency>
<groupId>org.springframework.boot</groupId>
<artifactId>spring-boot-configuration-processor</artifactId>
<optional>true</optional>
</dependency>
</dependencies>
Make sure that the |
Once a custom application has been created, it can be registered as described in Register a Stream App.
23. Creating a Stream
The Spring Cloud Data Flow Server exposes a full RESTful API for managing the lifecycle of stream definitions, but the easiest way to use is it is via the Spring Cloud Data Flow shell. Start the shell as described in the Getting Started section.
New streams are created by with the help of stream definitions. The definitions are built from a simple DSL. For example, let’s walk through what happens if we execute the following shell command:
dataflow:> stream create --definition "time | log" --name ticktock
This defines a stream named ticktock
based off the DSL expression time | log
. The DSL uses the "pipe" symbol |
, to connect a source to a sink.
Then to deploy the stream execute the following shell command (or alternatively add the --deploy
flag when creating the stream so that this step is not needed):
dataflow:> stream deploy --name ticktock
The Data Flow Server resolves time
and log
to maven coordinates and uses those to launch the time
and log
applications of the stream.
2016-06-01 09:41:21.728 INFO 79016 --- [nio-9393-exec-6] o.s.c.d.spi.local.LocalAppDeployer : deploying app ticktock.log instance 0
Logs will be in /var/folders/wn/8jxm_tbd1vj28c8vj37n900m0000gn/T/spring-cloud-dataflow-912434582726479179/ticktock-1464788481708/ticktock.log
2016-06-01 09:41:21.914 INFO 79016 --- [nio-9393-exec-6] o.s.c.d.spi.local.LocalAppDeployer : deploying app ticktock.time instance 0
Logs will be in /var/folders/wn/8jxm_tbd1vj28c8vj37n900m0000gn/T/spring-cloud-dataflow-912434582726479179/ticktock-1464788481910/ticktock.time
In this example, the time source simply sends the current time as a message each second, and the log sink outputs it using the logging framework.
You can tail the stdout
log (which has an "_<instance>" suffix). The log files are located within the directory displayed in the Data Flow Server’s log output, as shown above.
$ tail -f /var/folders/wn/8jxm_tbd1vj28c8vj37n900m0000gn/T/spring-cloud-dataflow-912434582726479179/ticktock-1464788481708/ticktock.log/stdout_0.log
2016-06-01 09:45:11.250 INFO 79194 --- [ kafka-binder-] log.sink : 06/01/16 09:45:11
2016-06-01 09:45:12.250 INFO 79194 --- [ kafka-binder-] log.sink : 06/01/16 09:45:12
2016-06-01 09:45:13.251 INFO 79194 --- [ kafka-binder-] log.sink : 06/01/16 09:45:13
23.1. Application properties
Application properties are the properties associated with each application in the stream. When the application is deployed, the application properties are applied to the application via command line arguments or environment variables based on the underlying deployment implementation.
23.1.1. Passing application properties when creating a stream
The following stream
dataflow:> stream create --definition "time | log" --name ticktock
can have application properties defined at the time of stream creation.
The shell command app info <appType>:<appName>
displays the white-listed application properties for the application.
For more info on the property white listing refer to Whitelisting application properties
Below are the white listed properties for the app time
:
dataflow:> app info source:time
╔══════════════════════════════╤══════════════════════════════╤══════════════════════════════╤══════════════════════════════╗
║ Option Name │ Description │ Default │ Type ║
╠══════════════════════════════╪══════════════════════════════╪══════════════════════════════╪══════════════════════════════╣
║trigger.time-unit │The TimeUnit to apply to delay│<none> │java.util.concurrent.TimeUnit ║
║ │values. │ │ ║
║trigger.fixed-delay │Fixed delay for periodic │1 │java.lang.Integer ║
║ │triggers. │ │ ║
║trigger.cron │Cron expression value for the │<none> │java.lang.String ║
║ │Cron Trigger. │ │ ║
║trigger.initial-delay │Initial delay for periodic │0 │java.lang.Integer ║
║ │triggers. │ │ ║
║trigger.max-messages │Maximum messages per poll, -1 │1 │java.lang.Long ║
║ │means infinity. │ │ ║
║trigger.date-format │Format for the date value. │<none> │java.lang.String ║
╚══════════════════════════════╧══════════════════════════════╧══════════════════════════════╧══════════════════════════════╝
Below are the white listed properties for the app log
:
dataflow:> app info sink:log
╔══════════════════════════════╤══════════════════════════════╤══════════════════════════════╤══════════════════════════════╗
║ Option Name │ Description │ Default │ Type ║
╠══════════════════════════════╪══════════════════════════════╪══════════════════════════════╪══════════════════════════════╣
║log.name │The name of the logger to use.│<none> │java.lang.String ║
║log.level │The level at which to log │<none> │org.springframework.integratio║
║ │messages. │ │n.handler.LoggingHandler$Level║
║log.expression │A SpEL expression (against the│payload │java.lang.String ║
║ │incoming message) to evaluate │ │ ║
║ │as the logged message. │ │ ║
╚══════════════════════════════╧══════════════════════════════╧══════════════════════════════╧══════════════════════════════╝
The application properties for the time
and log
apps can be specified at the time of stream
creation as follows:
dataflow:> stream create --definition "time --fixed-delay=5 | log --level=WARN" --name ticktock
Note that the properties fixed-delay
and level
defined above for the apps time
and log
are the 'short-form' property names provided by the shell completion.
These 'short-form' property names are applicable only for the white-listed properties and in all other cases, only fully qualified property names should be used.
23.2. Deployment properties
When deploying the stream, properties that control the deployment of the apps into the target platform are known as deployment
properties.
For instance, one can specify how many instances need to be deployed for the specific application defined in the stream using the deployment property called count
.
23.2.1. Application properties versus Deployer properties
Starting with version 1.2, the distinction between properties that are meant for the deployed app and properties that
govern how this app is deployed (thanks to some implementation of a
spring cloud deployer) is more explicit. The former should be
passed using the syntax app.<app-name>.<property-name>=<value>
while the latter use the
deployer.<app-name>.<short-property-name>=<value>
The following table recaps the difference in behavior between the two.
Application Properties | Deployer Properties | |
---|---|---|
Example Syntax |
|
|
What the application "sees" |
|
Nothing |
What the deployer "sees" |
Nothing |
|
Typical usage |
Passing/Overriding application properties, passing Spring Cloud Stream binder or partitionning properties |
Setting the number of instances, memory, disk, etc. |
23.2.2. Passing instance count as deployment property
If you would like to have multiple instances of an application in the stream, you can include a deployer property with the deploy command:
dataflow:> stream deploy --name ticktock --properties "deployer.time.count=3"
Note that count
is the reserved property name used by the underlying deployer. Hence, if the application also has a custom property named count
, it is not supported
when specified in 'short-form' form during stream deployment as it could conflict with the instance count deployer property. Instead, the count
as a custom application property can be
specified in its fully qualified form (example: app.foo.bar.count
) during stream deployment or it can be specified using 'short-form' or fully qualified form during the stream creation
where it will be considered as an app property.
23.2.3. Inline vs file reference properties
When using the Spring Cloud Data Flow Shell, there are two ways to provide deployment properties: either inline or via a file reference. Those two ways are exclusive and documented below:
- Inline properties
-
use the
--properties
shell option and list properties as a comma separated list of key=value pairs, like so:
stream deploy foo
--properties "deployer.transform.count=2,app.transform.producer.partitionKeyExpression=payload"
- Using a file reference
-
use the
--propertiesFile
option and point it to a local.properties
,.yaml
or.yml
file (i.e. that lives in the filesystem of the machine running the shell). Being read as a.properties
file, normal rules apply (ISO 8859-1 encoding,=
,<space>
or:
delimiter, etc.) although we recommend using=
as a key-value pair delimiter for consistency:
stream deploy foo --propertiesFile myprops.properties
where myprops.properties
contains:
deployer.transform.count=2
app.transform.producer.partitionKeyExpression=payload
Both the above properties will be passed as deployment properties for the stream foo
above.
In case of using YAML as the format for the deployment properties, use the .yaml
or .yml
file extention when deploying the stream,
stream deploy foo --propertiesFile myprops.yaml
where myprops.yaml
contains:
deployer:
transform:
count: 2
app:
transform:
producer:
partitionKeyExpression: payload
23.2.4. Passing application properties when deploying a stream
The application properties can also be specified when deploying a stream. When specified during deployment, these application properties can either be specified as 'short-form' property names (applicable for white-listed properties) or fully qualified property names. The application properties should have the prefix "app.<appName/label>".
For example, the stream
dataflow:> stream create --definition "time | log" --name ticktock
can be deployed with application properties using the 'short-form' property names:
dataflow:>stream deploy ticktock --properties "app.time.fixed-delay=5,app.log.level=ERROR"
When using the app label,
stream create ticktock --definition "a: time | b: log"
the application properties can be defined as:
stream deploy ticktock --properties "app.a.fixed-delay=4,app.b.level=ERROR"
23.2.5. Passing Spring Cloud Stream properties for the application
Spring Cloud Data Flow sets the required
Spring Cloud Stream properties for the applications inside the stream. Most importantly, the spring.cloud.stream.bindings.<input/output>.destination
is set internally for the apps to bind.
If someone wants to override any of the Spring Cloud Stream properties, they can be set via deployment properties.
For example, for the below stream
dataflow:> stream create --definition "http | transform --expression=payload.getValue('hello').toUpperCase() | log" --name ticktock
if there are multiple binders available in the classpath for each of the applications and the binder is chosen for each deployment then the stream can be deployed with the specific Spring Cloud Stream properties as:
dataflow:>stream deploy ticktock --properties "app.time.spring.cloud.stream.bindings.output.binder=kafka,app.transform.spring.cloud.stream.bindings.input.binder=kafka,app.transform.spring.cloud.stream.bindings.output.binder=rabbit,app.log.spring.cloud.stream.bindings.input.binder=rabbit"
Overriding the destination names is not recommended as Spring Cloud Data Flow takes care of setting this internally. |
23.2.6. Passing per-binding producer consumer properties
A Spring Cloud Stream application can have producer and consumer properties set per-binding
basis.
While Spring Cloud Data Flow supports specifying short-hand notation for per binding producer properties such as partitionKeyExpression
, partitionKeyExtractorClass
as described in Passing stream partition properties during stream deployment, all the supported Spring Cloud Stream producer/consumer properties can be set as Spring Cloud Stream properties for the app directly as well.
The consumer properties can be set for the inbound
channel name with the prefix app.[app/label name].spring.cloud.stream.bindings.<channelName>.consumer.
and the producer properties can be set for the outbound
channel name with the prefix app.[app/label name].spring.cloud.stream.bindings.<channelName>.producer.
.
For example, the stream
dataflow:> stream create --definition "time | log" --name ticktock
can be deployed with producer/consumer properties as:
dataflow:>stream deploy ticktock --properties "app.time.spring.cloud.stream.bindings.output.producer.requiredGroups=myGroup,app.time.spring.cloud.stream.bindings.output.producer.headerMode=raw,app.log.spring.cloud.stream.bindings.input.consumer.concurrency=3,app.log.spring.cloud.stream.bindings.input.consumer.maxAttempts=5"
The binder
specific producer/consumer properties can also be specified in a similar way.
For instance
dataflow:>stream deploy ticktock --properties "app.time.spring.cloud.stream.rabbit.bindings.output.producer.autoBindDlq=true,app.log.spring.cloud.stream.rabbit.bindings.input.consumer.transacted=true"
23.2.7. Passing stream partition properties during stream deployment
A common pattern in stream processing is to partition the data as it is streamed. This entails deploying multiple instances of a message consuming app and using content-based routing so that messages with a given key (as determined at runtime) are always routed to the same app instance. You can pass the partition properties during stream deployment to declaratively configure a partitioning strategy to route each message to a specific consumer instance.
See below for examples of deploying partitioned streams:
- app.[app/label name].producer.partitionKeyExtractorClass
-
The class name of a PartitionKeyExtractorStrategy (default
null
) - app.[app/label name].producer.partitionKeyExpression
-
A SpEL expression, evaluated against the message, to determine the partition key; only applies if
partitionKeyExtractorClass
is null. If both are null, the app is not partitioned (defaultnull
) - app.[app/label name].producer.partitionSelectorClass
-
The class name of a PartitionSelectorStrategy (default
null
) - app.[app/label name].producer.partitionSelectorExpression
-
A SpEL expression, evaluated against the partition key, to determine the partition index to which the message will be routed. The final partition index will be the return value (an integer) modulo
[nextModule].count
. If both the class and expression are null, the underlying binder’s default PartitionSelectorStrategy will be applied to the key (defaultnull
)
In summary, an app is partitioned if its count is > 1 and the previous app has a
partitionKeyExtractorClass
or partitionKeyExpression
(class takes precedence).
When a partition key is extracted, the partitioned app instance is determined by
invoking the partitionSelectorClass
, if present, or the partitionSelectorExpression % partitionCount
,
where partitionCount
is application count in the case of RabbitMQ, and the underlying
partition count of the topic in the case of Kafka.
If neither a partitionSelectorClass
nor a partitionSelectorExpression
is
present the result is key.hashCode() % partitionCount
.
23.2.8. Passing application content type properties
In a stream definition you can specify that the input or the output of an application need to be converted to a different type.
You can use the inputType
and outputType
properties to specify the content type for the incoming data and outgoing data, respectively.
For example, consider the following stream:
dataflow:>stream create tuple --definition "http | filter --inputType=application/x-spring-tuple
--expression=payload.hasFieldName('hello') | transform --expression=payload.getValue('hello').toUpperCase()
| log" --deploy
The http
app is expected to send the data in JSON and the filter
app receives the JSON data
and processes it as a Spring Tuple.
In order to do so, we use the inputType
property on the filter app to convert the data into the expected Spring Tuple format.
The transform
application processes the Tuple data and sends the processed data to the downstream log
application.
When sending some data to the http
application:
dataflow:>http post --data {"hello":"world","foo":"bar"} --contentType application/json --target http://localhost:<http-port>
At the log application you see the content as follows:
INFO 18745 --- [transform.tuple-1] log.sink : WORLD
Depending on how applications are chained, the content type conversion can be specified either as via the --outputType
in the upstream app or as an --inputType
in the downstream app.
For instance, in the above stream, instead of specifying the --inputType
on the 'transform' application to convert, the option --outputType=application/x-spring-tuple
can also be specified on the 'http' application.
For the complete list of message conversion and message converters, please refer to Spring Cloud Stream documentation.
23.2.9. Overriding application properties during stream deployment
Application properties that are defined during deployment override the same properties defined during the stream creation.
For example, the following stream has application properties defined during stream creation:
dataflow:> stream create --definition "time --fixed-delay=5 | log --level=WARN" --name ticktock
To override these application properties, one can specify the new property values during deployment:
dataflow:>stream deploy ticktock --properties "app.time.fixed-delay=4,app.log.level=ERROR"
23.3. Common application properties
In addition to configuration via DSL, Spring Cloud Data Flow provides a mechanism for setting common properties to all
the streaming applications that are launched by it.
This can be done by adding properties prefixed with spring.cloud.dataflow.applicationProperties.stream
when starting
the server.
When doing so, the server will pass all the properties, without the prefix, to the instances it launches.
For example, all the launched applications can be configured to use a specific Kafka broker by launching the Data Flow server with the following options:
--spring.cloud.dataflow.applicationProperties.stream.spring.cloud.stream.kafka.binder.brokers=192.168.1.100:9092
--spring.cloud.dataflow.applicationProperties.stream.spring.cloud.stream.kafka.binder.zkNodes=192.168.1.100:2181
This will cause the properties spring.cloud.stream.kafka.binder.brokers
and spring.cloud.stream.kafka.binder.zkNodes
to be passed to all the launched applications.
Properties configured using this mechanism have lower precedence than stream deployment properties.
They will be overridden if a property with the same key is specified at stream deployment time (e.g.
app.http.spring.cloud.stream.kafka.binder.brokers will override the common property).
|
24. Destroying a Stream
You can delete a stream by issuing the stream destroy
command from the shell:
dataflow:> stream destroy --name ticktock
If the stream was deployed, it will be undeployed before the stream definition is deleted.
25. Deploying and Undeploying Streams
Often you will want to stop a stream, but retain the name and definition for future use. In that case you can undeploy
the stream by name and issue the deploy
command at a later time to restart it.
dataflow:> stream undeploy --name ticktock
dataflow:> stream deploy --name ticktock
26. Other Source and Sink Application Types
Let’s try something a bit more complicated and swap out the time
source for something else. Another supported source type is http
, which accepts data for ingestion over HTTP POSTs. Note that the http
source accepts data on a different port from the Data Flow Server (default 8080). By default the port is randomly assigned.
To create a stream using an http
source, but still using the same log
sink, we would change the original command above to
dataflow:> stream create --definition "http | log" --name myhttpstream --deploy
which will produce the following output from the server
2016-06-01 09:47:58.920 INFO 79016 --- [io-9393-exec-10] o.s.c.d.spi.local.LocalAppDeployer : deploying app myhttpstream.log instance 0
Logs will be in /var/folders/wn/8jxm_tbd1vj28c8vj37n900m0000gn/T/spring-cloud-dataflow-912434582726479179/myhttpstream-1464788878747/myhttpstream.log
2016-06-01 09:48:06.396 INFO 79016 --- [io-9393-exec-10] o.s.c.d.spi.local.LocalAppDeployer : deploying app myhttpstream.http instance 0
Logs will be in /var/folders/wn/8jxm_tbd1vj28c8vj37n900m0000gn/T/spring-cloud-dataflow-912434582726479179/myhttpstream-1464788886383/myhttpstream.http
Note that we don’t see any other output this time until we actually post some data (using a shell command). In order to see the randomly assigned port on which the http source is listening, execute:
dataflow:> runtime apps
You should see that the corresponding http source has a url
property containing the host and port information on which it is listening. You are now ready to post to that url, e.g.:
dataflow:> http post --target http://localhost:1234 --data "hello"
dataflow:> http post --target http://localhost:1234 --data "goodbye"
and the stream will then funnel the data from the http source to the output log implemented by the log sink
2016-06-01 09:50:22.121 INFO 79654 --- [ kafka-binder-] log.sink : hello
2016-06-01 09:50:26.810 INFO 79654 --- [ kafka-binder-] log.sink : goodbye
Of course, we could also change the sink implementation. You could pipe the output to a file (file
), to hadoop (hdfs
) or to any of the other sink apps which are available. You can also define your own apps.
27. Simple Stream Processing
As an example of a simple processing step, we can transform the payload of the HTTP posted data to upper case using the stream definitions
http | transform --expression=payload.toUpperCase() | log
To create this stream enter the following command in the shell
dataflow:> stream create --definition "http | transform --expression=payload.toUpperCase() | log" --name mystream --deploy
Posting some data (using a shell command)
dataflow:> http post --target http://localhost:1234 --data "hello"
Will result in an uppercased 'HELLO' in the log
2016-06-01 09:54:37.749 INFO 80083 --- [ kafka-binder-] log.sink : HELLO
28. Stateful Stream Processing
To demonstrate the data partitioning functionality, let’s deploy the following stream with Kafka as the binder.
dataflow:>stream create --name words --definition "http --server.port=9900 | splitter --expression=payload.split(' ') | log"
Created new stream 'words'
dataflow:>stream deploy words --properties "app.splitter.producer.partitionKeyExpression=payload,deployer.log.count=2"
Deployed stream 'words'
dataflow:>http post --target http://localhost:9900 --data "How much wood would a woodchuck chuck if a woodchuck could chuck wood"
> POST (text/plain;Charset=UTF-8) http://localhost:9900 How much wood would a woodchuck chuck if a woodchuck could chuck wood
> 202 ACCEPTED
You’ll see the following in the server logs.
2016-06-05 18:33:24.982 INFO 58039 --- [nio-9393-exec-9] o.s.c.d.spi.local.LocalAppDeployer : deploying app words.log instance 0
Logs will be in /var/folders/c3/ctx7_rns6x30tq7rb76wzqwr0000gp/T/spring-cloud-dataflow-694182453710731989/words-1465176804970/words.log
2016-06-05 18:33:24.988 INFO 58039 --- [nio-9393-exec-9] o.s.c.d.spi.local.LocalAppDeployer : deploying app words.log instance 1
Logs will be in /var/folders/c3/ctx7_rns6x30tq7rb76wzqwr0000gp/T/spring-cloud-dataflow-694182453710731989/words-1465176804970/words.log
Review the words.log instance 0
logs:
2016-06-05 18:35:47.047 INFO 58638 --- [ kafka-binder-] log.sink : How
2016-06-05 18:35:47.066 INFO 58638 --- [ kafka-binder-] log.sink : chuck
2016-06-05 18:35:47.066 INFO 58638 --- [ kafka-binder-] log.sink : chuck
Review the words.log instance 1
logs:
2016-06-05 18:35:47.047 INFO 58639 --- [ kafka-binder-] log.sink : much
2016-06-05 18:35:47.066 INFO 58639 --- [ kafka-binder-] log.sink : wood
2016-06-05 18:35:47.066 INFO 58639 --- [ kafka-binder-] log.sink : would
2016-06-05 18:35:47.066 INFO 58639 --- [ kafka-binder-] log.sink : a
2016-06-05 18:35:47.066 INFO 58639 --- [ kafka-binder-] log.sink : woodchuck
2016-06-05 18:35:47.067 INFO 58639 --- [ kafka-binder-] log.sink : if
2016-06-05 18:35:47.067 INFO 58639 --- [ kafka-binder-] log.sink : a
2016-06-05 18:35:47.067 INFO 58639 --- [ kafka-binder-] log.sink : woodchuck
2016-06-05 18:35:47.067 INFO 58639 --- [ kafka-binder-] log.sink : could
2016-06-05 18:35:47.067 INFO 58639 --- [ kafka-binder-] log.sink : wood
This shows that payload splits that contain the same word are routed to the same application instance.
29. Tap a Stream
Taps can be created at various producer endpoints in a stream. For a stream like this:
stream create --definition "http | step1: transform --expression=payload.toUpperCase() | step2: transform --expression=payload+'!' | log" --name mainstream --deploy
taps can be created at the output of http
, step1
and step2
.
To create a stream that acts as a 'tap' on another stream requires to specify the source destination name
for the tap stream. The syntax for source destination name is:
`:<streamName>.<label/appName>`
To create a tap at the output of http
in the stream above, the source destination name is mainstream.http
To create a tap at the output of the first transform app in the stream above, the source destination name is mainstream.step1
The tap stream DSL looks like this:
stream create --definition ":mainstream.http > counter" --name tap_at_http --deploy
stream create --definition ":mainstream.step1 > jdbc" --name tap_at_step1_transformer --deploy
Note the colon (:) prefix before the destination names. The colon allows the parser to recognize this as a destination name instead of an app name.
30. Using Labels in a Stream
When a stream is comprised of multiple apps with the same name, they must be qualified with labels:
stream create --definition "http | firstLabel: transform --expression=payload.toUpperCase() | secondLabel: transform --expression=payload+'!' | log" --name myStreamWithLabels --deploy
31. Explicit Broker Destinations in a Stream
One can connect to a specific destination name located in the broker (Rabbit, Kafka etc.,) either at the source
or at the sink
position.
The following stream has the destination name at the source
position:
stream create --definition ":myDestination > log" --name ingest_from_broker --deploy
This stream receives messages from the destination myDestination
located at the broker and connects it to the log
app.
The following stream has the destination name at the sink
position:
stream create --definition "http > :myDestination" --name ingest_to_broker --deploy
This stream sends the messages from the http
app to the destination myDestination
located at the broker.
From the above streams, notice that the http
and log
apps are interacting with each other via the broker (through the destination myDestination
) rather than having a pipe directly between http
and log
within a single stream.
It is also possible to connect two different destinations (source
and sink
positions) at the broker in a stream.
stream create --definition ":destination1 > :destination2" --name bridge_destinations --deploy
In the above stream, both the destinations (destination1
and destination2
) are located in the broker. The messages flow from the source destination to the sink destination via a bridge
app that connects them.
32. Directed Graphs in a Stream
If directed graphs are needed instead of the simple linear streams described above, two features are relevant.
First, named destinations may be used as a way to combine the output from multiple streams or for multiple consumers to share the output from a single stream.
This can be done using the DSL syntax http > :mydestination
or :mydestination > log
.
Second, you may need to determine the output channel of a stream based on some information that is only known at runtime. In that case, a router may be used in the sink position of a stream definition. For more information, refer to the Router Sink starter’s README.
33. Stream applications with multiple binder configurations
In some cases, a stream can have its applications bound to multiple spring cloud stream binders when they are required to connect to different messaging middleware configurations. In those cases, it is important to make sure the applications are configured appropriately with their binder configurations. For example, let’s consider the following stream:
http | transform --expression=payload.toUpperCase() | log
and in this stream, each application connects to messaging middleware in the following way:
Http source sends events to RabbitMQ (rabbit1)
Transform processor receives events from RabbitMQ (rabbit1) and sends the processed events into Kafka (kafka1)
Log sink receives events from Kafka (kafka1)
Here, rabbit1
and kafka1
are the binder names given in the spring cloud stream application properties.
Based on this setup, the applications will have the following binder(s) in their classpath with the appropriate configuration:
Http - Rabbit binder
Transform - Both Kafka and Rabbit binders
Log - Kafka binder
The spring-cloud-stream binder
configuration properties can be set within the applications themselves.
If not, they can be passed via deployment
properties when the stream is deployed.
For example,
dataflow:>stream create --definition "http | transform --expression=payload.toUpperCase() | log" --name mystream
dataflow:>stream deploy mystream --properties "app.http.spring.cloud.stream.bindings.output.binder=rabbit1,app.transform.spring.cloud.stream.bindings.input.binder=rabbit1,
app.transform.spring.cloud.stream.bindings.output.binder=kafka1,app.log.spring.cloud.stream.bindings.input.binder=kafka1"
One can override any of the binder configuration properties by specifying them via deployment properties.
Tasks
This section goes into more detail about how you can work with Spring Cloud Task. It covers topics such as creating and running task applications.
If you’re just starting out with Spring Cloud Data Flow, you should probably read the Getting Started guide before diving into this section.
34. Introducing Spring Cloud Task
A task executes a process on demand. In this case a task is a
Spring Boot application that is annotated with
@EnableTask
. Hence a user launches a task that performs a certain process, and once
complete the task ends. An example of a task would be a boot application that exports
data from a JDBC repository to an HDFS instance. Tasks record the start time and the end
time as well as the boot exit code in a relational database. The task implementation is
based on the Spring Cloud Task project.
35. The Lifecycle of a task
Before we dive deeper into the details of creating Tasks, we need to understand the typical lifecycle for tasks in the context of Spring Cloud Data Flow:
-
Register a Task App
-
Create a Task Definition
-
Launch a Task
-
Task Execution
-
Destroy a Task Definition
35.1. Creating a custom Task Application
While Spring Cloud Task does provide a number of out of the box applications (via the spring-cloud-task-app-starters), most task applications will be custom developed. In order to create a custom task application:
-
Create a new project via Spring Initializer via either the website or your IDE making sure to select the following starters:
-
Cloud Task
- This dependency is thespring-cloud-starter-task
. -
JDBC
- This is the dependency for thespring-jdbc
starter.
-
-
Within your new project, create a new class that will serve as your main class:
@EnableTask
@SpringBootApplication
public class MyTask {
public static void main(String[] args) {
SpringApplication.run(MyTask.class, args);
}
}
-
With this, you’ll need one or more
CommandLineRunner
orApplicationRunner
within your application. You can either implement your own or use the ones provided by Spring Boot (there is one for running batch jobs for example). -
Packaging your application up via Spring Boot into an über jar is done via the standard Boot conventions.
-
The packaged application can be registered and deployed as noted below.
35.2. Registering a Task Application
Register a Task App with the App Registry using the Spring Cloud Data Flow Shell
app register
command. You must provide a unique name and a URI that can be
resolved to the app artifact. For the type, specify "task". Here are a few examples:
dataflow:>app register --name task1 --type task --uri maven://com.example:mytask:1.0.2
dataflow:>app register --name task2 --type task --uri file:///Users/example/mytask-1.0.2.jar
dataflow:>app register --name task3 --type task --uri http://example.com/mytask-1.0.2.jar
When providing a URI with the maven
scheme, the format should conform to the following:
maven://<groupId>:<artifactId>[:<extension>[:<classifier>]]:<version>
If you would like to register multiple apps at one time, you can store them in a properties file
where the keys are formatted as <type>.<name>
and the values are the URIs. For example, this
would be a valid properties file:
task.foo=file:///tmp/foo.jar
task.bar=file:///tmp/bar.jar
Then use the app import
command and provide the location of the properties file via --uri
:
app import --uri file:///tmp/task-apps.properties
For convenience, we have the static files with application-URIs (for both maven and docker) available for all the out-of-the-box Task app-starters. You can point to this file and import all the application-URIs in bulk. Otherwise, as explained in previous paragraphs, you can register them individually or have your own custom property file with only the required application-URIs in it. It is recommended, however, to have a "focused" list of desired application-URIs in a custom property file.
List of available static property files:
Artifact Type | Stable Release | SNAPSHOT Release |
---|---|---|
Maven |
http://bit.ly/Belmont-BUILD-SNAPSHOT-task-applications-maven |
|
Docker |
http://bit.ly/Belmont-BUILD-SNAPSHOT-task-applications-docker |
For example, if you would like to register all out-of-the-box task applications in bulk, you can with the following command.
dataflow:>app import --uri http://bit.ly/Belmont-RC1-task-applications-maven
You can also pass the --local
option (which is TRUE by default) to indicate whether the
properties file location should be resolved within the shell process itself. If the location should
be resolved from the Data Flow Server process, specify --local false
.
When using either app register
or app import
, if a task app is already registered with
the provided name, it will not be overridden by default. If you would like to override the
pre-existing task app, then include the --force
option.
In some cases the Resource is resolved on the server side, whereas in others the URI will be passed to a runtime container instance where it is resolved. Consult the specific documentation of each Data Flow Server for more detail. |
35.3. Creating a Task
Create a Task Definition from a Task App by providing a definition name as well as
properties that apply to the task execution. Creating a task definition can be done via
the restful API or the shell. To create a task definition using the shell, use the
task create
command to create the task definition. For example:
dataflow:>task create mytask --definition "timestamp --format=\"yyyy\""
Created new task 'mytask'
A listing of the current task definitions can be obtained via the restful API or the
shell. To get the task definition list using the shell, use the task list
command.
35.4. Launching a Task
An adhoc task can be launched via the restful API or via the shell. To launch an ad-hoc
task via the shell use the task launch
command. For example:
dataflow:>task launch mytask
Launched task 'mytask'
When a task is launched, any properties that need to be passed as the command line arguments to the task application can be set when launching the task as follows:
dataflow:>task launch mytask --arguments "--server.port=8080,--foo=bar"
Additional properties meant for a TaskLauncher
itself can be passed
in using a --properties
option. Format of this option is a comma
delimited string of properties prefixed with app.<task definition
name>.<property>
. Properties are passed
to TaskLauncher
as application properties and it is up to an
implementation to choose how those are passed into an actual task
application. If the property is prefixed with deployer
instead of app
it is
passed to TaskLauncher
as a deployment property and its meaning may
be TaskLauncher
implementation specific.
dataflow:>task launch mytask --properties "deployer.timestamp.foo1=bar1,app.timestamp.foo2=bar2"
35.4.1. Common application properties
In addition to configuration via DSL, Spring Cloud Data Flow provides a mechanism for setting common properties to all
the task applications that are launched by it.
This can be done by adding properties prefixed with spring.cloud.dataflow.applicationProperties.task
when starting the server.
When doing so, the server will pass all the properties, without the prefix, to the instances it launches.
For example, all the launched applications can be configured to use the properties foo
and fizz
by launching the Data Flow server
with the following options:
--spring.cloud.dataflow.applicationProperties.task.foo=bar
--spring.cloud.dataflow.applicationProperties.task.fizz=bar2
This will cause the properties foo=bar
and fizz=bar2
to be passed to all the launched applications.
Properties configured using this mechanism have lower precedence than task deployment properties.
They will be overridden if a property with the same key is specified at task launch time (e.g. app.trigger.fizz
will override the common property).
|
35.5. Reviewing Task Executions
Once the task is launched the state of the task is stored in a relational DB. The state includes:
-
Task Name
-
Start Time
-
End Time
-
Exit Code
-
Exit Message
-
Last Updated Time
-
Parameters
A user can check the status of their task executions via the restful API or by the shell.
To display the latest task executions via the shell use the task execution list
command.
To get a list of task executions for just one task definition, add --name
and
the task definition name, for example task execution list --name foo
. To retrieve full
details for a task execution use the task display
command with the id of the task execution,
for example task display --id 549
.
35.6. Destroying a Task
Destroying a Task Definition will remove the definition from the definition repository.
This can be done via the restful API or via the shell. To destroy a task via the shell
use the task destroy
command. For example:
dataflow:>task destroy mytask
Destroyed task 'mytask'
The task execution information for previously launched tasks for the definition will remain in the task repository.
This will not stop any currently executing tasks for this definition, instead it just removes the task definition from the database. |
36. Task Repository
Out of the box Spring Cloud Data Flow offers an embedded instance of the H2 database. The H2 is good for development purposes but is not recommended for production use.
36.1. Configuring the Task Execution Repository
To add a driver for the database that will store the Task Execution information, a dependency for the driver will need to be added to a maven pom file and the Spring Cloud Data Flow will need to be rebuilt. Since Spring Cloud Data Flow is comprised of an SPI for each environment it supports, please review the SPI’s documentation on which POM should be updated to add the dependency and how to build. This document will cover how to setup the dependency for local SPI.
36.1.1. Local
-
Open the spring-cloud-dataflow-server-local/pom.xml in your IDE.
-
In the
dependencies
section add the dependency for the database driver required. In the sample below postgresql has been chosen.
<dependencies>
...
<dependency>
<groupId>org.postgresql</groupId>
<artifactId>postgresql</artifactId>
</dependency>
...
</dependencies>
-
Save the changed pom.xml
-
Build the application as described here: Building Spring Cloud Data Flow
36.1.2. Task Application Repository
When launching a task application be sure that the database driver that is being used by Spring Cloud Data Flow is also a dependency on the task application. For example if your Spring Cloud Data Flow is set to use Postgresql, be sure that the task application also has Postgresql as a dependency.
When executing tasks externally (i.e. command line) and you wish for Spring Cloud Data Flow to show the TaskExecutions in its UI, be sure that common datasource settings are shared among the both. By default Spring Cloud Task will use a local H2 instance and the execution will not be recorded to the database used by Spring Cloud Data Flow. |
36.2. Datasource
To configure the datasource Add the following properties to the dataflow-server.yml or via environment variables:
-
spring.datasource.url
-
spring.datasource.username
-
spring.datasource.password
-
spring.datasource.driver-class-name
For example adding postgres would look something like this:
-
Environment variables:
export spring_datasource_url=jdbc:postgresql://localhost:5432/mydb
export spring_datasource_username=myuser
export spring_datasource_password=mypass
export spring_datasource_driver-class-name="org.postgresql.Driver"
-
dataflow-server.yml
spring:
datasource:
url: jdbc:postgresql://localhost:5432/mydb
username: myuser
password: mypass
driver-class-name:org.postgresql.Driver
37. Subscribing to Task/Batch Events
You can also tap into various task/batch events when the task is launched.
If the task is enabled to generate task and/or batch events (with the additional dependencies spring-cloud-task-stream
and spring-cloud-stream-binder-kafka
, in the case of Kafka as the binder), those events are published during the task lifecycle.
By default, the destination names for those published events on the broker (rabbit, kafka etc.,) are the event names themselves (for instance: task-events
, job-execution-events
etc.,).
dataflow:>task create myTask --definition “myBatchJob"
dataflow:>task launch myTask
dataflow:>stream create task-event-subscriber1 --definition ":task-events > log" --deploy
You can control the destination name for those events by specifying explicit names when launching the task such as:
dataflow:>task launch myTask --properties "spring.cloud.stream.bindings.task-events.destination=myTaskEvents"
dataflow:>stream create task-event-subscriber2 --definition ":myTaskEvents > log" --deploy
The default Task/Batch event and destination names on the broker are enumerated below:
Event |
Destination |
Task events |
|
Job Execution events |
|
Step Execution events |
|
Item Read events |
|
Item Process events |
|
Item Write events |
|
Skip events |
|
38. Launching Tasks from a Stream
You can launch a task from a stream by using one of the available task-launcher
sinks. Currently the platforms supported
via the task-launcher
sinks are
local,
Cloud Foundry, and
Yarn.
task-launcher-local is meant for development purposes only.
|
A task-launcher
sink expects a message containing a TaskLaunchRequest object in its payload. From the TaskLaunchRequest
object the task-launcher
will obtain the URI of the artifact to be launched as well as the environment properties, command line arguments, deployment properties and application name to be used by the task.
The task-launcher-local can be added to the available sinks by executing the app register command as follows (for the Rabbit Binder):
app register --name task-launcher-local --type sink --uri maven://org.springframework.cloud.stream.app:task-launcher-local-sink-rabbit:jar:1.2.0.RELEASE
In the case of a maven based task that is to be launched, the task-launcher
application is responsible for downloading the artifact. You must configure the task-launcher
with the appropriate configuration of Maven Properties such as --maven.remote-repositories.repo1.url=http://repo.spring.io/libs-milestone"
to resolve artifacts, in this case against a milestone repo. Note that this repo can be different than the one used to register the task-launcher
application itself.
38.1. TriggerTask
One way to launch a task using the task-launcher
is to use the triggertask source. The triggertask
source
will emit a message with a TaskLaunchRequest
object containing the required launch information.
The triggertask
can be added to the available sources by executing the app register command as follows (for the Rabbit Binder):
app register --type source --name triggertask --uri maven://org.springframework.cloud.stream.app:triggertask-source-rabbit:1.2.0.RELEASE
An example of this would be to launch the timestamp task once every 60 seconds, the stream to implement this would look like:
stream create foo --definition "triggertask --triggertask.uri=maven://org.springframework.cloud.task.app:timestamp-task:jar:1.2.0.RELEASE --trigger.fixed-delay=60 --triggertask.environment-properties=spring.datasource.url=jdbc:h2:tcp://localhost:19092/mem:dataflow,spring.datasource.username=sa | task-launcher-local --maven.remote-repositories.repo1.url=http://repo.spring.io/libs-release" --deploy
If you execute runtime apps
you can find the log file for the task launcher sink. Tailing that file you can find the log file for the launched tasks. The setting of triggertask.environment-properties
is so that all the task executions can be collected in the same H2 database used in the local version of the Data Flow Server. You can then see the list of task executions using the shell command task execution list
dataflow:>task execution list
╔════════════════════╤══╤════════════════════════════╤════════════════════════════╤═════════╗
║ Task Name │ID│ Start Time │ End Time │Exit Code║
╠════════════════════╪══╪════════════════════════════╪════════════════════════════╪═════════╣
║timestamp-task_26176│4 │Tue May 02 12:13:49 EDT 2017│Tue May 02 12:13:49 EDT 2017│0 ║
║timestamp-task_32996│3 │Tue May 02 12:12:49 EDT 2017│Tue May 02 12:12:49 EDT 2017│0 ║
║timestamp-task_58971│2 │Tue May 02 12:11:50 EDT 2017│Tue May 02 12:11:50 EDT 2017│0 ║
║timestamp-task_13467│1 │Tue May 02 12:10:50 EDT 2017│Tue May 02 12:10:50 EDT 2017│0 ║
╚════════════════════╧══╧════════════════════════════╧════════════════════════════╧═════════╝
38.2. TaskLaunchRequest-transform
Another option to start a task using the task-launcher
would be to create a stream using the
Tasklaunchrequest-transform processor to translate a message payload to a TaskLaunchRequest
.
The tasklaunchrequest-transform
can be added to the available processors by executing the app register command as follows (for the Rabbit Binder):
app register --type processor --name tasklaunchrequest-transform --uri maven://org.springframework.cloud.stream.app:tasklaunchrequest-transform-processor-rabbit:1.2.0.RELEASE
For example:
stream create task-stream --definition "http --port=9000 | tasklaunchrequest-transform --uri=maven://org.springframework.cloud.task.app:timestamp-task:jar:1.2.0.RELEASE | task-launcher-local --maven.remote-repositories.repo1.url=http://repo.spring.io/libs-release"
39. Composed Tasks
Spring Cloud Data Flow allows a user to create a directed graph where each node of the graph is a task application. This is done by using the DSL for composed tasks. A composed task can be created via the RESTful API, the Spring Cloud Data Flow Shell, or the Spring Cloud Data Flow UI.
39.1. Configuring the Composed Task Runner in Spring Cloud Data Flow
Composed tasks are executed via a task application called the Composed Task Runner.
39.1.1. Registering the Composed Task Runner application
Out of the box the Composed Task Runner application is not registered with Spring Cloud Data Flow. So, to launch composed tasks we must first register the Composed Task Runner as an application with Spring Cloud Data Flow as follows:
app register --name composed-task-runner --type task --uri maven://org.springframework.cloud.task.app:composedtaskrunner-task:<DESIRED_VERSION>
You can also configure Spring Cloud Data Flow to use a different task definition
name for the composed task runner. This can be done by setting the
spring.cloud.dataflow.task.composedTaskRunnerName
property to the name
of your choice. You can then register the composed task runner application with
the name you set using that property.
39.1.2. Configuring the Composed Task Runner application
The Composed Task Runner application has a dataflow.server.uri
property that is used for validation and for launching child tasks. This defaults
to localhost:9393
. If you run a distributed Spring Cloud Data Flow server, like you would do if you deploy the server on Cloud Foundry,
YARN or Kubernetes, then you need to provide the URI that can be used to access the server. You can either provide this dataflow.server.uri
property for the Composed Task Runner application when launching a composed task, or you can provide a spring.cloud.dataflow.server.uri
property
for the Spring Cloud Data Flow server when it is started. For the latter case the dataflow.server.uri
Composed Task Runner application property
will be automatically set when a composed task is launched.
39.2. Creating, Launching, and Destroying a Composed Task
39.2.1. Creating a Composed Task
The DSL for the composed tasks is used when creating a task definition via the task create command. For example:
dataflow:> app register --name timestamp --type task --uri maven://org.springframework.cloud.task.app:timestamp-task:<DESIRED_VERSION>
dataflow:> app register --name mytaskapp --type task --uri file:///home/tasks/mytask.jar
dataflow:> task create my-composed-task --definition "mytaskapp && timestamp"
dataflow:> task launch my-composed-task
In the example above we assume that the applications to be used by our composed task have not been registered yet. So the first two steps we register two task applications. We then create our composed task definition by using the task create command. The composed task DSL in the example above will, when launched, execute mytaskapp and then execute the timestamp application.
But before we launch the my-composed-task definition, we can view what Spring Cloud Data Flow generated for us. This can be done by executing the task list command.
dataflow:>task list
╔══════════════════════════╤═══════════════════════════════════════════════════════════════
║ Task Name │ Task Definition
╠══════════════════════════╪═══════════════════════════════════════════════════════════════
║my-composed-task │mytaskapp && timestamp
║my-composed-task-mytaskapp│mytaskapp
║my-composed-task-timestamp│timestamp
Spring Cloud Data Flow created three task definitions, one for each of the
applications that comprises our composed task (my-composed-task-mytaskapp
and
my-composed-task-timestamp
) as well as the composed task (my-composed-task
)
definition. We also see that each of the generated
names for the child tasks is comprised of the name of the composed task and
the name of the application separated by a dash -
. i.e. my-composed-task -
mytaskapp.
Task Application Parameters
The task applications that comprise the composed task definition can also contain parameters. For example:
dataflow:> task create my-composed-task --definition "mytaskapp --displayMessage=hello && timestamp --format=YYYY"
39.2.2. Launching a Composed Task
Launching a composed task is done the same way as launching a stand-alone task. i.e.
task launch my-composed-task
Once the task is launched and assuming all the tasks complete successfully you will
see three task executions when executing a task execution list
. For example:
dataflow:>task execution list
╔══════════════════════════╤═══╤════════════════════════════╤════════════════════════════╤═════════╗
║ Task Name │ID │ Start Time │ End Time │Exit Code║
╠══════════════════════════╪═══╪════════════════════════════╪════════════════════════════╪═════════╣
║my-composed-task-timestamp│713│Wed Apr 12 16:43:07 EDT 2017│Wed Apr 12 16:43:07 EDT 2017│0 ║
║my-composed-task-mytaskapp│712│Wed Apr 12 16:42:57 EDT 2017│Wed Apr 12 16:42:57 EDT 2017│0 ║
║my-composed-task │711│Wed Apr 12 16:42:55 EDT 2017│Wed Apr 12 16:43:15 EDT 2017│0 ║
╚══════════════════════════╧═══╧════════════════════════════╧════════════════════════════╧═════════╝
In the example above we see that my-compose-task launched and it also launched
the other tasks in sequential order and all of them executed successfully with
"Exit Code" as 0
.
Exit Statuses
The following list shows how the Exit Status will be set for each step (task) contained in the composed task following each step execution.
-
If the
TaskExecution
has anExitMessage
that will be used as theExitStatus
-
If no
ExitMessage
is present and theExitCode
is set to zero then theExitStatus
for the step will beCOMPLETED
. -
If no
ExitMessage
is present and theExitCode
is set to any non zero number then theExitStatus
for the step will beFAILED
.
39.2.3. Destroying a Composed Task
The same command used to destroy a stand-alone task is the same as destroying a composed task. The only difference is that destroying a composed task will also destroy the child tasks associated with it. For example
dataflow:>task list
╔══════════════════════════╤═══════════════════════════════════════════════════════════════
║ Task Name │ Task Definition
╠══════════════════════════╪═══════════════════════════════════════════════════════════════
║my-composed-task │mytaskapp && timestamp
║my-composed-task-mytaskapp│mytaskapp
║my-composed-task-timestamp│timestamp
...
dataflow:>task destroy my-composed-task
dataflow:>task list
╔══════════════════════════╤═══════════════════════════════════════════════════════════════
║ Task Name │ Task Definition
╠══════════════════════════╪═══════════════════════════════════════════════════════════════
╚══════════════════════════╧═══════════════════════════════════════════════════════════════
39.2.4. Stopping a Composed Task
In cases where a composed task execution needs to be stopped. This can be done via the:
-
RESTful API
-
Spring Cloud Data Flow Dashboard by selecting the Job’s tab and then clicking the stop button by the job execution that needs to be stopped.
The composed task run will be stopped
when the currently running child task completes. The step associated with the
child task that was running at the time that the composed task was stopped will
be marked as STOPPED
as well as the composed task job execution.
39.2.5. Restarting a Composed Task
In cases where a composed task fails during execution and the status of the
composed task is FAILED
then the task can be restarted. This can be done
via the:
-
RESTful API
-
Shell by launching the task using the same parameters
-
Spring Cloud Data Flow Dashboard by selecting the Job’s tab and then clicking the restart button by the job execution that needs to be restarted.
Restarting a Composed Task job that has been stopped (via the
Spring Cloud Data Flow Dashboard or RESTful API), will relaunch the
STOPPED child task, and then launch the remaining (unlaunched) child tasks
in the specified order.
|
39.3. Composed Task DSL
39.3.1. Conditional Execution
Conditional execution is expressed using a double ampersand symbol &&
.
This allows each task in the sequence to be launched only if the previous task
successfully completed. For example:
task create my-composed-task --definition "foo && bar"
When the composed task my-composed-task is launched, it will launch the
task foo
and if it completes successfully, then the task bar
will be
launched. If the foo
task fails, then the task bar
will not launch.
You can also use the Spring Cloud Data Flow Dashboard to create your conditional execution. By using the designer to drag and drop applications that are required, and connecting them together to create your directed graph. For example:
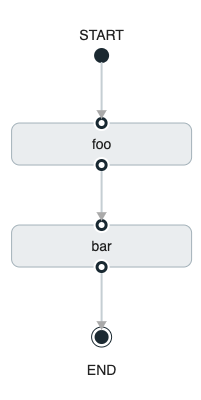
The diagram above is a screen capture of the directed graph as it being created using the Spring Cloud Data Flow Dashboard. We see that are 4 components in the diagram that comprise a conditional execution:
-
Start icon - All directed graphs start from this symbol. There will only be one.
-
Task icon - Represents each task in the directed graph.
-
End icon - Represents the termination of a directed graph.
-
Solid line arrow - Represents the flow conditional execution flow between:
-
Two applications
-
The start control node and an application
-
An application and the end control node
-
You can view a diagram of your directed graph by clicking the detail button next to the composed task definition on the definitions tab. |
39.3.2. Transitional Execution
The DSL supports fine grained control over the transitions taken during the
execution of the directed graph. Transitions are specified by providing a
condition for equality based on the exit status of the previous task.
A task transition is represented by the following symbol ->
.
Basic Transition
A basic transition would look like the following:
task create my-transition-composed-task --definition "foo 'FAILED' -> bar 'COMPLETED' -> baz"
In the example above foo
would launch and if it had an exit status of FAILED
,
then the bar
task would launch. If the exit status of foo
was COMPLETED
then baz
would launch. All other statuses returned by foo
will have no effect
and task would terminate normally.
Using the Spring Cloud Data Flow Dashboard to create the same "basic transition" would look like:
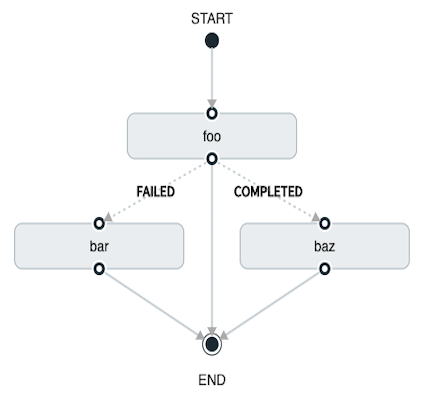
The diagram above is a screen capture of the directed graph as it being created using the Spring Cloud Data Flow Dashboard. Notice that there are 2 different types of connectors:
-
Dashed line - Is the line used to represent transitions from the application to one of the possible destination applications.
-
Solid line - Used to connect applications in a conditional execution or a connection between the application and a control node (end, start).
When creating a transition, link the application to each of possible destination using the connector. Once complete go to each connection and select it by clicking it. A bolt icon should appear, click that icon and enter the exit status required for that connector. The solid line for that connector will turn to a dashed line.
Transition With a Wildcard
Wildcards are supported for transitions by the DSL for example:
task create my-transition-composed-task --definition "foo 'FAILED' -> bar '*' -> baz"
In the example above foo
would launch and if it had an exit status of FAILED
,
then the bar
task would launch. Any exit status of foo
other than FAILED
then baz
would launch.
Using the Spring Cloud Data Flow Dashboard to create the same "transition with wildcard" would look like:
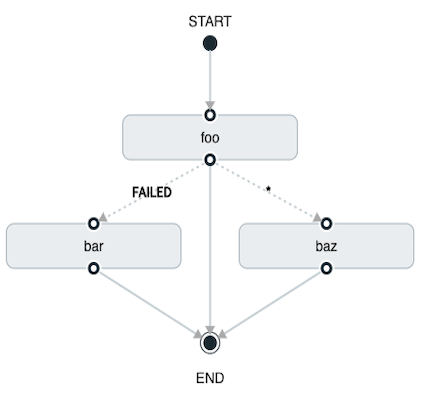
Transition With a Following Conditional Execution
A transition can be followed by a conditional execution so long as the wildcard is not used. For example:
task create my-transition-conditional-execution-task --definition "foo 'FAILED' -> bar 'UNKNOWN' -> baz && qux && quux"
In the example above foo
would launch and if it had an exit status of FAILED
,
then the bar
task would launch. If foo
had an exit status of UNKNOWN
then
baz
would launch. Any exit status of foo
other than FAILED
or UNKNOWN
then qux
would launch and upon successful completion quux
would launch.
Using the Spring Cloud Data Flow Dashboard to create the same "transition with conditional execution" would look like:
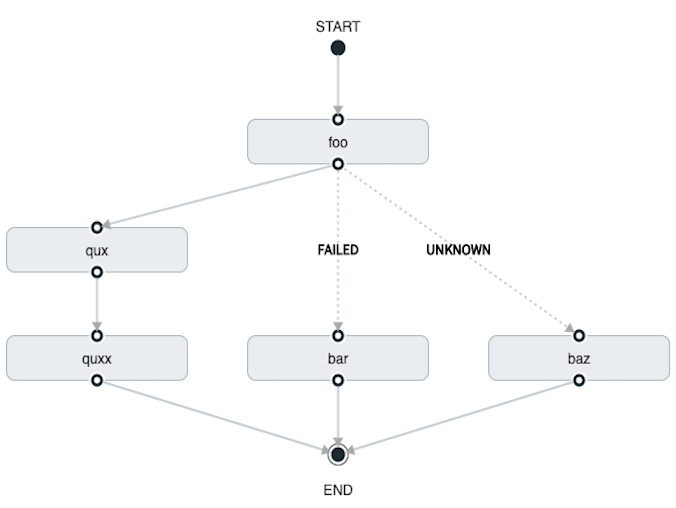
In this diagram we see the dashed line (transition) connecting the foo application
to the target applications, but a solid line connecting the conditional executions
between foo , qux , and quux .
|
39.3.3. Split Execution
Splits allow for multiple tasks within a composed task to be run in parallel.
It is denoted by using angle brackets <> to group tasks and flows that are to
be run in parallel. These tasks and flows are separated by the double pipe ||
. For example:
task create my-split-task --definition "<foo || bar || baz>"
The example above will launch tasks foo
, bar
and baz
in parallel.
Using the Spring Cloud Data Flow Dashboard to create the same "split execution" would look like:
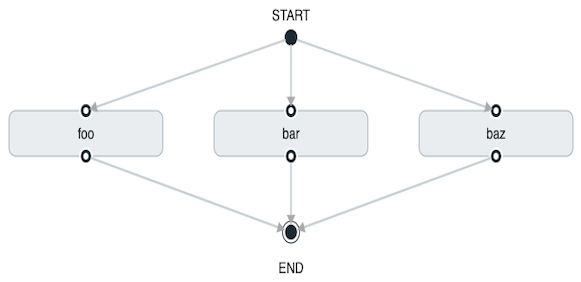
With the task DSL a user may also execute multiple split groups in succession. For example:
task create my-split-task --definition "<foo || bar || baz> && <qux || quux>"
In the example above tasks foo
, bar
and baz
will be launched in parallel,
once they all complete then tasks qux
, quux
will be launched in parallel.
Once they complete the composed task will end. However if foo
, bar
, or
baz
fails then, the split containing qux
and quux
will not launch.
Using the Spring Cloud Data Flow Dashboard to create the same "split with multiple groups" would look like:
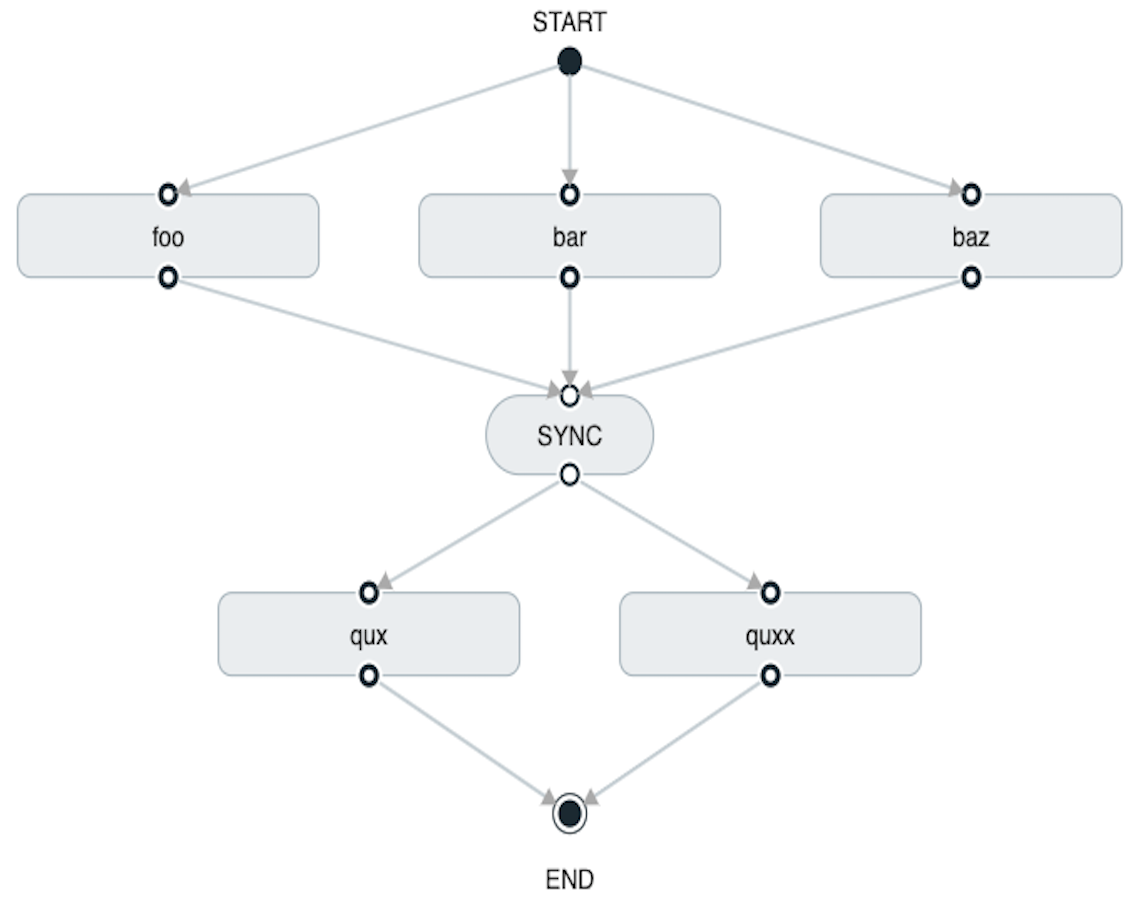
Notice that there is a SYNC
control node that is by the designer when
connecting two consecutive splits.
Split Containing Conditional Execution
A split can also have a conditional execution within the angle brackets. For example:
task create my-split-task --definition "<foo && bar || baz>"
In the example above we see that foo
and baz
will be launched in parallel,
however bar
will not launch until foo
completes successfully.
Using the Spring Cloud Data Flow Dashboard to create the same "split containing conditional execution" would look like:
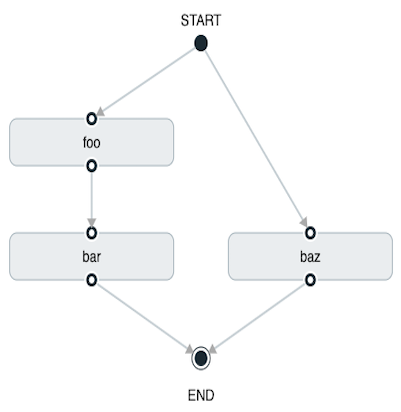
Dashboard
This section describe how to use the Dashboard of Spring Cloud Data Flow.
40. Introduction
Spring Cloud Data Flow provides a browser-based GUI and it currently includes 6 tabs:
-
Apps Lists all available applications and provides the control to register/unregister them
-
Runtime Provides the Data Flow cluster view with the list of all running applications
-
Streams List, create, deploy, and destroy Stream Definitions
-
Tasks List, create, launch and destroy Task Definitions
-
Jobs Perform Batch Job related functions
-
Analytics Create data visualizations for the various analytics applications
Upon starting Spring Cloud Data Flow, the Dashboard is available at:
http://<host>:<port>/dashboard
For example: http://localhost:9393/dashboard
If you have enabled https, then it will be located at https://localhost:9393/dashboard
.
If you have enabled security, a login form is available at http://localhost:9393/dashboard/#/login
.
The default Dashboard server port is 9393
|
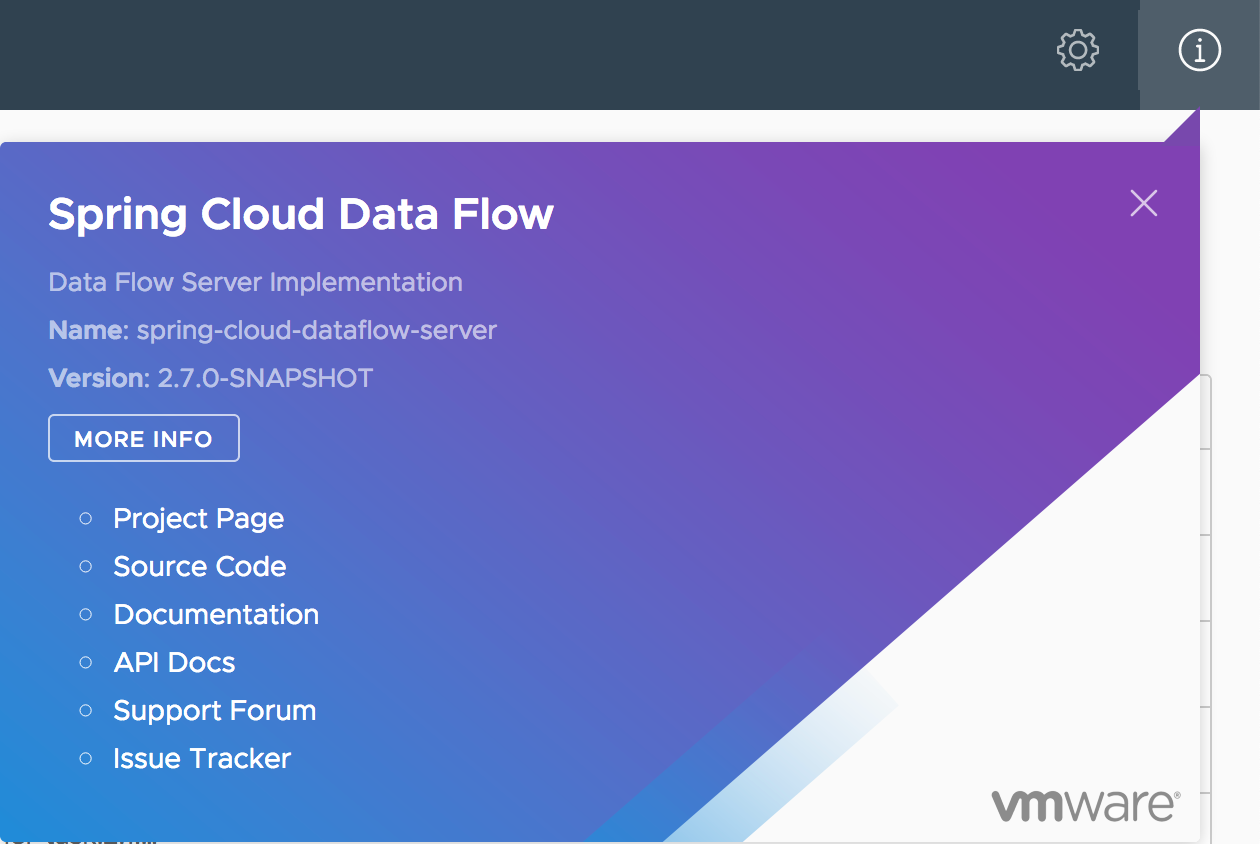
41. Apps
The Apps section of the Dashboard lists all the available applications and provides the control to register/unregister them (if applicable). It is possible to import a number of applications at once using the Bulk Import Applications action.
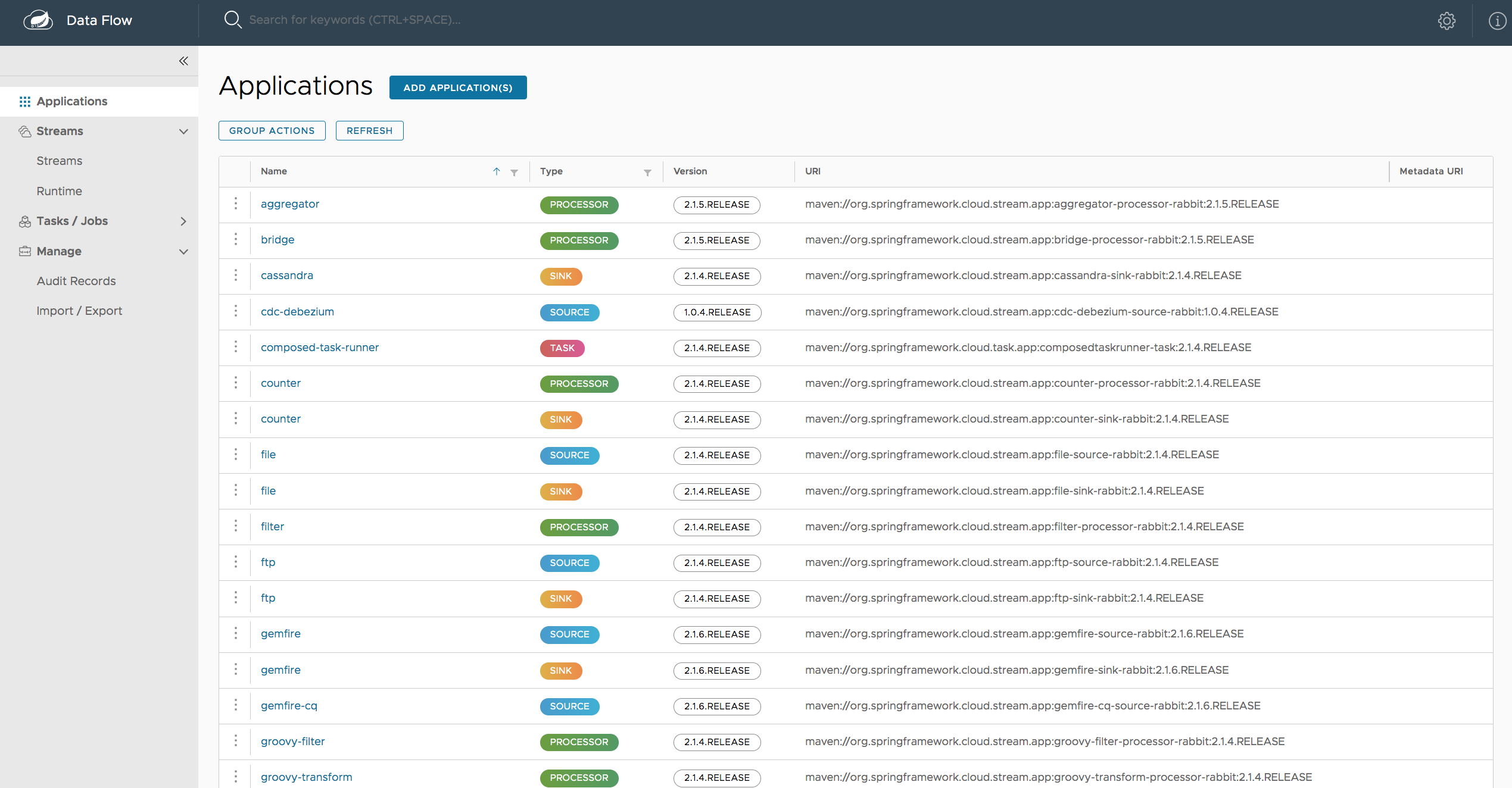
41.1. Bulk Import of Applications
The bulk import applications page provides numerous options for defining and importing a set of applications in one go. For bulk import the application definitions are expected to be expressed in a properties style:
<type>.<name> = <coordinates>
For example:
task.timestamp=maven://org.springframework.cloud.task.app:timestamp-task:1.2.0.RELEASE
processor.transform=maven://org.springframework.cloud.stream.app:transform-processor-rabbit:1.2.0.RELEASE
At the top of the bulk import page an Uri can be specified that points to a properties file stored elsewhere, it should contain properties formatted as above. Alternatively, using the textbox labeled Apps as Properties it is possible to directly list each property string. Finally, if the properties are stored in a local file the Select Properties File option will open a local file browser to select the file. After setting your definitions via one of these routes, click Import.
At the bottom of the page there are quick links to the property files for common groups of stream apps and task apps. If those meet your needs, simply select your appropriate variant (rabbit, kafka, docker, etc) and click the Import action on those lines to immediately import all those applications.
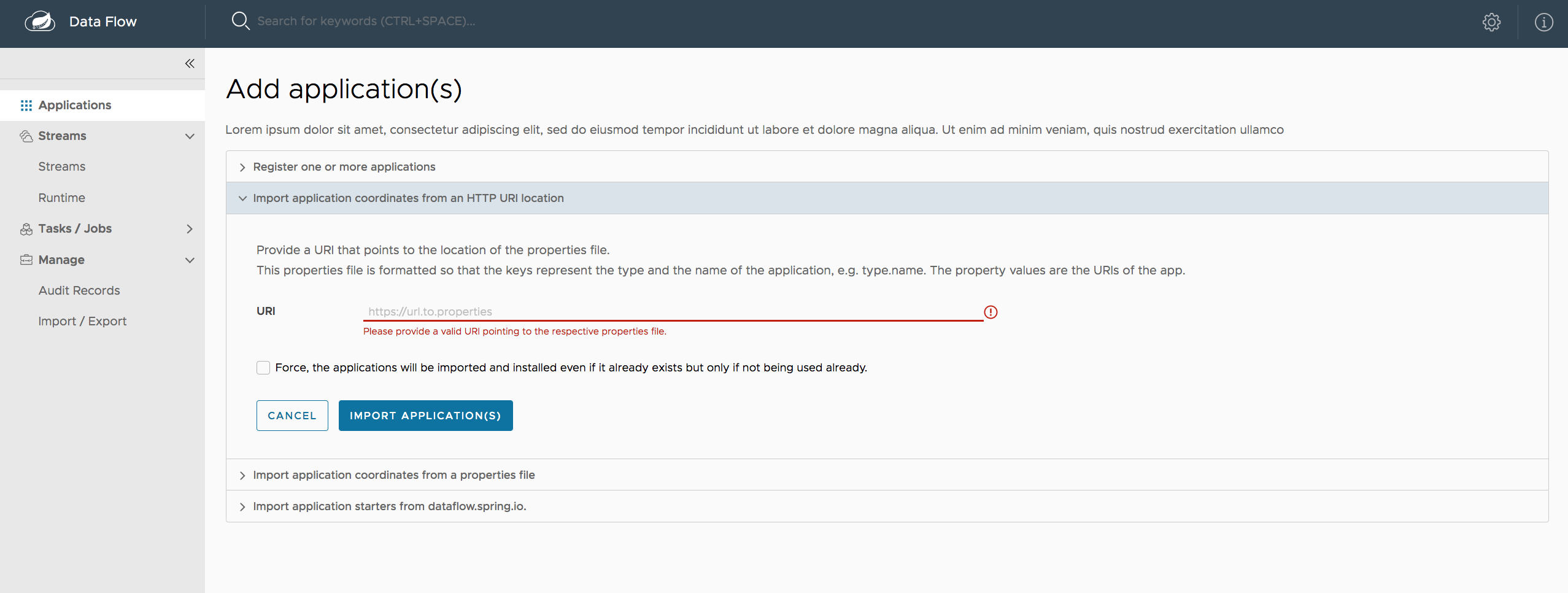
42. Runtime
The Runtime section of the Dashboard application shows the Spring Cloud Data Flow cluster view with the list of all running applications. For each runtime app the state of the deployment and the number of deployed instances is shown. A list of the used deployment properties is available by clicking on the app id.
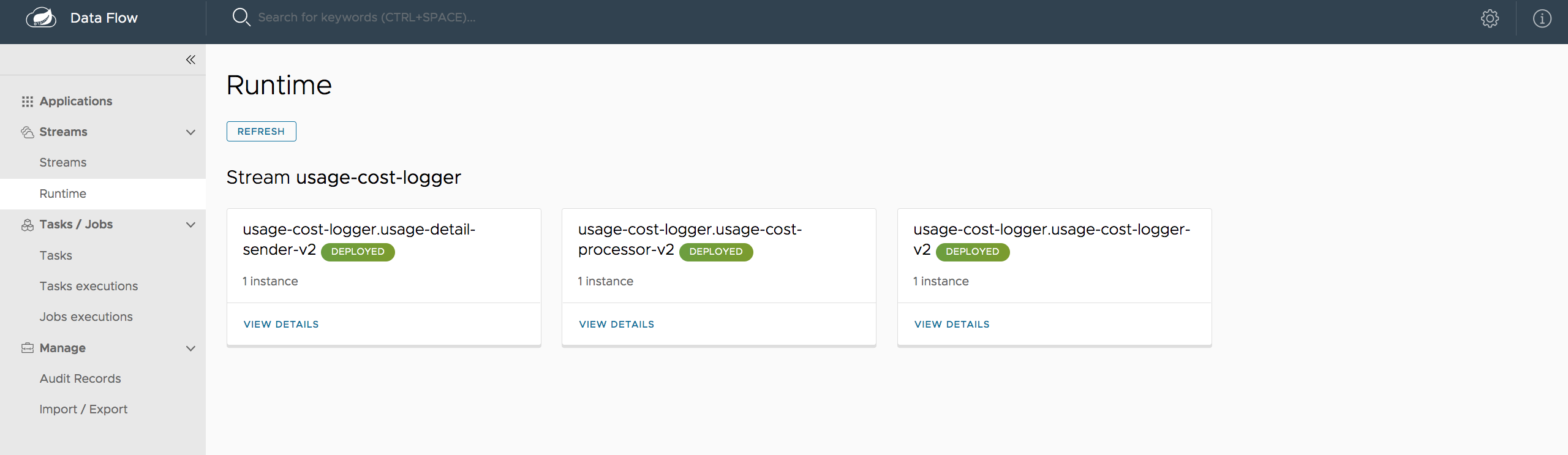
43. Streams
The Streams section of the Dashboard provides the Definitions tab that provides a listing of Stream definitions. There you have the option to deploy or undeploy those stream definitions. Additionally you can remove the definition by clicking on destroy. Each row includes an arrow on the left, which can be clicked to see a visual representation of the definition. Hovering over the boxes in the visual representation will show more details about the apps including any options passed to them. In this screenshot the timer stream has been expanded to show the visual representation:
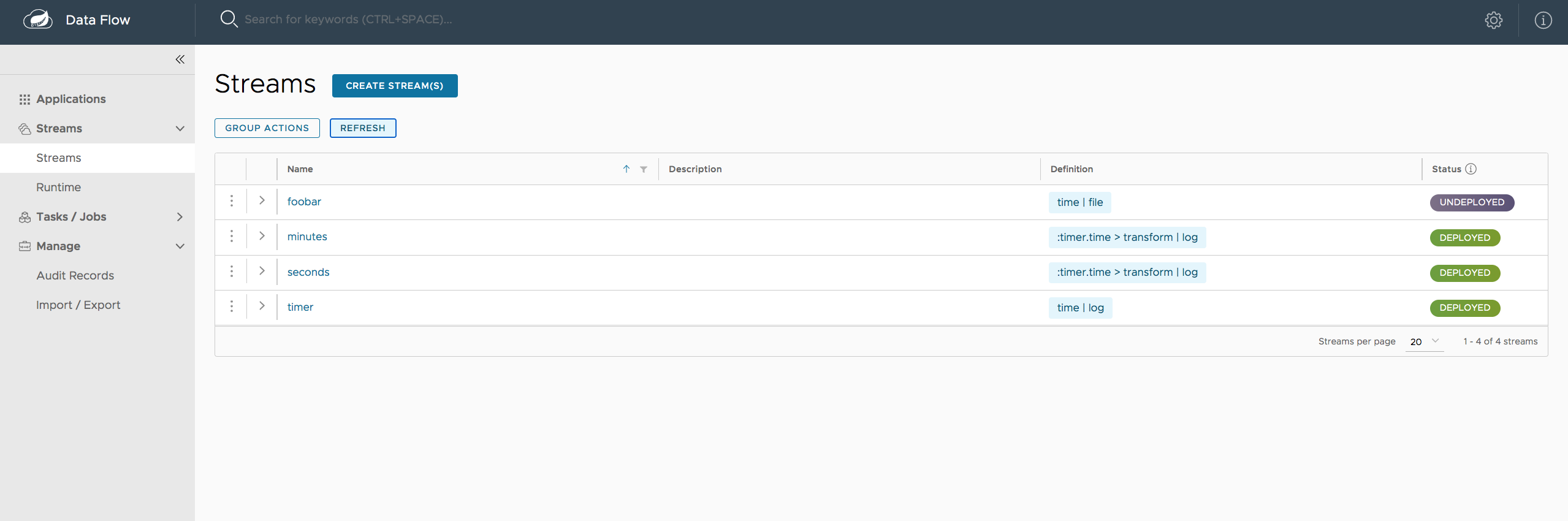
If the details button is clicked the view will change to show a visual representation of that stream and also any related streams. In the above example, if clicking details for the timer stream, the view will change to the one shown below which clearly shows the relationship between the three streams (two of them are tapping into the timer stream).
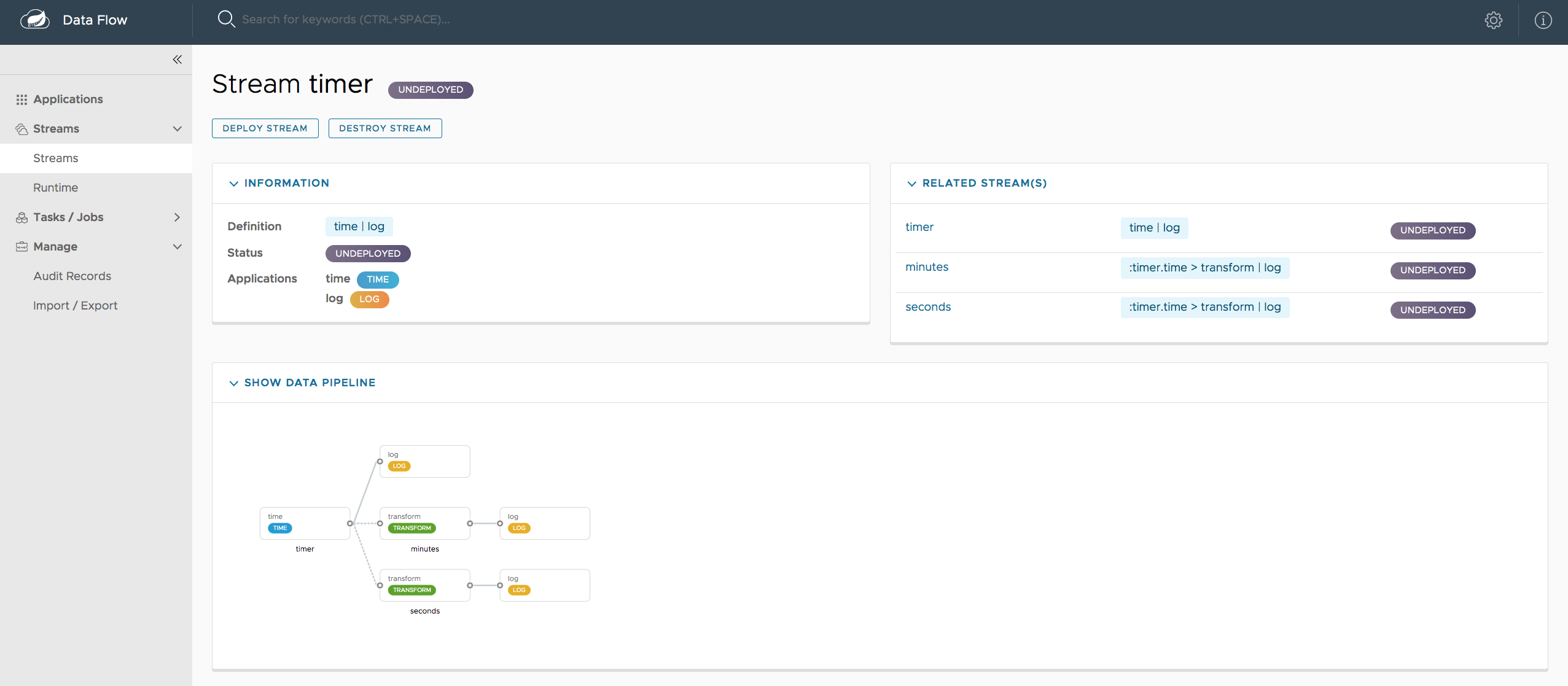
44. Create Stream
The Create Stream section of the Dashboard includes the Spring Flo designer tab that provides the canvas application, offering a interactive graphical interface for creating data pipelines.
In this tab, you can:
-
Create, manage, and visualize stream pipelines using DSL, a graphical canvas, or both
-
Write pipelines via DSL with content-assist and auto-complete
-
Use auto-adjustment and grid-layout capabilities in the GUI for simpler and interactive organization of pipelines
Watch this screencast that highlights some of the "Flo for Spring Cloud Data Flow" capabilities. Spring Flo wiki includes more detailed content on core Flo capabilities.
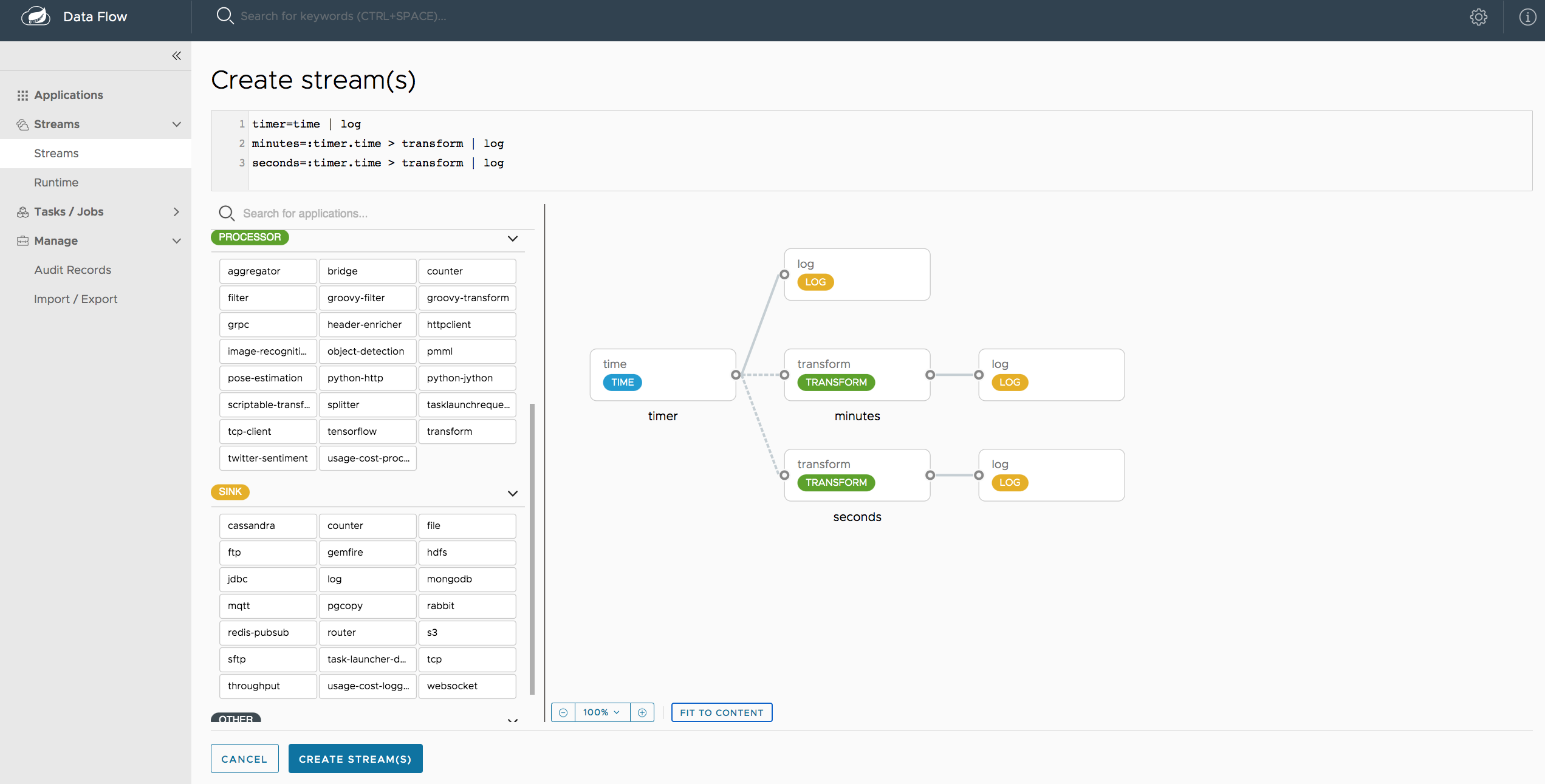
45. Tasks
The Tasks section of the Dashboard currently has three tabs:
-
Apps
-
Definitions
-
Executions
45.1. Apps
Apps encapsulate a unit of work into a reusable component. Within the Data Flow runtime environment Apps allow users to create definitions for Streams as well as Tasks. Consequently, the Apps tab within the Tasks section allows users to create Task definitions.
You will also use this tab to create Batch Jobs. |
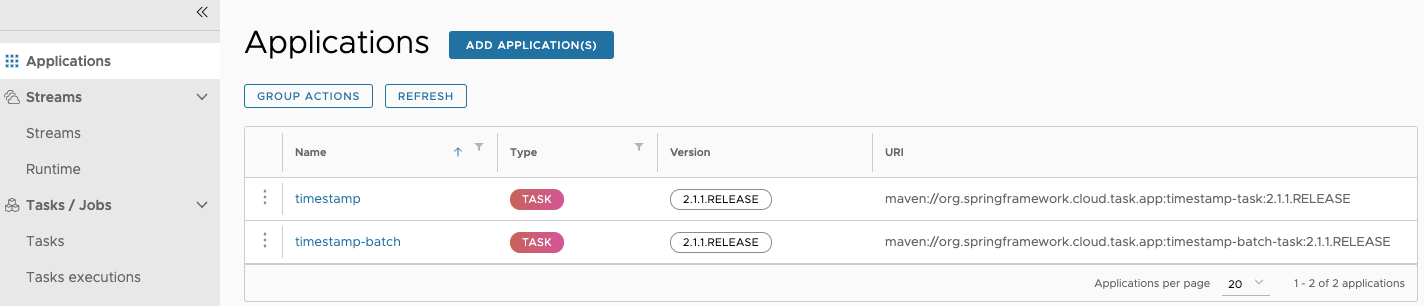
On this screen you can perform the following actions:
-
View details such as the task app options.
-
Create a Task Definition from the respective App.
45.1.1. Create a Task Definition from a selected Task App
On this screen you can create a new Task Definition. As a minimum you must provide a name for the new definition. You will also have the option to specify various properties that are used during the deployment of the app.
Each parameter is only included if the Include checkbox is selected. |
45.1.2. View Task App Details
On this page you can view the details of a selected task app, including the list of available options (properties) for that app.
45.2. Definitions
This page lists the Data Flow Task definitions and provides actions to launch or destroy those tasks. It also provides a shortcut operation to define one or more tasks using simple textual input, indicated by the bulk define tasks button.
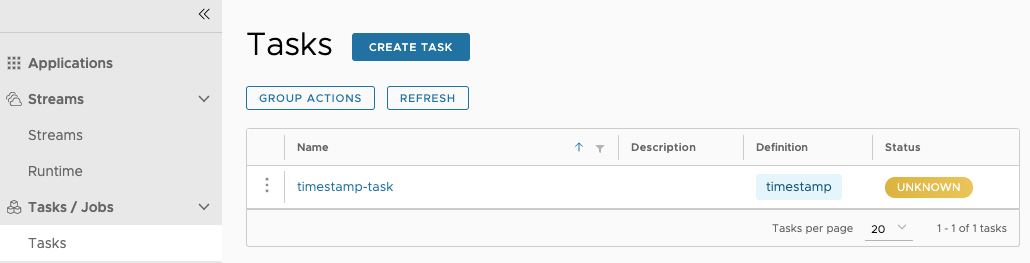
45.2.1. Creating Task Definitions using the bulk define interface
After pressing bulk define tasks, the following screen will be shown.
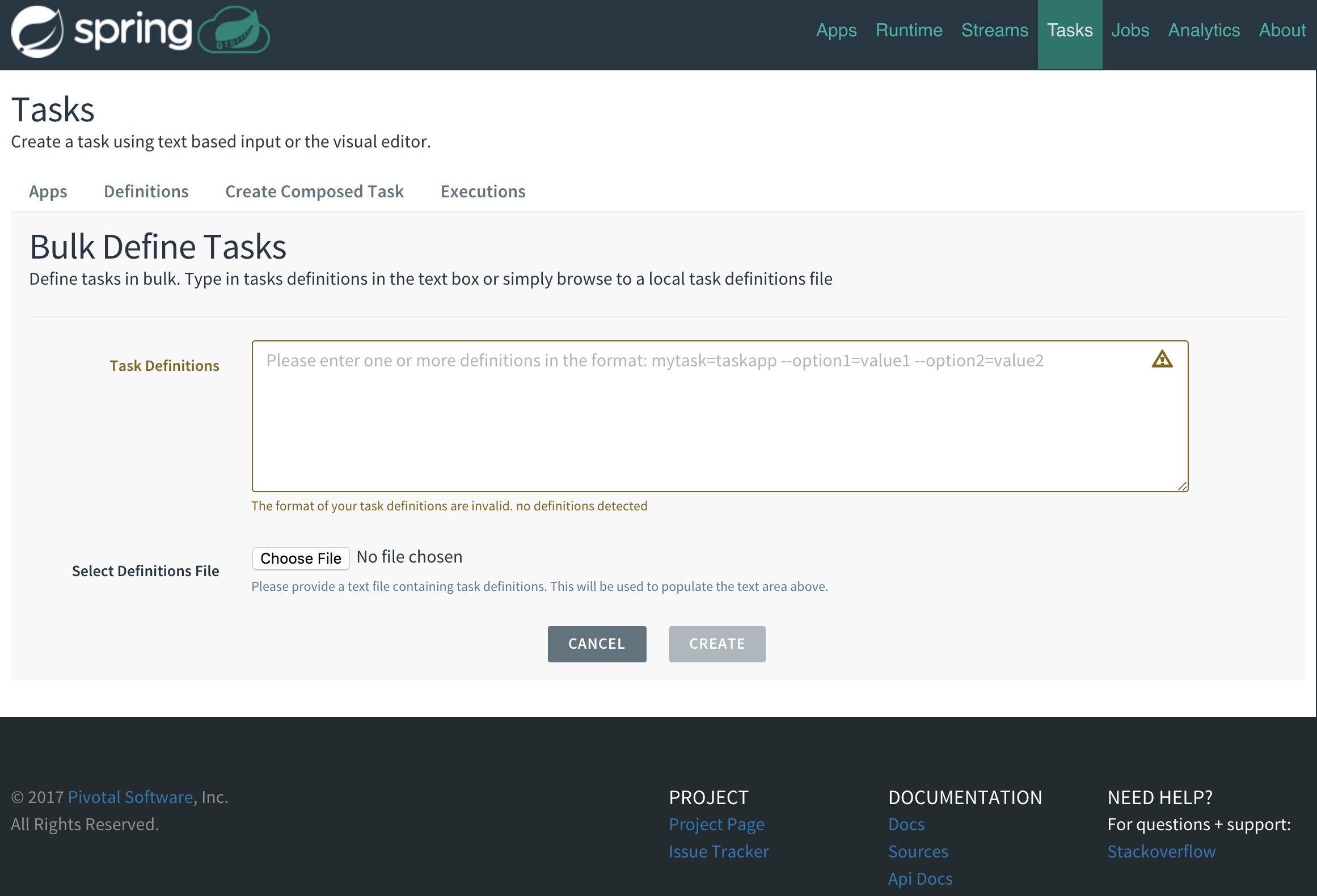
It includes a textbox where one or more definitions can be entered and then various actions performed on those definitions. The required input text format for task definitions is very basic, each line should be of the form:
<task-definition-name> = <task-application> <options>
For example:
demo-timestamp = timestamp --format=hhmmss
After entering any data a validator will run asynchronously to verify both the syntax and that the application name entered is a valid application and it supports the options specified. If validation fails the editor will show the errors with more information via tooltips.
To make it easier to enter definitions into the text area, content assist is supported. Pressing Ctrl+Space will invoke content assist to suggest simple task names (based on the line on which it is invoked), task applications and task application options. Press ESCape to close the content assist window without taking a selection.
If the validator should not verify the applications or the options (for example if specifying non-whitelisted options to the applications) then turn off that part of validation by toggling the checkbox off on the Verify Apps button - the validator will then only perform syntax checking. When correctly validated, the create button will be clickable and on pressing it the UI will proceed to create each task definition. If there are any errors during creation then after creation finishes the editor will show any lines of input, as it cannot be used in task definitions. These can then be fixed up and creation repeated. There is an import file button to open a file browser on the local file system if the definitions are in a file and it is easier to import than copy/paste.
Bulk loading of composed task definitions is not currently supported. |
45.2.2. Creating Composed Task Definitions
The dashboard includes the Create Composed Task tab that provides the canvas application, offering a interactive graphical interface for creating composed tasks.
In this tab, you can:
-
Create and visualize composed tasks using DSL, a graphical canvas, or both
-
Use auto-adjustment and grid-layout capabilities in the GUI for simpler and interactive organization of the composed task
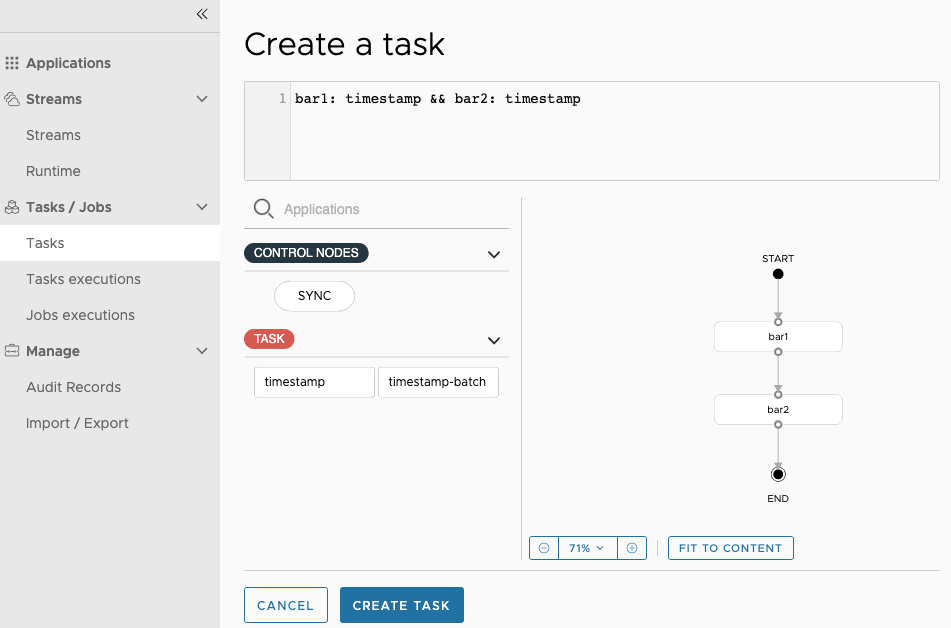
45.2.3. Launching Tasks
Once the task definition is created, they can be launched through the Dashboard
as well. Navigate to the Definitions tab. Select the Task you want to launch by
pressing Launch
.
On the following screen, you can define one or more Task parameters by entering:
-
Parameter Key
-
Parameter Value
Task parameters are not typed.
45.3. Executions

46. Jobs
The Jobs section of the Dashboard allows you to inspect Batch Jobs. The main section of the screen provides a list of Job Executions. Batch Jobs are Tasks that were executing one or more Batch Job. As such each Job Execution has a back reference to the Task Execution Id (Task Id).
In case of a failed job, you can also restart the task. When dealing with long-running Batch Jobs, you can also request to stop it.

46.1. List job executions
This page lists the Batch Job Executions and provides the option to restart or stop a specific job execution, provided the operation is available. Furthermore, you have the option to view the Job execution details.
The list of Job Executions also shows the state of the underlying Job Definition. Thus, if the underlying definition has been deleted, deleted will be shown.
46.1.1. Job execution details
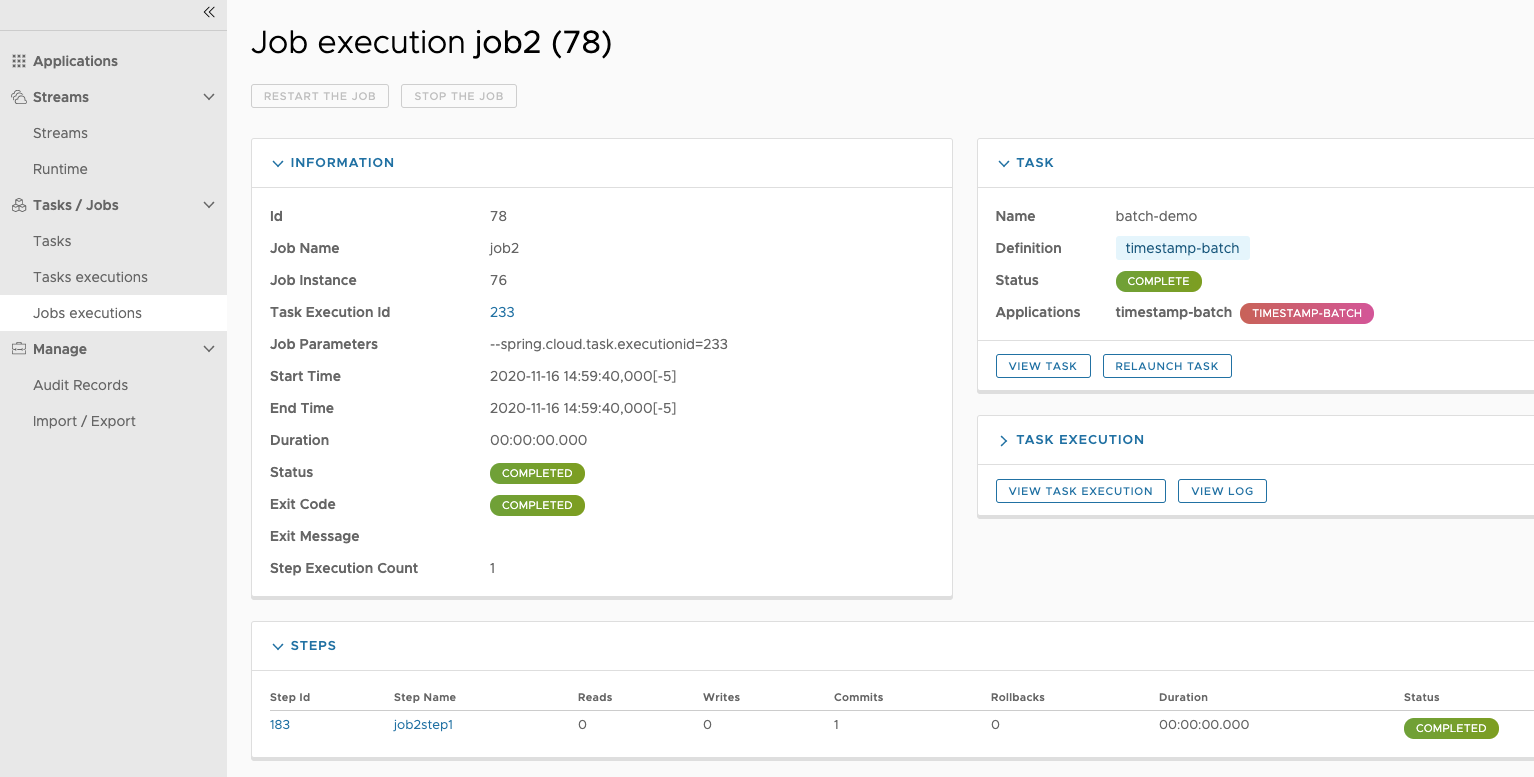
The Job Execution Details screen also contains a list of the executed steps. You can further drill into the Step Execution Details by clicking onto the magnifying glass.
46.1.2. Step execution details
On the top of the page, you will see progress indicator the respective step, with the option to refresh the indicator. Furthermore, a link is provided to view the step execution history.
The Step Execution details screen provides a complete list of all Step Execution Context key/value pairs.
In case of exceptions, the Exit Description field will contain additional error information. Please be aware, though, that this field can only have a maximum of 2500 characters. Therefore, in case of long exception stacktraces, trimming of error messages may occur. In that case, please refer to the server log files for further details. |
46.1.3. Step Execution Progress
On this screen, you can see a progress bar indicator in regards to the execution of the current step. Under the Step Execution History, you can also view various metrics associated with the selected step such as duration, read counts, write counts etc.
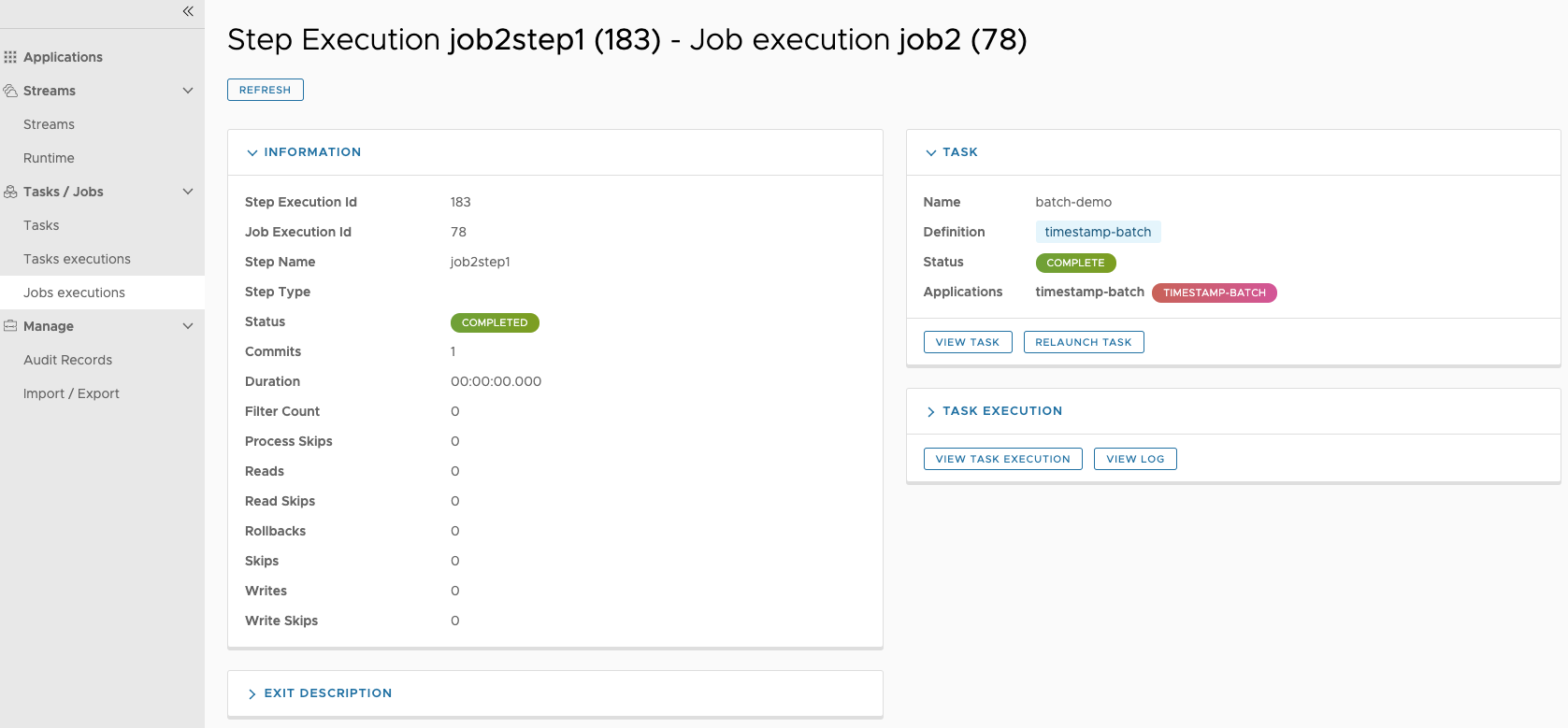
47. Analytics
The Analytics section of the Dashboard provided data visualization capabilities for the various analytics applications available in Spring Cloud Data Flow:
-
Counters
-
Field-Value Counters
-
Aggregate Counters
For example, if you create a stream with a Counter application, you can now easily create the corresponding graph from within the Dashboard tab:
-
Under
Metric Type
, selectCounters
from the select box -
Under
Stream
, selecttweetcount
-
Under
Visualization
, select the desired chart option,Bar Chart
Using the icons to the right, you can add additional charts to the Dashboard, re-arange the order of created dashboards or remove data visualizations.
‘How-to’ guides
This section provides answers to some common ‘how do I do that…’ type of questions that often arise when using Spring Cloud Data Flow.
If you are having a specific problem that we don’t cover here, you might want to check out
stackoverflow.com to see if someone has
already provided an answer; this is also a great place to ask new questions (please use
the spring-cloud-dataflow
tag).
We’re also more than happy to extend this section; If you want to add a ‘how-to’ you can send us a pull request.
48. Configure Maven Properties
You can set the maven properties such as local maven repository location, remote maven repositories and their authentication credentials including
the proxy server properties via commandline properties when starting the Dataflow server or using the SPRING_APPLICATION_JSON
environment property
for the Dataflow server.
The remote maven repositories need to be configured explicitly if the apps are resolved using maven repository except for local
Data Flow server. The other
Data Flow server implementations (that use maven resources for app artifacts resolution) have no default value for remote repositories.
The local
server has repo.spring.io/libs-snapshot
as the default remote repository.
To pass the properties as commandline options:
$ java -jar <dataflow-server>.jar --maven.localRepository=mylocal
--maven.remote-repositories.repo1.url=https://repo1
--maven.remote-repositories.repo1.auth.username=repo1user
--maven.remote-repositories.repo1.auth.password=repo1pass
--maven.remote-repositories.repo2.url=https://repo2 --maven.proxy.host=proxyhost
--maven.proxy.port=9018 --maven.proxy.auth.username=proxyuser
--maven.proxy.auth.password=proxypass
or, using the SPRING_APPLICATION_JSON
environment property:
export SPRING_APPLICATION_JSON='{ "maven": { "local-repository": "local","remote-repositories": { "repo1": { "url": "https://repo1", "auth": { "username": "repo1user", "password": "repo1pass" } },
"repo2": { "url": "https://repo2" } }, "proxy": { "host": "proxyhost", "port": 9018, "auth": { "username": "proxyuser", "password": "proxypass" } } } }'
Formatted JSON:
SPRING_APPLICATION_JSON='{
"maven": {
"local-repository": "local",
"remote-repositories": {
"repo1": {
"url": "https://repo1",
"auth": {
"username": "repo1user",
"password": "repo1pass"
}
},
"repo2": {
"url": "https://repo2"
}
},
"proxy": {
"host": "proxyhost",
"port": 9018,
"auth": {
"username": "proxyuser",
"password": "proxypass"
}
}
}
}'
Depending on Spring Cloud Data Flow server implementation, you may have to pass the
environment properties using the platform specific environment-setting capabilities. For instance,
in Cloud Foundry, you’d be passing them as cf set-env SPRING_APPLICATION_JSON .
|
49. Logging
Spring Cloud Data Flow is built upon several Spring projects, but ultimately the dataflow-server is a Spring Boot app, so the logging techniques that apply to any Spring Boot application are applicable here as well.
While troubleshooting, following are the two primary areas where enabling the DEBUG logs could be useful.
49.1. Deployment Logs
Spring Cloud Data Flow builds upon Spring Cloud Deployer SPI and the platform specific dataflow-server uses the respective SPI implementations. Specifically, if we were to troubleshoot deployment specific issues; such as the network errors, it’d be useful to enable the DEBUG logs at the underlying deployer and the libraries used by it.
-
For instance, if you’d like to enable DEBUG logs for the local-deployer, you’d be starting the server with following.
$ java -jar <dataflow-server>.jar --logging.level.org.springframework.cloud.deployer.spi.local=DEBUG
(where,
org.springframework.cloud.deployer.spi.local
is the global package for everything local-deployer related) -
For instance, if you’d like to enable DEBUG logs for the cloudfoundry-deployer, you’d be setting the following environment variable and upon restaging the dataflow-server, we will see more logs around request, response and the elaborate stack traces (upon failures). The cloudfoundry-deployer uses cf-java-client, so we will have to enable DEBUG logs for this library.
$ cf set-env dataflow-server JAVA_OPTS '-Dlogging.level.cloudfoundry-client=DEBUG' $ cf restage dataflow-server
(where,
cloudfoundry-client
is the global package for everythingcf-java-client
related) -
If there’s a need to review Reactor logs, which is used by the
cf-java-client
, then the following would be helpful.$ cf set-env dataflow-server JAVA_OPTS '-Dlogging.level.cloudfoundry-client=DEBUG -Dlogging.level.reactor.ipc.netty=DEBUG' $ cf restage dataflow-server
(where,
reactor.ipc.netty
is the global package for everythingreactor-netty
related)
Similar to the local-deployer and cloudfoundry-deployer options as discussed above, there
are equivalent settings available for Apache YARN, Apache Mesos and Kubernetes variants, too. Check out the
respective SPI implementations to
find out more details about the packages to configure for logging.
|
49.2. Application Logs
The streaming applications in Spring Cloud Data Flow are Spring Boot applications and they can be independently setup with logging configurations.
For instance, if you’d have to troubleshoot the header
and payload
specifics that are being passed
around source, processor and sink channels, you’d be deploying the stream with the following
options.
dataflow:>stream create foo --definition "http --logging.level.org.springframework.integration=DEBUG | transform --logging.level.org.springframework.integration=DEBUG | log --logging.level.org.springframework.integration=DEBUG" --deploy
(where, org.springframework.integration
is the global package for everything Spring Integration related,
which is responsible for messaging channels)
These properties can also be specified via deployment
properties when deploying the stream.
dataflow:>stream deploy foo --properties "app.*.logging.level.org.springframework.integration=DEBUG"
50. Frequently asked questions
In this section, we will review the frequently discussed questions in Spring Cloud Data Flow.
50.1. Advanced SpEL expressions
One of the powerful features of SpEL expressions is functions.
Spring Integration provides jsonPath()
and xpath()
out-of-the-box SpEL-functions, if appropriate libraries are in the classpath.
All the provided Spring Cloud Stream application starters are supplied with the json-path
and spring-integration-xml
jars, thus we can use those SpEL-functions in Spring Cloud Data Flow streams whenever expressions are possible.
For example we can transform JSON-aware payload
from the HTTP request using some jsonPath()
expression:
dataflow:>stream create jsonPathTransform --definition "http | transform --expression=#jsonPath(payload,'$.price') | log" --deploy
...
dataflow:> http post --target http://localhost:8080 --data {"symbol":"SCDF","price":72.04}
dataflow:> http post --target http://localhost:8080 --data {"symbol":"SCDF","price":72.06}
dataflow:> http post --target http://localhost:8080 --data {"symbol":"SCDF","price":72.08}
In this sample we apply jsonPath for the incoming payload to extract just only the price
field value.
Similar syntax can be used with splitter
or filter
expression
options.
Actually any available SpEL-based option has access to the built-in SpEL-functions.
For example we can extract some value from JSON data to calculate the partitionKey
before sending output to the Binder:
dataflow:>stream deploy foo --properties "deployer.transform.count=2,app.transform.producer.partitionKeyExpression=#jsonPath(payload,'$.symbol')"
The same syntax can be applied for xpath()
SpEL-function when you deal with XML data.
Any other custom SpEL-function can also be used, but for this purpose you should build a library with the @Configuration
class containing an appropriate SpelFunctionFactoryBean
@Bean
definition.
The target Spring Cloud Stream application starter should be re-packaged to supply such a custom extension via built-in Spring Boot @ComponentScan
mechanism or auto-configuration hook.
50.2. How to use JDBC-sink?
The JDBC-sink can be used to insert message payload data into a relational database table. By default,
it inserts the entire payload into a table named after the jdbc.table-name
property, and if it is not set,
by default the application expects to use a table with the name messages
. To alter this behavior, the
JDBC sink accepts several options that you can pass using the --foo=bar notation in the stream, or change globally.
The JDBC sink has a jdbc.initialize
property that if set to true
will result in the sink creating a table based on the specified configuration when the it starts up. If that initialize property is false
, which is the default, you will have to make sure that the table to use is already available.
A stream definition using jdbc
sink relying on all defaults with MySQL as the backing database looks
like the following. In this example, the system time is persisted in MySQL for every second.
dataflow:>stream create --name mydata --definition "time | jdbc --spring.datasource.url=jdbc:mysql://localhost:3306/test --spring.datasource.username=root --spring.datasource.password=root --spring.datasource.driver-class-name=org.mariadb.jdbc.Driver" --deploy
For this to work, you’d have to have the following table in the MySQL database.
CREATE TABLE test.messages
(
payload varchar(255)
);
mysql> desc test.messages;
+---------+--------------+------+-----+---------+-------+
| Field | Type | Null | Key | Default | Extra |
+---------+--------------+------+-----+---------+-------+
| payload | varchar(255) | YES | | NULL | |
+---------+--------------+------+-----+---------+-------+
1 row in set (0.00 sec)
mysql> select * from test.messages;
+-------------------+
| payload |
+-------------------+
| 04/25/17 09:10:04 |
| 04/25/17 09:10:06 |
| 04/25/17 09:10:07 |
| 04/25/17 09:10:08 |
| 04/25/17 09:10:09 |
.............
.............
.............
50.3. How to use multiple message-binders?
For situations where the data is consumed and processed between two different message brokers, Spring
Cloud Data Flow provides easy to override global configurations, out-of-the-box bridge-processor
,
and DSL primitives to build these type of topologies.
Let’s assume we have data queueing up in RabbitMQ (e.g., queue = fooRabbit
) and the requirement
is to consume all the payloads and publish them to Apache Kafka (e.g., topic = barKafka
), as the
destination for downstream processing.
Follow the global application of configurations to define multiple binder configurations.
# Apache Kafka Global Configurations (i.e., identified by "kafka1")
spring.cloud.dataflow.applicationProperties.stream.spring.cloud.stream.binders.kafka1.type=kafka
spring.cloud.dataflow.applicationProperties.stream.spring.cloud.stream.binders.kafka1.environment.spring.cloud.stream.kafka.binder.brokers=localhost:9092
spring.cloud.dataflow.applicationProperties.stream.spring.cloud.stream.binders.kafka1.environment.spring.cloud.stream.kafka.binder.zkNodes=localhost:2181
# RabbitMQ Global Configurations (i.e., identified by "rabbit1")
spring.cloud.dataflow.applicationProperties.stream.spring.cloud.stream.binders.rabbit1.type=rabbit
spring.cloud.dataflow.applicationProperties.stream.spring.cloud.stream.binders.rabbit1.environment.spring.rabbitmq.host=localhost
spring.cloud.dataflow.applicationProperties.stream.spring.cloud.stream.binders.rabbit1.environment.spring.rabbitmq.port=5672
In this example, both the message brokers are running locally and reachable at localhost
with respective ports.
|
These properties can be supplied in a ".properties" file that is accessible to the server directly or via
config-server
.
java -jar spring-cloud-dataflow-server-local/target/spring-cloud-dataflow-server-local-1.1.4.RELEASE.jar --spring.config.location=<PATH-TO-FILE>/foo.properties
Spring Cloud Data Flow internally uses bridge-processor
to directly connect different named channel
destinations. Since we are publishing and subscribing from two different messaging systems, you’d have
to build the bridge-processor
with both RabbitMQ and Apache Kafka binders in the classpath. To do that,
head over to start-scs.cfapps.io/ and select Bridge Processor
, Kafka binder starter
, and
Rabbit binder starter
as the dependencies and follow the patching procedure described in the
reference guide.
Specifically, for the bridge-processor
, you’d have to import the BridgeProcessorConfiguration
provided by the starter.
Once you have the necessary adjustments, you can build the application. Let’s register the name of the
application as multiBinderBridge
.
dataflow:>app register --type processor --name multiBinderBridge --uri file:///<PATH-TO-FILE>/multipleBinderBridge-0.0.1-SNAPSHOT.jar
It is time to create a stream definition with the newly registered processor application.
dataflow:>stream create fooRabbitToBarKafka --definition ":fooRabbit > multiBinderBridge --spring.cloud.stream.bindings.input.binder=rabbit1 --spring.cloud.stream.bindings.output.binder=kafka1 > :barKafka" --deploy
Since we are to consume messages from RabbitMQ (i.e., identified by rabbit1 ) and then
publish the payload to Apache Kafka (i.e., identified by kafka1 ), we are supplying them as input
and output channel settings respectively.
|
The queue fooRabbit in RabbitMQ is where the stream is consuming events from and the topic
barKafka in Apache Kafka is where the data is finally landing.
|
REST API Guide
In this section you will learn all about the Spring Cloud Data Flow REST API.
51. Overview
Spring Cloud Data Flow provides a REST API allowing you to access all aspects of the server. In fact the Spring Cloud Data Flow Shell is a first-class consumer of that API.
If you plan on using the REST API using Java, please also consider using the provided Java client (DataflowTemplate) that uses the REST API internally. |
51.1. HTTP verbs
Spring Cloud Data Flow tries to adhere as closely as possible to standard HTTP and REST conventions in its use of HTTP verbs.
Verb | Usage |
---|---|
|
Used to retrieve a resource |
|
Used to create a new resource |
|
Used to update an existing resource, including partial updates. Also used for
resources that imply the concept of |
|
Used to delete an existing resource |
51.2. HTTP status codes
RESTful note tries to adhere as closely as possible to standard HTTP and REST conventions in its use of HTTP status codes.
Status code | Usage |
---|---|
|
The request completed successfully |
|
A new resource has been created successfully. The resource’s URI is available from the response’s |
|
An update to an existing resource has been applied successfully |
|
The request was malformed. The response body will include an error providing further information |
|
The requested resource did not exist |
|
The requested resource already exists, e.g. the task already exists or the stream was already being deployed |
|
Returned in cases the Job Execution cannot be stopped or restarted |
51.3. Headers
Every response has the following header(s):
Name | Description |
---|---|
|
The Content-Type of the payload, e.g. |
51.4. Errors
Path | Type | Description |
---|---|---|
|
|
The HTTP error that occurred, e.g. |
|
|
A description of the cause of the error |
|
|
The path to which the request was made |
|
|
The HTTP status code, e.g. |
|
|
The time, in milliseconds, at which the error occurred |
51.5. Hypermedia
Spring Cloud Data Flow uses hypermedia and resources include links to other resources
in their responses. Responses are in Hypertext Application from resource to resource Language (HAL) format. Links can be found beneath the _links
key. Users of the API should not create URIs themselves, instead they should use the above-described links to navigate.
52. Resources
52.1. Index
The index provides the entry point into Spring Cloud Data Flow’s REST API.
52.1.1. Accessing the index
A GET
request is used to access the index
Request structure
GET / HTTP/1.1
Host: localhost:9393
Example request
$ curl 'http://localhost:9393/' -i
Response structure
Path | Type | Description |
---|---|---|
|
|
Links to other resources |
|
|
Incremented each time a change is implemented in this REST API |
Example response
HTTP/1.1 200 OK
Content-Type: application/hal+json;charset=UTF-8
Content-Length: 4030
{
"_links" : {
"dashboard" : {
"href" : "http://localhost:9393/dashboard"
},
"streams/definitions" : {
"href" : "http://localhost:9393/streams/definitions"
},
"streams/definitions/definition" : {
"href" : "http://localhost:9393/streams/definitions/{name}",
"templated" : true
},
"streams/deployments" : {
"href" : "http://localhost:9393/streams/deployments"
},
"streams/deployments/deployment" : {
"href" : "http://localhost:9393/streams/deployments/{name}",
"templated" : true
},
"runtime/apps" : {
"href" : "http://localhost:9393/runtime/apps"
},
"runtime/apps/app" : {
"href" : "http://localhost:9393/runtime/apps/{appId}",
"templated" : true
},
"runtime/apps/instances" : {
"href" : "http://localhost:9393/runtime/apps/interface%20org.springframework.web.util.UriComponents%24UriTemplateVariables/instances"
},
"metrics/streams" : {
"href" : "http://localhost:9393/metrics/streams"
},
"tasks/definitions" : {
"href" : "http://localhost:9393/tasks/definitions"
},
"tasks/definitions/definition" : {
"href" : "http://localhost:9393/tasks/definitions/{name}",
"templated" : true
},
"tasks/executions" : {
"href" : "http://localhost:9393/tasks/executions"
},
"tasks/executions/name" : {
"href" : "http://localhost:9393/tasks/executions{?name}",
"templated" : true
},
"tasks/executions/execution" : {
"href" : "http://localhost:9393/tasks/executions/{id}",
"templated" : true
},
"jobs/executions" : {
"href" : "http://localhost:9393/jobs/executions"
},
"jobs/executions/name" : {
"href" : "http://localhost:9393/jobs/executions{?name}",
"templated" : true
},
"jobs/executions/execution" : {
"href" : "http://localhost:9393/jobs/executions/{id}",
"templated" : true
},
"jobs/executions/execution/steps" : {
"href" : "http://localhost:9393/jobs/executions/{jobExecutionId}/steps",
"templated" : true
},
"jobs/executions/execution/steps/step" : {
"href" : "http://localhost:9393/jobs/executions/{jobExecutionId}/steps/{stepId}",
"templated" : true
},
"jobs/executions/execution/steps/step/progress" : {
"href" : "http://localhost:9393/jobs/executions/{jobExecutionId}/steps/{stepId}/progress",
"templated" : true
},
"jobs/instances/name" : {
"href" : "http://localhost:9393/jobs/instances{?name}",
"templated" : true
},
"jobs/instances/instance" : {
"href" : "http://localhost:9393/jobs/instances/{id}",
"templated" : true
},
"tools/parseTaskTextToGraph" : {
"href" : "http://localhost:9393/tools"
},
"tools/convertTaskGraphToText" : {
"href" : "http://localhost:9393/tools"
},
"counters" : {
"href" : "http://localhost:9393/metrics/counters"
},
"counters/counter" : {
"href" : "http://localhost:9393/metrics/counters/{name}",
"templated" : true
},
"field-value-counters" : {
"href" : "http://localhost:9393/metrics/field-value-counters"
},
"field-value-counters/counter" : {
"href" : "http://localhost:9393/metrics/field-value-counters/{name}",
"templated" : true
},
"aggregate-counters" : {
"href" : "http://localhost:9393/metrics/aggregate-counters"
},
"aggregate-counters/counter" : {
"href" : "http://localhost:9393/metrics/aggregate-counters/{name}",
"templated" : true
},
"apps" : {
"href" : "http://localhost:9393/apps"
},
"about" : {
"href" : "http://localhost:9393/about"
},
"completions/stream" : {
"href" : "http://localhost:9393/completions/stream{?start,detailLevel}",
"templated" : true
},
"completions/task" : {
"href" : "http://localhost:9393/completions/task{?start,detailLevel}",
"templated" : true
}
},
"api.revision" : 14
}
Links
The main element of the index are the links as they allow you to traverse the API and execute the desired functionality:
Relation | Description |
---|---|
|
Access meta information, including enabled features, security info, version information |
|
Access the dashboard UI |
|
Handle registered applications |
|
Exposes the DSL completion features for Stream |
|
Exposes the DSL completion features for Task |
|
Exposes metrics for the stream applications |
|
Provides the JobExecution resource |
|
Provides details for a specific JobExecution |
|
Provides the steps for a JobExecution |
|
Returns the details for a specific step |
|
Provides progress information for a specific step |
|
Retrieve Job Executions by Job name |
|
Provides the job instance resource for a specific job instance |
|
Provides the Job instance resource for a specific job name |
|
Provides the runtime application resource |
|
Exposes the runtime status for a specific app |
|
Provides the status for app instances |
|
Provides the task definition resource |
|
Provides details for a specific task definition |
|
Returns Task executions and allows lanching of tasks |
|
Returns all task executions for a given Task name |
|
Provides details for a specific task execution |
|
Exposes the Streams resource |
|
Handle a specific Stream definition |
|
Provides Stream deployment operations |
|
Request (un-)deployment of an existing stream definition |
|
Exposes the resource for dealing with Counters |
|
Handle a specific counter |
|
Provides the resource for dealing with aggregate counters |
|
Handle a specific aggregate counter |
|
Provides the resource for dealing with field-value-counters |
|
Handle a specific field-value-counter |
|
Parse a task definition into a graph structure |
|
Convert a graph format into DSL text format |
52.2. Server Meta Information
52.2.1. Retrieving information about the server
A GET
request will return meta information for Spring Cloud Data Flow. This includes:
-
Runtime Environment Information
-
Information regarding which features are enabled
-
Dependency information of Spring Cloud Data Flow Server
-
Security information
Request structure
GET /about HTTP/1.1
Accept: application/json
Host: localhost:9393
Example request
$ curl 'http://localhost:9393/about' -i -H 'Accept: application/json'
Response structure
HTTP/1.1 200 OK
Content-Type: application/json;charset=UTF-8
Content-Length: 1815
{
"featureInfo" : {
"analyticsEnabled" : true,
"streamsEnabled" : true,
"tasksEnabled" : true
},
"versionInfo" : {
"implementation" : {
"name" : "spring-cloud-starter-dataflow-server-local",
"version" : "1.2.0.RC2"
},
"core" : {
"name" : "Spring Cloud Data Flow Core",
"version" : "1.2.0.RC2"
},
"dashboard" : {
"name" : "Spring Cloud Dataflow UI",
"version" : "1.2.0.RELEASE"
}
},
"securityInfo" : {
"authenticationEnabled" : false,
"authorizationEnabled" : false,
"formLogin" : false,
"authenticated" : false,
"username" : null,
"roles" : [ ]
},
"runtimeEnvironment" : {
"appDeployer" : {
"deployerImplementationVersion" : "1.2.0.RELEASE",
"deployerName" : "LocalAppDeployer",
"deployerSpiVersion" : "1.2.0.RELEASE",
"javaVersion" : "1.8.0_121",
"platformApiVersion" : "Linux 4.4.0-75-generic",
"platformClientVersion" : "4.4.0-75-generic",
"platformHostVersion" : "4.4.0-75-generic",
"platformSpecificInfo" : { },
"platformType" : "Local",
"springBootVersion" : "1.5.2.RELEASE",
"springVersion" : "4.3.7.RELEASE"
},
"taskLauncher" : {
"deployerImplementationVersion" : "1.2.0.RELEASE",
"deployerName" : "LocalTaskLauncher",
"deployerSpiVersion" : "1.2.0.RELEASE",
"javaVersion" : "1.8.0_121",
"platformApiVersion" : "Linux 4.4.0-75-generic",
"platformClientVersion" : "4.4.0-75-generic",
"platformHostVersion" : "4.4.0-75-generic",
"platformSpecificInfo" : { },
"platformType" : "Local",
"springBootVersion" : "1.5.2.RELEASE",
"springVersion" : "4.3.7.RELEASE"
}
},
"_links" : {
"self" : {
"href" : "http://localhost:9393/about"
}
}
}
52.3. Registered Applications
52.3.1. Listing Applications
A GET
request will list all applications known to Spring Cloud Data Flow.
Request structure
GET /apps?type=source HTTP/1.1
Accept: application/json
Host: localhost:9393
Request parameters
Parameter | Description |
---|---|
|
Restrict the returned apps to the type of the app. |
Example request
$ curl 'http://localhost:9393/apps?type=source' -i -H 'Accept: application/json'
Response structure
HTTP/1.1 200 OK
Content-Type: application/json;charset=UTF-8
Content-Length: 185
{
"_links" : {
"self" : {
"href" : "http://localhost:9393/apps"
}
},
"page" : {
"size" : 0,
"totalElements" : 0,
"totalPages" : 1,
"number" : 0
}
}
52.4. Stream Definitions
52.4.1. Example "stream create" request for a ticktock
stream
curl -X POST -d "name=ticktock&definition=time | log" localhost:9393/streams/definitions?deploy=false
52.5. Stream Deployments
52.5.1. Example "stream deploy" request for a ticktock
stream
curl -X POST http://localhost:9393/streams/deployments/ticktock
52.6. Task Definitions
52.6.1. Creating a new Task Definition
Creating a task definition is achieved by POSTing to the task definitions endpoint.
Request structure
POST /tasks/definitions HTTP/1.1
Host: localhost:9393
Content-Type: application/x-www-form-urlencoded
name=my-task&definition=timestamp+--format%3D%27YYYY+MM+DD%27
Request parameters
Parameter | Description |
---|---|
|
The name for the created task definitions |
|
The definition for the task, using Data Flow DSL |
Example request
$ curl 'http://localhost:9393/tasks/definitions' -i -X POST -d 'name=my-task&definition=timestamp+--format%3D%27YYYY+MM+DD%27'
Response structure
HTTP/1.1 200 OK
Content-Type: application/hal+json;charset=UTF-8
Content-Length: 225
{
"name" : "my-task",
"dslText" : "timestamp --format='YYYY MM DD'",
"composed" : false,
"status" : "unknown",
"_links" : {
"self" : {
"href" : "http://localhost:9393/tasks/definitions/my-task"
}
}
}
52.7. Task Launch
52.7.1. Launching a Task
Launching a task is done by requesting the creation of a new Task Execution.
Request structure
POST /tasks/executions HTTP/1.1
Host: localhost:9393
Content-Type: application/x-www-form-urlencoded
name=my-task&properties=app.my-task.foo%3Dbar%2Cdeployer.my-task.something-else%3D3&arguments=--server.port%3D8080%2C--foo%3Dbar
Request parameters
Parameter | Description |
---|---|
|
The name of the task definition to launch |
|
Application and Deployer properties to use while launching |
|
Command line arguments to pass to the task |
Example request
$ curl 'http://localhost:9393/tasks/executions' -i -X POST -d 'name=my-task&properties=app.my-task.foo%3Dbar%2Cdeployer.my-task.something-else%3D3&arguments=--server.port%3D8080%2C--foo%3Dbar'
Response structure
HTTP/1.1 201 Created
Content-Type: application/json;charset=UTF-8
Content-Length: 1
1
52.8. Metrics for Stream Applications
This REST endpoint exposes metrics for stream applications. This REST enpoint requires the Metrics Collector application to be running as a separate service. If not running, this endpoint will return an empty response.
In order to learn more about the Metrics Collector, please also refer to chapter Monitoring Deployed Applications |
52.8.1. Request structure
For example, a typical request may look like:
GET /metrics/streams HTTP/1.1
Accept: application/json
Host: localhost:9393
52.8.2. Example request
$ curl 'http://localhost:9393/metrics/streams' -i -H 'Accept: application/json'
52.8.3. Response structure
This REST endpoint uses Hystrix via the Spring Cloud Netflix project under the covers to make a proxy HTTP request to the Metrics Collector.
52.8.4. Example response
Therefore, the endpoint will not generate an error in case the Metrics Collector is not running, but rather gracefully degrade and return an empty response such as the following:
HTTP/1.1 200 OK
Content-Type: application/json;charset=UTF-8
Content-Length: 3
[ ]
However, if metrics are being collected and the Metrics Collector is running you
should see a response similar to the listing below. The metrics data returned in
the listing below is based on the example stream definition created
in chapter Monitoring Deployed Applications time | log
with
two instances of each application deployed.
HTTP/1.1 200 OK
Content-Type: application/json;charset=UTF-8
Content-Length: 30240
[ {
"name" : "foostream",
"applications" : [ {
"name" : "log120RS",
"instances" : [ {
"guid" : "13208",
"index" : 1,
"properties" : {
"spring.cloud.dataflow.stream.app.label" : "log120RS",
"spring.application.index" : "1",
"spring.application.name" : "log-sink",
"spring.cloud.dataflow.stream.name" : "foostream",
"spring.cloud.application.guid" : "13208",
"spring.cloud.dataflow.stream.app.type" : "sink",
"spring.cloud.application.group" : "foostream"
},
"metrics" : [ {
"name" : "integration.channel.input.errorRate.mean",
"value" : 0.0
}, {
"name" : "integration.channel.input.errorRate.max",
"value" : 0.0
}, {
"name" : "integration.channel.input.errorRate.min",
"value" : 0.0
}, {
"name" : "integration.channel.input.errorRate.stdev",
"value" : 0.0
}, {
"name" : "integration.channel.input.errorRate.count",
"value" : 0.0
}, {
"name" : "integration.channel.input.sendCount",
"value" : 373.0
}, {
"name" : "integration.channel.input.sendRate.mean",
"value" : 1.0
}, {
"name" : "integration.channel.input.sendRate.max",
"value" : 2.01
}, {
"name" : "integration.channel.input.sendRate.min",
"value" : 0.7
}, {
"name" : "integration.channel.input.sendRate.stdev",
"value" : 0.0
}, {
"name" : "integration.channel.input.sendRate.count",
"value" : 373.0
}, {
"name" : "integration.channel.errorChannel.errorRate.mean",
"value" : 0.0
}, {
"name" : "integration.channel.errorChannel.errorRate.max",
"value" : 0.0
}, {
"name" : "integration.channel.errorChannel.errorRate.min",
"value" : 0.0
}, {
"name" : "integration.channel.errorChannel.errorRate.stdev",
"value" : 0.0
}, {
"name" : "integration.channel.errorChannel.errorRate.count",
"value" : 0.0
}, {
"name" : "integration.channel.errorChannel.sendCount",
"value" : 0.0
}, {
"name" : "integration.channel.errorChannel.sendRate.mean",
"value" : 0.0
}, {
"name" : "integration.channel.errorChannel.sendRate.max",
"value" : 0.0
}, {
"name" : "integration.channel.errorChannel.sendRate.min",
"value" : 0.0
}, {
"name" : "integration.channel.errorChannel.sendRate.stdev",
"value" : 0.0
}, {
"name" : "integration.channel.errorChannel.sendRate.count",
"value" : 0.0
}, {
"name" : "integration.channel.applicationMetrics.errorRate.mean",
"value" : 0.0
}, {
"name" : "integration.channel.applicationMetrics.errorRate.max",
"value" : 0.0
}, {
"name" : "integration.channel.applicationMetrics.errorRate.min",
"value" : 0.0
}, {
"name" : "integration.channel.applicationMetrics.errorRate.stdev",
"value" : 0.0
}, {
"name" : "integration.channel.applicationMetrics.errorRate.count",
"value" : 0.0
}, {
"name" : "integration.channel.applicationMetrics.sendCount",
"value" : 74.0
}, {
"name" : "integration.channel.applicationMetrics.sendRate.mean",
"value" : 0.2
}, {
"name" : "integration.channel.applicationMetrics.sendRate.max",
"value" : 13.49
}, {
"name" : "integration.channel.applicationMetrics.sendRate.min",
"value" : 5.0
}, {
"name" : "integration.channel.applicationMetrics.sendRate.stdev",
"value" : 0.0
}, {
"name" : "integration.channel.applicationMetrics.sendRate.count",
"value" : 74.0
}, {
"name" : "integration.channel.nullChannel.errorRate.mean",
"value" : 0.0
}, {
"name" : "integration.channel.nullChannel.errorRate.max",
"value" : 0.0
}, {
"name" : "integration.channel.nullChannel.errorRate.min",
"value" : 0.0
}, {
"name" : "integration.channel.nullChannel.errorRate.stdev",
"value" : 0.0
}, {
"name" : "integration.channel.nullChannel.errorRate.count",
"value" : 0.0
}, {
"name" : "integration.channel.nullChannel.sendCount",
"value" : 0.0
}, {
"name" : "integration.channel.nullChannel.sendRate.mean",
"value" : 0.0
}, {
"name" : "integration.channel.nullChannel.sendRate.max",
"value" : 0.0
}, {
"name" : "integration.channel.nullChannel.sendRate.min",
"value" : 0.0
}, {
"name" : "integration.channel.nullChannel.sendRate.stdev",
"value" : 0.0
}, {
"name" : "integration.channel.nullChannel.sendRate.count",
"value" : 0.0
}, {
"name" : "integration.handler.org.springframework.cloud.stream.app.log.sink.LogSinkConfiguration.logSinkHandler.serviceActivator.handler.duration.mean",
"value" : 0.22
}, {
"name" : "integration.handler.org.springframework.cloud.stream.app.log.sink.LogSinkConfiguration.logSinkHandler.serviceActivator.handler.duration.max",
"value" : 5.42
}, {
"name" : "integration.handler.org.springframework.cloud.stream.app.log.sink.LogSinkConfiguration.logSinkHandler.serviceActivator.handler.duration.min",
"value" : 0.11
}, {
"name" : "integration.handler.org.springframework.cloud.stream.app.log.sink.LogSinkConfiguration.logSinkHandler.serviceActivator.handler.duration.stdev",
"value" : 0.17
}, {
"name" : "integration.handler.org.springframework.cloud.stream.app.log.sink.LogSinkConfiguration.logSinkHandler.serviceActivator.handler.duration.count",
"value" : 373.0
}, {
"name" : "integration.handler.org.springframework.cloud.stream.app.log.sink.LogSinkConfiguration.logSinkHandler.serviceActivator.handler.activeCount",
"value" : 0.0
}, {
"name" : "integration.handler.logSinkHandler.duration.mean",
"value" : 0.22
}, {
"name" : "integration.handler.logSinkHandler.duration.max",
"value" : 5.4
}, {
"name" : "integration.handler.logSinkHandler.duration.min",
"value" : 0.11
}, {
"name" : "integration.handler.logSinkHandler.duration.stdev",
"value" : 0.17
}, {
"name" : "integration.handler.logSinkHandler.duration.count",
"value" : 373.0
}, {
"name" : "integration.handler.logSinkHandler.activeCount",
"value" : 0.0
}, {
"name" : "integration.handler._org.springframework.integration.errorLogger.handler.duration.mean",
"value" : 0.0
}, {
"name" : "integration.handler._org.springframework.integration.errorLogger.handler.duration.max",
"value" : 0.0
}, {
"name" : "integration.handler._org.springframework.integration.errorLogger.handler.duration.min",
"value" : 0.0
}, {
"name" : "integration.handler._org.springframework.integration.errorLogger.handler.duration.stdev",
"value" : 0.0
}, {
"name" : "integration.handler._org.springframework.integration.errorLogger.handler.duration.count",
"value" : 0.0
}, {
"name" : "integration.handler._org.springframework.integration.errorLogger.handler.activeCount",
"value" : 0.0
}, {
"name" : "integration.handlerCount",
"value" : 3.0
}, {
"name" : "integration.channelCount",
"value" : 4.0
}, {
"name" : "integration.sourceCount",
"value" : 0.0
}, {
"name" : "integration.channel.input.send.mean",
"value" : 1.0
}, {
"name" : "integration.channel.errorChannel.send.mean",
"value" : 0.0
}, {
"name" : "integration.channel.applicationMetrics.send.mean",
"value" : 0.2
}, {
"name" : "integration.channel.nullChannel.send.mean",
"value" : 0.0
} ]
}, {
"guid" : "60633",
"index" : 0,
"properties" : {
"spring.cloud.dataflow.stream.app.label" : "log120RS",
"spring.application.index" : "0",
"spring.application.name" : "log-sink",
"spring.cloud.dataflow.stream.name" : "foostream",
"spring.cloud.application.guid" : "60633",
"spring.cloud.dataflow.stream.app.type" : "sink",
"spring.cloud.application.group" : "foostream"
},
"metrics" : [ {
"name" : "integration.channel.input.errorRate.mean",
"value" : 0.0
}, {
"name" : "integration.channel.input.errorRate.max",
"value" : 0.0
}, {
"name" : "integration.channel.input.errorRate.min",
"value" : 0.0
}, {
"name" : "integration.channel.input.errorRate.stdev",
"value" : 0.0
}, {
"name" : "integration.channel.input.errorRate.count",
"value" : 0.0
}, {
"name" : "integration.channel.input.sendCount",
"value" : 372.0
}, {
"name" : "integration.channel.input.sendRate.mean",
"value" : 1.0
}, {
"name" : "integration.channel.input.sendRate.max",
"value" : 1.98
}, {
"name" : "integration.channel.input.sendRate.min",
"value" : 0.8
}, {
"name" : "integration.channel.input.sendRate.stdev",
"value" : 0.0
}, {
"name" : "integration.channel.input.sendRate.count",
"value" : 372.0
}, {
"name" : "integration.channel.errorChannel.errorRate.mean",
"value" : 0.0
}, {
"name" : "integration.channel.errorChannel.errorRate.max",
"value" : 0.0
}, {
"name" : "integration.channel.errorChannel.errorRate.min",
"value" : 0.0
}, {
"name" : "integration.channel.errorChannel.errorRate.stdev",
"value" : 0.0
}, {
"name" : "integration.channel.errorChannel.errorRate.count",
"value" : 0.0
}, {
"name" : "integration.channel.errorChannel.sendCount",
"value" : 0.0
}, {
"name" : "integration.channel.errorChannel.sendRate.mean",
"value" : 0.0
}, {
"name" : "integration.channel.errorChannel.sendRate.max",
"value" : 0.0
}, {
"name" : "integration.channel.errorChannel.sendRate.min",
"value" : 0.0
}, {
"name" : "integration.channel.errorChannel.sendRate.stdev",
"value" : 0.0
}, {
"name" : "integration.channel.errorChannel.sendRate.count",
"value" : 0.0
}, {
"name" : "integration.channel.applicationMetrics.errorRate.mean",
"value" : 0.0
}, {
"name" : "integration.channel.applicationMetrics.errorRate.max",
"value" : 0.0
}, {
"name" : "integration.channel.applicationMetrics.errorRate.min",
"value" : 0.0
}, {
"name" : "integration.channel.applicationMetrics.errorRate.stdev",
"value" : 0.0
}, {
"name" : "integration.channel.applicationMetrics.errorRate.count",
"value" : 0.0
}, {
"name" : "integration.channel.applicationMetrics.sendCount",
"value" : 74.0
}, {
"name" : "integration.channel.applicationMetrics.sendRate.mean",
"value" : 0.2
}, {
"name" : "integration.channel.applicationMetrics.sendRate.max",
"value" : 13.09
}, {
"name" : "integration.channel.applicationMetrics.sendRate.min",
"value" : 5.0
}, {
"name" : "integration.channel.applicationMetrics.sendRate.stdev",
"value" : 0.0
}, {
"name" : "integration.channel.applicationMetrics.sendRate.count",
"value" : 74.0
}, {
"name" : "integration.channel.nullChannel.errorRate.mean",
"value" : 0.0
}, {
"name" : "integration.channel.nullChannel.errorRate.max",
"value" : 0.0
}, {
"name" : "integration.channel.nullChannel.errorRate.min",
"value" : 0.0
}, {
"name" : "integration.channel.nullChannel.errorRate.stdev",
"value" : 0.0
}, {
"name" : "integration.channel.nullChannel.errorRate.count",
"value" : 0.0
}, {
"name" : "integration.channel.nullChannel.sendCount",
"value" : 0.0
}, {
"name" : "integration.channel.nullChannel.sendRate.mean",
"value" : 0.0
}, {
"name" : "integration.channel.nullChannel.sendRate.max",
"value" : 0.0
}, {
"name" : "integration.channel.nullChannel.sendRate.min",
"value" : 0.0
}, {
"name" : "integration.channel.nullChannel.sendRate.stdev",
"value" : 0.0
}, {
"name" : "integration.channel.nullChannel.sendRate.count",
"value" : 0.0
}, {
"name" : "integration.handler.org.springframework.cloud.stream.app.log.sink.LogSinkConfiguration.logSinkHandler.serviceActivator.handler.duration.mean",
"value" : 0.22
}, {
"name" : "integration.handler.org.springframework.cloud.stream.app.log.sink.LogSinkConfiguration.logSinkHandler.serviceActivator.handler.duration.max",
"value" : 3.46
}, {
"name" : "integration.handler.org.springframework.cloud.stream.app.log.sink.LogSinkConfiguration.logSinkHandler.serviceActivator.handler.duration.min",
"value" : 0.12
}, {
"name" : "integration.handler.org.springframework.cloud.stream.app.log.sink.LogSinkConfiguration.logSinkHandler.serviceActivator.handler.duration.stdev",
"value" : 0.18
}, {
"name" : "integration.handler.org.springframework.cloud.stream.app.log.sink.LogSinkConfiguration.logSinkHandler.serviceActivator.handler.duration.count",
"value" : 372.0
}, {
"name" : "integration.handler.org.springframework.cloud.stream.app.log.sink.LogSinkConfiguration.logSinkHandler.serviceActivator.handler.activeCount",
"value" : 0.0
}, {
"name" : "integration.handler.logSinkHandler.duration.mean",
"value" : 0.21
}, {
"name" : "integration.handler.logSinkHandler.duration.max",
"value" : 2.84
}, {
"name" : "integration.handler.logSinkHandler.duration.min",
"value" : 0.11
}, {
"name" : "integration.handler.logSinkHandler.duration.stdev",
"value" : 0.18
}, {
"name" : "integration.handler.logSinkHandler.duration.count",
"value" : 372.0
}, {
"name" : "integration.handler.logSinkHandler.activeCount",
"value" : 0.0
}, {
"name" : "integration.handler._org.springframework.integration.errorLogger.handler.duration.mean",
"value" : 0.0
}, {
"name" : "integration.handler._org.springframework.integration.errorLogger.handler.duration.max",
"value" : 0.0
}, {
"name" : "integration.handler._org.springframework.integration.errorLogger.handler.duration.min",
"value" : 0.0
}, {
"name" : "integration.handler._org.springframework.integration.errorLogger.handler.duration.stdev",
"value" : 0.0
}, {
"name" : "integration.handler._org.springframework.integration.errorLogger.handler.duration.count",
"value" : 0.0
}, {
"name" : "integration.handler._org.springframework.integration.errorLogger.handler.activeCount",
"value" : 0.0
}, {
"name" : "integration.handlerCount",
"value" : 3.0
}, {
"name" : "integration.channelCount",
"value" : 4.0
}, {
"name" : "integration.sourceCount",
"value" : 0.0
}, {
"name" : "integration.channel.input.send.mean",
"value" : 1.0
}, {
"name" : "integration.channel.errorChannel.send.mean",
"value" : 0.0
}, {
"name" : "integration.channel.applicationMetrics.send.mean",
"value" : 0.2
}, {
"name" : "integration.channel.nullChannel.send.mean",
"value" : 0.0
} ]
} ],
"aggregateMetrics" : [ {
"name" : "integration.channel.nullChannel.send.mean",
"value" : 0.0
}, {
"name" : "integration.channel.applicationMetrics.send.mean",
"value" : 0.4
}, {
"name" : "integration.channel.errorChannel.send.mean",
"value" : 0.0
}, {
"name" : "integration.channel.input.send.mean",
"value" : 2.0
} ]
}, {
"name" : "time120RS",
"instances" : [ {
"guid" : "50467",
"index" : 0,
"properties" : {
"spring.cloud.dataflow.stream.app.label" : "time120RS",
"spring.application.index" : "0",
"spring.application.name" : "time-source",
"spring.cloud.dataflow.stream.name" : "foostream",
"spring.cloud.application.guid" : "50467",
"spring.cloud.dataflow.stream.app.type" : "source",
"spring.cloud.application.group" : "foostream"
},
"metrics" : [ {
"name" : "integration.channel.output.errorRate.mean",
"value" : 0.0
}, {
"name" : "integration.channel.output.errorRate.max",
"value" : 0.0
}, {
"name" : "integration.channel.output.errorRate.min",
"value" : 0.0
}, {
"name" : "integration.channel.output.errorRate.stdev",
"value" : 0.0
}, {
"name" : "integration.channel.output.errorRate.count",
"value" : 0.0
}, {
"name" : "integration.channel.output.sendCount",
"value" : 369.0
}, {
"name" : "integration.channel.output.sendRate.mean",
"value" : 1.0
}, {
"name" : "integration.channel.output.sendRate.max",
"value" : 1.02
}, {
"name" : "integration.channel.output.sendRate.min",
"value" : 1.0
}, {
"name" : "integration.channel.output.sendRate.stdev",
"value" : 0.0
}, {
"name" : "integration.channel.output.sendRate.count",
"value" : 369.0
}, {
"name" : "integration.channel.errorChannel.errorRate.mean",
"value" : 0.0
}, {
"name" : "integration.channel.errorChannel.errorRate.max",
"value" : 0.0
}, {
"name" : "integration.channel.errorChannel.errorRate.min",
"value" : 0.0
}, {
"name" : "integration.channel.errorChannel.errorRate.stdev",
"value" : 0.0
}, {
"name" : "integration.channel.errorChannel.errorRate.count",
"value" : 0.0
}, {
"name" : "integration.channel.errorChannel.sendCount",
"value" : 0.0
}, {
"name" : "integration.channel.errorChannel.sendRate.mean",
"value" : 0.0
}, {
"name" : "integration.channel.errorChannel.sendRate.max",
"value" : 0.0
}, {
"name" : "integration.channel.errorChannel.sendRate.min",
"value" : 0.0
}, {
"name" : "integration.channel.errorChannel.sendRate.stdev",
"value" : 0.0
}, {
"name" : "integration.channel.errorChannel.sendRate.count",
"value" : 0.0
}, {
"name" : "integration.channel.applicationMetrics.errorRate.mean",
"value" : 0.0
}, {
"name" : "integration.channel.applicationMetrics.errorRate.max",
"value" : 0.0
}, {
"name" : "integration.channel.applicationMetrics.errorRate.min",
"value" : 0.0
}, {
"name" : "integration.channel.applicationMetrics.errorRate.stdev",
"value" : 0.0
}, {
"name" : "integration.channel.applicationMetrics.errorRate.count",
"value" : 0.0
}, {
"name" : "integration.channel.applicationMetrics.sendCount",
"value" : 73.0
}, {
"name" : "integration.channel.applicationMetrics.sendRate.mean",
"value" : 0.2
}, {
"name" : "integration.channel.applicationMetrics.sendRate.max",
"value" : 11.05
}, {
"name" : "integration.channel.applicationMetrics.sendRate.min",
"value" : 5.0
}, {
"name" : "integration.channel.applicationMetrics.sendRate.stdev",
"value" : 0.0
}, {
"name" : "integration.channel.applicationMetrics.sendRate.count",
"value" : 73.0
}, {
"name" : "integration.channel.nullChannel.errorRate.mean",
"value" : 0.0
}, {
"name" : "integration.channel.nullChannel.errorRate.max",
"value" : 0.0
}, {
"name" : "integration.channel.nullChannel.errorRate.min",
"value" : 0.0
}, {
"name" : "integration.channel.nullChannel.errorRate.stdev",
"value" : 0.0
}, {
"name" : "integration.channel.nullChannel.errorRate.count",
"value" : 0.0
}, {
"name" : "integration.channel.nullChannel.sendCount",
"value" : 0.0
}, {
"name" : "integration.channel.nullChannel.sendRate.mean",
"value" : 0.0
}, {
"name" : "integration.channel.nullChannel.sendRate.max",
"value" : 0.0
}, {
"name" : "integration.channel.nullChannel.sendRate.min",
"value" : 0.0
}, {
"name" : "integration.channel.nullChannel.sendRate.stdev",
"value" : 0.0
}, {
"name" : "integration.channel.nullChannel.sendRate.count",
"value" : 0.0
}, {
"name" : "integration.handler._org.springframework.integration.errorLogger.handler.duration.mean",
"value" : 0.0
}, {
"name" : "integration.handler._org.springframework.integration.errorLogger.handler.duration.max",
"value" : 0.0
}, {
"name" : "integration.handler._org.springframework.integration.errorLogger.handler.duration.min",
"value" : 0.0
}, {
"name" : "integration.handler._org.springframework.integration.errorLogger.handler.duration.stdev",
"value" : 0.0
}, {
"name" : "integration.handler._org.springframework.integration.errorLogger.handler.duration.count",
"value" : 0.0
}, {
"name" : "integration.handler._org.springframework.integration.errorLogger.handler.activeCount",
"value" : 0.0
}, {
"name" : "integration.source.org.springframework.cloud.stream.app.time.source.TimeSourceConfiguration.publishTime.inboundChannelAdapter.source.messageCount",
"value" : 369.0
}, {
"name" : "integration.handlerCount",
"value" : 1.0
}, {
"name" : "integration.channelCount",
"value" : 4.0
}, {
"name" : "integration.sourceCount",
"value" : 1.0
}, {
"name" : "integration.channel.output.send.mean",
"value" : 1.0
}, {
"name" : "integration.channel.errorChannel.send.mean",
"value" : 0.0
}, {
"name" : "integration.channel.applicationMetrics.send.mean",
"value" : 0.2
}, {
"name" : "integration.channel.nullChannel.send.mean",
"value" : 0.0
} ]
}, {
"guid" : "61434",
"index" : 1,
"properties" : {
"spring.cloud.dataflow.stream.app.label" : "time120RS",
"spring.application.index" : "1",
"spring.application.name" : "time-source",
"spring.cloud.dataflow.stream.name" : "foostream",
"spring.cloud.application.guid" : "61434",
"spring.cloud.dataflow.stream.app.type" : "source",
"spring.cloud.application.group" : "foostream"
},
"metrics" : [ {
"name" : "integration.channel.output.errorRate.mean",
"value" : 0.0
}, {
"name" : "integration.channel.output.errorRate.max",
"value" : 0.0
}, {
"name" : "integration.channel.output.errorRate.min",
"value" : 0.0
}, {
"name" : "integration.channel.output.errorRate.stdev",
"value" : 0.0
}, {
"name" : "integration.channel.output.errorRate.count",
"value" : 0.0
}, {
"name" : "integration.channel.output.sendCount",
"value" : 375.0
}, {
"name" : "integration.channel.output.sendRate.mean",
"value" : 1.0
}, {
"name" : "integration.channel.output.sendRate.max",
"value" : 1.02
}, {
"name" : "integration.channel.output.sendRate.min",
"value" : 1.0
}, {
"name" : "integration.channel.output.sendRate.stdev",
"value" : 0.0
}, {
"name" : "integration.channel.output.sendRate.count",
"value" : 375.0
}, {
"name" : "integration.channel.errorChannel.errorRate.mean",
"value" : 0.0
}, {
"name" : "integration.channel.errorChannel.errorRate.max",
"value" : 0.0
}, {
"name" : "integration.channel.errorChannel.errorRate.min",
"value" : 0.0
}, {
"name" : "integration.channel.errorChannel.errorRate.stdev",
"value" : 0.0
}, {
"name" : "integration.channel.errorChannel.errorRate.count",
"value" : 0.0
}, {
"name" : "integration.channel.errorChannel.sendCount",
"value" : 0.0
}, {
"name" : "integration.channel.errorChannel.sendRate.mean",
"value" : 0.0
}, {
"name" : "integration.channel.errorChannel.sendRate.max",
"value" : 0.0
}, {
"name" : "integration.channel.errorChannel.sendRate.min",
"value" : 0.0
}, {
"name" : "integration.channel.errorChannel.sendRate.stdev",
"value" : 0.0
}, {
"name" : "integration.channel.errorChannel.sendRate.count",
"value" : 0.0
}, {
"name" : "integration.channel.applicationMetrics.errorRate.mean",
"value" : 0.0
}, {
"name" : "integration.channel.applicationMetrics.errorRate.max",
"value" : 0.0
}, {
"name" : "integration.channel.applicationMetrics.errorRate.min",
"value" : 0.0
}, {
"name" : "integration.channel.applicationMetrics.errorRate.stdev",
"value" : 0.0
}, {
"name" : "integration.channel.applicationMetrics.errorRate.count",
"value" : 0.0
}, {
"name" : "integration.channel.applicationMetrics.sendCount",
"value" : 74.0
}, {
"name" : "integration.channel.applicationMetrics.sendRate.mean",
"value" : 0.2
}, {
"name" : "integration.channel.applicationMetrics.sendRate.max",
"value" : 12.88
}, {
"name" : "integration.channel.applicationMetrics.sendRate.min",
"value" : 5.0
}, {
"name" : "integration.channel.applicationMetrics.sendRate.stdev",
"value" : 0.0
}, {
"name" : "integration.channel.applicationMetrics.sendRate.count",
"value" : 74.0
}, {
"name" : "integration.channel.nullChannel.errorRate.mean",
"value" : 0.0
}, {
"name" : "integration.channel.nullChannel.errorRate.max",
"value" : 0.0
}, {
"name" : "integration.channel.nullChannel.errorRate.min",
"value" : 0.0
}, {
"name" : "integration.channel.nullChannel.errorRate.stdev",
"value" : 0.0
}, {
"name" : "integration.channel.nullChannel.errorRate.count",
"value" : 0.0
}, {
"name" : "integration.channel.nullChannel.sendCount",
"value" : 0.0
}, {
"name" : "integration.channel.nullChannel.sendRate.mean",
"value" : 0.0
}, {
"name" : "integration.channel.nullChannel.sendRate.max",
"value" : 0.0
}, {
"name" : "integration.channel.nullChannel.sendRate.min",
"value" : 0.0
}, {
"name" : "integration.channel.nullChannel.sendRate.stdev",
"value" : 0.0
}, {
"name" : "integration.channel.nullChannel.sendRate.count",
"value" : 0.0
}, {
"name" : "integration.handler._org.springframework.integration.errorLogger.handler.duration.mean",
"value" : 0.0
}, {
"name" : "integration.handler._org.springframework.integration.errorLogger.handler.duration.max",
"value" : 0.0
}, {
"name" : "integration.handler._org.springframework.integration.errorLogger.handler.duration.min",
"value" : 0.0
}, {
"name" : "integration.handler._org.springframework.integration.errorLogger.handler.duration.stdev",
"value" : 0.0
}, {
"name" : "integration.handler._org.springframework.integration.errorLogger.handler.duration.count",
"value" : 0.0
}, {
"name" : "integration.handler._org.springframework.integration.errorLogger.handler.activeCount",
"value" : 0.0
}, {
"name" : "integration.source.org.springframework.cloud.stream.app.time.source.TimeSourceConfiguration.publishTime.inboundChannelAdapter.source.messageCount",
"value" : 375.0
}, {
"name" : "integration.handlerCount",
"value" : 1.0
}, {
"name" : "integration.channelCount",
"value" : 4.0
}, {
"name" : "integration.sourceCount",
"value" : 1.0
}, {
"name" : "integration.channel.output.send.mean",
"value" : 1.0
}, {
"name" : "integration.channel.errorChannel.send.mean",
"value" : 0.0
}, {
"name" : "integration.channel.applicationMetrics.send.mean",
"value" : 0.2
}, {
"name" : "integration.channel.nullChannel.send.mean",
"value" : 0.0
} ]
} ],
"aggregateMetrics" : [ {
"name" : "integration.channel.output.send.mean",
"value" : 2.0
}, {
"name" : "integration.channel.nullChannel.send.mean",
"value" : 0.0
}, {
"name" : "integration.channel.applicationMetrics.send.mean",
"value" : 0.4
}, {
"name" : "integration.channel.errorChannel.send.mean",
"value" : 0.0
} ]
} ]
} ]
Data Flow Template
53. Overview
The central entrypoint is the DataFlowTemplate
class in package org.springframework.cloud.dataflow.rest.client
.
This class implements the interface DataFlowOperations
and delegates to sub-templates
that provide the specific functionality for each feature-set:
Interface | Description |
---|---|
StreamOperations |
REST client for stream operations |
CounterOperations |
REST client for counter operations |
FieldValueCounterOperations |
REST client for field value counter operations |
AggregateCounterOperations |
REST client for aggregate counter operations |
TaskOperations |
REST client for task operations |
JobOperations |
REST client for job operations |
AppRegistryOperations |
REST client for app registry operations |
CompletionOperations |
REST client for completion operations |
RuntimeOperations |
REST Client for runtime operations |
When the DataFlowTemplate
is being initialized, the sub-templates will be discovered
via the REST relations, which are provided by HATEOAS.[1]
If a resource cannot be resolved, the respective sub-template will result in being NULL. A common cause is that Spring Cloud Data Flow offers for specific sets of features to be enabled/disabled when launching. For more information see Feature Toggles. |
54. Using the Data Flow Template
When using the Data Flow Template the only needed Data Flow dependency is the Spring Cloud Data Flow Rest Client:
<dependency>
<groupId>org.springframework.cloud</groupId>
<artifactId>spring-cloud-dataflow-rest-client</artifactId>
<version>1.2.0.RC2</version>
</dependency>
With that dependency you will get the DataFlowTemplate
class as well as all needed
dependencies to make calls to a Spring Cloud Data Flow server.
When instantiating the DataFlowTemplate
, you will also pass in a RestTemplate
.
Please be aware that the needed RestTemplate
requires some additional configuration
to be valid in the context of the DataFlowTemplate
. When declaring a RestTemplate
as a bean, the following configuration will suffice:
@Bean
public static RestTemplate restTemplate() {
RestTemplate restTemplate = new RestTemplate();
restTemplate.setErrorHandler(new VndErrorResponseErrorHandler(restTemplate.getMessageConverters()));
for(HttpMessageConverter<?> converter : restTemplate.getMessageConverters()) {
if (converter instanceof MappingJackson2HttpMessageConverter) {
final MappingJackson2HttpMessageConverter jacksonConverter =
(MappingJackson2HttpMessageConverter) converter;
jacksonConverter.getObjectMapper()
.registerModule(new Jackson2HalModule())
.addMixIn(JobExecution.class, JobExecutionJacksonMixIn.class)
.addMixIn(JobParameters.class, JobParametersJacksonMixIn.class)
.addMixIn(JobParameter.class, JobParameterJacksonMixIn.class)
.addMixIn(JobInstance.class, JobInstanceJacksonMixIn.class)
.addMixIn(ExitStatus.class, ExitStatusJacksonMixIn.class)
.addMixIn(StepExecution.class, StepExecutionJacksonMixIn.class)
.addMixIn(ExecutionContext.class, ExecutionContextJacksonMixIn.class)
.addMixIn(StepExecutionHistory.class, StepExecutionHistoryJacksonMixIn.class);
}
}
return restTemplate;
}
Now you can instantiate the DataFlowTemplate
with:
DataFlowTemplate dataFlowTemplate = new DataFlowTemplate(
new URI("http://localhost:9393/"), restTemplate); (1)
1 | The URI points to the ROOT of your Spring Cloud Data Flow Server. |
Depending on your requirements, you can now make calls to the server. For instance, if you like to get a list of currently available applications you can execute:
PagedResources<AppRegistrationResource> apps = dataFlowTemplate.appRegistryOperations().list();
System.out.println(String.format("Retrieved %s application(s)",
apps.getContent().size()));
for (AppRegistrationResource app : apps.getContent()) {
System.out.println(String.format("App Name: %s, App Type: %s, App URI: %s",
app.getName(),
app.getType(),
app.getUri()));
}
Appendices
Appendix A: Migrating from Spring XD to Spring Cloud Data Flow
A.1. Terminology Changes
Old | New |
---|---|
XD-Admin |
Server (implementations: local, cloud foundry, apache yarn, kubernetes, and apache mesos) |
XD-Container |
N/A |
Modules |
Applications |
Admin UI |
Dashboard |
Message Bus |
Binders |
Batch / Job |
Task |
A.2. Modules to Applications
If you have custom Spring XD modules, you’d have to refactor them to use Spring Cloud Stream and Spring Cloud Task annotations, with updated dependencies and built as normal Spring Boot "applications".
A.2.1. Custom Applications
-
Spring XD’s stream and batch modules are refactored into Spring Cloud Stream and Spring Cloud Task application-starters, respectively. These applications can be used as the reference while refactoring Spring XD modules
-
There are also some samples for Spring Cloud Stream and Spring Cloud Task applications for reference
-
If you’d like to create a brand new custom application, use the getting started guide for Spring Cloud Stream and Spring Cloud Task applications and as well as review the development guide
-
Alternatively, if you’d like to patch any of the out-of-the-box stream applications, you can follow the procedure here
A.2.2. Application Registration
-
Custom Stream/Task application requires being installed to a maven repository for Local, YARN, and CF implementations or as docker images, when deploying to Kubernetes and Mesos. Other than maven and docker resolution, you can also resolve application artifacts from
http
,file
, or ashdfs
coordinates -
Unlike Spring XD, you do not have to upload the application bits while registering custom applications anymore; instead, you’re expected to register the application coordinates that are hosted in the maven repository or by other means as discussed in the previous bullet
-
By default, none of the out-of-the-box applications are preloaded already. It is intentionally designed to provide the flexibility to register app(s), as you find appropriate for the given use-case requirement
-
Depending on the binder choice, you can manually add the appropriate binder dependency to build applications specific to that binder-type. Alternatively, you can follow the Spring Initialzr procedure to create an application with binder embedded in it
A.2.3. Application Properties
-
counter-sink:
-
The peripheral
redis
is not required in Spring Cloud Data Flow. If you intend to use thecounter-sink
, thenredis
becomes required, and you’re expected to have your own runningredis
cluster
-
-
field-value-counter-sink:
-
The peripheral
redis
is not required in Spring Cloud Data Flow. If you intend to use thefield-value-counter-sink
, thenredis
becomes required, and you’re expected to have your own runningredis
cluster
-
-
aggregate-counter-sink:
-
The peripheral
redis
is not required in Spring Cloud Data Flow. If you intend to use theaggregate-counter-sink
, thenredis
becomes required, and you’re expected to have your own runningredis
cluster
-
A.3. Message Bus to Binders
Terminology wise, in Spring Cloud Data Flow, the message bus implementation is commonly referred to as binders.
A.3.1. Message Bus
Similar to Spring XD, there’s an abstraction available to extend the binder interface. By default, we take the opinionated view of Apache Kafka and RabbitMQ as the production-ready binders and are available as GA releases.
A.3.2. Binders
Selecting a binder is as simple as providing the right binder dependency in the classpath. If you’re to choose Kafka as the binder, you’d register stream applications that are pre-built with Kafka binder in it. If you were to create a custom application with Kafka binder, you’d add the following dependency in the classpath.
<dependency>
<groupId>org.springframework.cloud</groupId>
<artifactId>spring-cloud-stream-binder-kafka</artifactId>
<version>1.0.2.RELEASE</version>
</dependency>
-
Spring Cloud Stream supports Apache Kafka, RabbitMQ and experimental Google PubSub and Solace JMS. All binder implementations are maintained and managed in their individual repositories
-
Every Stream/Task application can be built with a binder implementation of your choice. All the out-of-the-box applications are pre-built for both Kafka and Rabbit and they’re readily available for use as maven artifacts [Spring Cloud Stream / Spring Cloud Task or docker images [Spring Cloud Stream / Spring Cloud Task Changing the binder requires selecting the right binder dependency. Alternatively, you can download the pre-built application from this version of Spring Initializr with the desired “binder-starter” dependency
A.3.3. Named Channels
Fundamentally, all the messaging channels are backed by pub/sub semantics. Unlike Spring XD, the
messaging channels are backed only by topics
or topic-exchange
and there’s no representation of
queues
in the new architecture.
-
${xd.module.index}
is not supported anymore; instead, you can directly interact with named destinations -
stream.index
changes to:<stream-name>.<label/app-name>
-
for instance:
ticktock.0
changes to:ticktock.time
-
-
“topic/queue” prefixes are not required to interact with named-channels
-
for instance:
topic:foo
changes to:foo
-
for instance:
stream create stream1 --definition ":foo > log"
-
A.3.4. Directed Graphs
If you’re building non-linear streams, you could take advantage of named destinations to build directed graphs.
for instance, in Spring XD:
stream create f --definition "queue:foo > transform --expression=payload+'-foo' | log" --deploy
stream create b --definition "queue:bar > transform --expression=payload+'-bar' | log" --deploy
stream create r --definition "http | router --expression=payload.contains('a')?'queue:foo':'queue:bar'" --deploy
for instance, in Spring Cloud Data Flow:
stream create f --definition ":foo > transform --expression=payload+'-foo' | log" --deploy
stream create b --definition ":bar > transform --expression=payload+'-bar' | log" --deploy
stream create r --definition "http | router --expression=payload.contains('a')?'foo':'bar'" --deploy
A.4. Batch to Tasks
A Task by definition, is any application that does not run forever, including Spring Batch jobs, and they end/stop at some point. Task applications can be majorly used for on-demand use-cases such as database migration, machine learning, scheduled operations etc. Using Spring Cloud Task, users can build Spring Batch jobs as microservice applications.
-
Spring Batch jobs from Spring XD are being refactored to Spring Boot applications a.k.a Spring Cloud Task applications
-
Unlike Spring XD, these “Tasks” don’t require explicit deployment; instead, a task is ready to be launched directly once the definition is declared
A.5. Shell/DSL Commands
Old Command | New Command |
---|---|
module upload |
app register / app import |
module list |
app list |
module info |
app info |
admin config server |
dataflow config server |
job create |
task create |
job launch |
task launch |
job list |
task list |
job status |
task status |
job display |
task display |
job destroy |
task destroy |
job execution list |
task execution list |
runtime modules |
runtime apps |
A.6. REST-API
Old API | New API |
---|---|
/modules |
/apps |
/runtime/modules |
/runtime/apps |
/runtime/modules/{moduleId} |
/runtime/apps/{appId} |
/jobs/definitions |
/task/definitions |
/jobs/deployments |
/task/deployments |
A.7. UI / Flo
The Admin-UI is now renamed as Dashboard. The URI for accessing the Dashboard is changed from localhost:9393/admin-ui to localhost:9393/dashboard
-
(New) Apps: Lists all the registered applications that are available for use. This view includes informational details such as the URI and the properties supported by each application. You can also register/unregister applications from this view
-
Runtime: Container changes to Runtime. The notion of
xd-container
is gone, replaced by out-of-the-box applications running as autonomous Spring Boot applications. The Runtime tab displays the applications running in the runtime platforms (implementations: cloud foundry, apache yarn, apache mesos, or kubernetes). You can click on each application to review relevant details about the application such as where it is running with, and what resources etc. -
Spring Flo is now an OSS product. Flo for Spring Cloud Data Flow’s “Create Stream”, the designer-tab comes pre-built in the Dashboard
-
(New) Tasks:
-
The sub-tab “Modules” is renamed to “Apps”
-
The sub-tab “Definitions” lists all the Task definitions, including Spring Batch jobs that are orchestrated as Tasks
-
The sub-tab “Executions” lists all the Task execution details similar to Spring XD’s Job executions
-
A.8. Architecture Components
Spring Cloud Data Flow comes with a significantly simplified architecture. In fact, when compared with Spring XD, there are less peripherals that are necessary to operationalize Spring Cloud Data Flow.
A.8.1. ZooKeeper
ZooKeeper is not used in the new architecture.
A.8.2. RDBMS
Spring Cloud Data Flow uses an RDBMS instead of Redis for stream/task definitions, application registration, and for job repositories.The default configuration uses an embedded H2 instance, but Oracle, DB2, SqlServer, MySQL/MariaDB, PostgreSQL, H2, and HSQLDB databases are supported. To use Oracle, DB2 and SqlServer you will need to create your own Data Flow Server using Spring Initializr and add the appropriate JDBC driver dependency.
A.8.3. Redis
Running a Redis cluster is only required for analytics functionality. Specifically, when the counter-sink
,
field-value-counter-sink
, or aggregate-counter-sink
applications are used, it is expected to also
have a running instance of Redis cluster.
A.8.4. Cluster Topology
Spring XD’s xd-admin
and xd-container
server components are replaced by stream and task
applications themselves running as autonomous Spring Boot applications. The applications run natively
on various platforms including Cloud Foundry, Apache YARN, Apache Mesos, or Kubernetes. You can develop,
test, deploy, scale +/-, and interact with (Spring Boot) applications individually, and they can
evolve in isolation.
A.9. Central Configuration
To support centralized and consistent management of an application’s configuration properties, Spring Cloud Config client libraries have been included into the Spring Cloud Data Flow server as well as the Spring Cloud Stream applications provided by the Spring Cloud Stream App Starters. You can also pass common application properties to all streams when the Data Flow Server starts.
A.10. Distribution
Spring Cloud Data Flow is a Spring Boot application. Depending on the platform of your choice, you
can download the respective release uber-jar and deploy/push it to the runtime platform
(cloud foundry, apache yarn, kubernetes, or apache mesos). For example, if you’re running Spring
Cloud Data Flow on Cloud Foundry, you’d download the Cloud Foundry server implementation and do a
cf push
as explained in the reference guide.
A.11. Hadoop Distribution Compatibility
The hdfs-sink
application builds upon Spring Hadoop 2.4.0 release, so this application is compatible
with following Hadoop distributions.
-
Cloudera - cdh5
-
Pivotal Hadoop - phd30
-
Hortonworks Hadoop - hdp24
-
Hortonworks Hadoop - hdp23
-
Vanilla Hadoop - hadoop26
-
Vanilla Hadoop - 2.7.x (default)
A.12. YARN Deployment
Spring Cloud Data Flow can be deployed and used with Apche YARN in two different ways.
-
Deploy the server directly in a YARN cluster
-
Leverage Apache Ambari plugin to provision Spring Cloud Data Flow as a service
A.13. Use Case Comparison
Let’s review some use-cases to compare and contrast the differences between Spring XD and Spring Cloud Data Flow.
A.13.1. Use Case #1
(It is assumed both XD and SCDF distributions are already downloaded)
Description: Simple ticktock
example using local/singlenode.
Spring XD | Spring Cloud Data Flow |
---|---|
Start
|
Start a binder of your choice Start
|
Start
|
Start
|
Create
|
Create
|
Review |
Review |
A.13.2. Use Case #2
(It is assumed both XD and SCDF distributions are already downloaded)
Description: Stream with custom module/application.
Spring XD | Spring Cloud Data Flow |
---|---|
Start
|
Start a binder of your choice Start
|
Start
|
Start
|
Register custom “processor” module to transform payload to a desired format
|
Register custom “processor” application to transform payload to a desired format
|
Create a stream with custom module
|
Create a stream with custom application
|
Review results in the |
Review results by tailing the |
A.13.3. Use Case #3
(It is assumed both XD and SCDF distributions are already downloaded)
Description: Simple batch-job.
Spring XD | Spring Cloud Data Flow |
---|---|
Start
|
Start
|
Start
|
Start
|
Register custom “batch-job” module
|
Register custom “batch-job” as task application
|
Create a job with custom batch-job module
|
Create a task with custom batch-job application
|
Deploy job
|
NA |
Launch job
|
Launch task
|
Review results in the |
Review results by tailing the |
Appendix B: Building
To build the source you will need to install JDK 1.8.
The build uses the Maven wrapper so you don’t have to install a specific version of Maven. To enable the tests for Redis you should run the server before bulding. See below for more information on how to run Redis.
The main build command is
$ ./mvnw clean install
You can also add '-DskipTests' if you like, to avoid running the tests.
You can also install Maven (>=3.3.3) yourself and run the mvn command
in place of ./mvnw in the examples below. If you do that you also
might need to add -P spring if your local Maven settings do not
contain repository declarations for spring pre-release artifacts.
|
Be aware that you might need to increase the amount of memory
available to Maven by setting a MAVEN_OPTS environment variable with
a value like -Xmx512m -XX:MaxPermSize=128m . We try to cover this in
the .mvn configuration, so if you find you have to do it to make a
build succeed, please raise a ticket to get the settings added to
source control.
|
The projects that require middleware generally include a
docker-compose.yml
, so consider using
Docker Compose to run the middeware servers
in Docker containers. See the README in the
scripts demo
repository for specific instructions about the common cases of mongo,
rabbit and redis.
B.1. Documentation
There is a "full" profile that will generate documentation. You can build just the documentation by executing
$ ./mvnw clean package -DskipTests -P full -pl spring-cloud-dataflow-docs -am
B.2. Working with the code
If you don’t have an IDE preference we would recommend that you use Spring Tools Suite or Eclipse when working with the code. We use the m2eclipe eclipse plugin for maven support. Other IDEs and tools should also work without issue.
B.2.1. Importing into eclipse with m2eclipse
We recommend the m2eclipe eclipse plugin when working with eclipse. If you don’t already have m2eclipse installed it is available from the "eclipse marketplace".
Unfortunately m2e does not yet support Maven 3.3, so once the projects
are imported into Eclipse you will also need to tell m2eclipse to use
the .settings.xml
file for the projects. If you do not do this you
may see many different errors related to the POMs in the
projects. Open your Eclipse preferences, expand the Maven
preferences, and select User Settings. In the User Settings field
click Browse and navigate to the Spring Cloud project you imported
selecting the .settings.xml
file in that project. Click Apply and
then OK to save the preference changes.
Alternatively you can copy the repository settings from .settings.xml into your own ~/.m2/settings.xml .
|
B.2.2. Importing into eclipse without m2eclipse
If you prefer not to use m2eclipse you can generate eclipse project metadata using the following command:
$ ./mvnw eclipse:eclipse
The generated eclipse projects can be imported by selecting import existing projects
from the file
menu.
Appendix C: Contributing
Spring Cloud is released under the non-restrictive Apache 2.0 license, and follows a very standard Github development process, using Github tracker for issues and merging pull requests into master. If you want to contribute even something trivial please do not hesitate, but follow the guidelines below.
C.1. Sign the Contributor License Agreement
Before we accept a non-trivial patch or pull request we will need you to sign the contributor’s agreement. Signing the contributor’s agreement does not grant anyone commit rights to the main repository, but it does mean that we can accept your contributions, and you will get an author credit if we do. Active contributors might be asked to join the core team, and given the ability to merge pull requests.
C.2. Code Conventions and Housekeeping
None of these is essential for a pull request, but they will all help. They can also be added after the original pull request but before a merge.
-
Use the Spring Framework code format conventions. If you use Eclipse you can import formatter settings using the
eclipse-code-formatter.xml
file from the Spring Cloud Build project. If using IntelliJ, you can use the Eclipse Code Formatter Plugin to import the same file. -
Make sure all new
.java
files to have a simple Javadoc class comment with at least an@author
tag identifying you, and preferably at least a paragraph on what the class is for. -
Add the ASF license header comment to all new
.java
files (copy from existing files in the project) -
Add yourself as an
@author
to the .java files that you modify substantially (more than cosmetic changes). -
Add some Javadocs and, if you change the namespace, some XSD doc elements.
-
A few unit tests would help a lot as well — someone has to do it.
-
If no-one else is using your branch, please rebase it against the current master (or other target branch in the main project).
-
When writing a commit message please follow these conventions, if you are fixing an existing issue please add
Fixes gh-XXXX
at the end of the commit message (where XXXX is the issue number).